Data Storage and Analytics Trends and Predictions 2025 From Industry InsidersData Storage and Analytics Trends and Predictions 2025 From Industry Insiders
IT leaders and industry insiders share their data storage, management, and analytics trends and predictions for 2025.
January 21, 2025
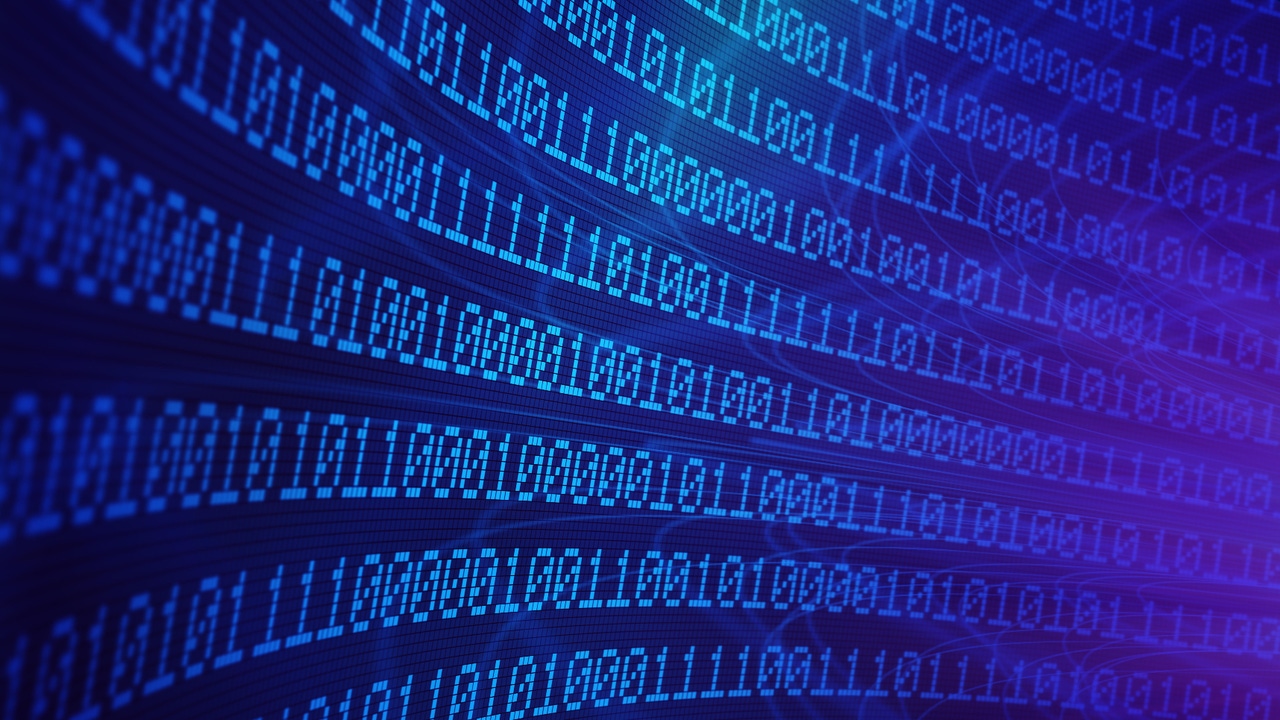
Data is vital to organizations because it serves as the foundation for decision-making, innovation, and competitive advantage in an increasingly digital and interconnected world. And its value continues to grow, driven by AI's transformative role in enabling organizations to leverage data more effectively.
What's in store for data storage and analytics in 2025? IT leaders and industry insiders are claiming AI will transform storage and lead to higher-quality data, and that we'll see a real-time analytics revolution.
Read on to see what else they are expecting from all things data in 2025.
But first, explore our 2025 tech predictions, including "anti-predictions" that challenge widely anticipated IT trends with fresh insights from our experts:
What the Tech Industry Expects From Data Storage, Management and Analytics in 2025
AI Will Transform Storage
In 2025, AI will continue to proliferate across all industries, driving new opportunities and challenges. The integration of AI into storage systems will be particularly transformative, with AI-powered solutions becoming increasingly common for optimizing performance, enhancing security and ensuring data reliability. This increase in AI workloads will lead to a surge in demand for high-performance storage solutions that can support these data-intensive applications, including large language models (LLMs), machine learning model training, and real-time data analytics. This will increase the requirements to data storage technologies in order to handle AI's specific needs for speed, scalability and efficiency. — Boyan Ivanov, CEO, StorPool Storage
Storage-Class Memory and AI-Driven Optimization Poised to Revolutionize Cloud Storage Efficiency
While hardware innovations such as DNA data storage and quantum storage are still confined to the research lab, storage-class memory, a technology partway between SSDs and DRAM memory, shows promise in combining the speed of RAM with the capacity of disk storage, albeit with a higher price point than SSDs. On the software side, cloud storage platforms will begin using machine learning algorithms to automatically optimize data placement, access patterns, and redundancy configurations. For example, training AI models to predict storage needs and automatically move data between hot, warm, and cold tiers to ensure the most efficient use of resources. — Pat Patterson, chief evangelist, Backblaze
Organizations Will Increasingly Employ the 'Goldilocks' Principle
As businesses look to control costs in anticipation of a potential economic downturn, finding the sweet spot between having enough capacity to store mission-critical data while minimizing storage spending will be seen as a higher priority in the coming year. While there are several avenues available to finding what's "just right" in terms of volume, performance, scalability, efficiency and manageability, the best one will be a storage system that accommodates present-day requirements with the ability to adapt in the future as workloads evolve. — Judy Kaldenberg, senior VP of sales and marketing, Nexsan
Data Localization Gains Momentum
2025 will bring a greater focus on data localization. Although cloud storage isn't going anywhere, stricter regulations and increased storage demand brought on by AI will give rise to awareness of and interest in the idea of storing data closer to where it was originally collected. We'll also see data being stored closer to the edge, especially for emerging technologies such as self-driving cars, smart homes, and AI models that need real-time, real-world data to operate quickly and avoid outages. — Sterling Wilson, Field CTO, Object First
Balancing AI Demands with Energy-Efficient Storage
As AI workloads grow, so do the energy demands and costs associated with them, pushing organizations to seek cost-saving, energy-efficient strategies. One key focus will be data storage, as vast datasets are crucial for AI but costly to maintain. Organizations will increasingly turn to scalable, low-power storage solutions, leveraging cold storage for less frequently accessed data to cut energy consumption. However, this "cold" data won't stay idle; it will be proactively recovered for reuse, re-monetization, and model recalibration as business needs evolve. By balancing high-performance access with efficient cold storage, companies can meet AI demands while reducing costs and environmental impact. — Tim Sherbak, Enterprise Products and Solutions manager, Quantum
Organizations Will Pursue Storage Systems That Can Do a Little Bit of Everything
Organizations looking to gain the versatility required to right-size their storage needs will seek out systems that combine different features and functions into one, such as hybrid arrays with flash for performance and spinning disk for deep capacity. Storage systems that can do a little bit of everything — efficiently unify different platforms and protocols (like block and file) to support different use cases while offering a variety of data management options for protection, security and business continuity — will be the ones most attractive to those needing to balance often-times divergent financial and operational requirements. — Judy Kaldenberg, senior VP of sales and marketing, Nexsan
Future-Proofing Against Growing Data Needs
There's an adage currently making the rounds that the best time to plant a tree is 20 years ago. The second-best time is now. While 2025 will see organizations right-sizing their storage infrastructures to match today's business environment, they will also need to plan to accommodate inevitable data growth by future proofing their storage infrastructure. Whatever storage protocols or hardware put into place must account for evolving workloads, data types, security and compliance policies, and more. In order to best prepare for storage needs both now and in the future, 2025 will see increased adoption of scalable storage systems that can grow as capacity needs increase to better offer flexibility to meet future requirements. — Judy Kaldenberg, senior VP of sales and marketing, Nexsan
Software-Defined Storage (SDS) Becoming a First-in-the-List Approach to Storage
As enterprises increasingly shift toward hybrid and multicloud environments, traditional hardware-based storage systems no longer meet the agility, scalability, manageability, and cost-efficiency demands of modern IT infrastructures. SDS, which runs on standard servers & networks and decouples data from the underlying hardware, offers unmatched flexibility in deploying, managing, and scaling storage resources across on-premises data centers and cloud environments. And for many hardware/data center refresh and data center consolidation projects, SDS is becoming a first-in-the-list option. We will continue seeing a transition toward fully automated, software-defined storage solutions with an emphasis on performance, security, manageability, APIs, and cost reduction across larger and more complex infrastructure projects. — Boyan Ivanov, CEO, StorPool Storage
AWS S3 Will Be Primary Storage Choice for Large-Scale Applications
In 2025, we'll see more solutions emerge explicitly built around AWS S3. In the past, developers tended to use S3 for cold storage. The reason is that it's relatively cheap to store data on S3, and expensive to access it. But over time, developers figured out how to use caching and other techniques to overcome the access penalty. Those techniques have become common knowledge, making S3 a popular choice as primary storage for large-scale applications. Developers can take advantage of S3's resilience and availability without having to worry about the cost. — Sunny Bains, software architect at PingCAP
Cyberstorage for Proactive Defense
In 2025, the growing threat landscape will make cyberstorage a critical feature of enterprise storage solutions. Cyberstorage integrates advanced security measures such as AI-driven threat detection, automated responses, and air-gapped immutable backups directly into storage systems. These capabilities will transform storage from a passive asset into an active defender against cyberattacks, providing organizations with real-time protection against data breaches, ransomware, and other malicious activities. — Aron Brand, CTO, CTERA
Unstructured Data Lakes
The rise of corporate AI agents in 2025 will push enterprises to develop unstructured data lakes that are AI-ready. These data lakes will be designed to handle the diverse, unstructured data needed for RAG (Retrieval-Augmented Generation) and large language models, ensuring data is collected, stored, and curated in a way that optimizes machine learning models. With robust security and compliance controls, these AI-ready data lakes will help organizations unlock new insights from data, allowing AI to drive decision-making and innovation across business functions. — Aron Brand, CTO, CTERA
Immutable Storage Becomes the Standard
With the increasing frequency of ransomware attacks and compliance requirements, the need for WORM (Write Once, Read Many) immutable storage will become ubiquitous by 2025. Storage systems will be designed to create air-gapped, tamper-proof repositories that cannot be deleted or modified by cybercriminals, even if they gain administrator credentials. This standardization of immutable storage will ensure that organizations can always recover clean copies of their data, offering stronger guarantees than what traditional backup repositories provide, and serving as a crucial safeguard against malicious encryption or data corruption. — Aron Brand, CTO, CTERA
The Rise of the Hybrid Lakehouse
The resurgence of on-prem data architectures will see lakehouses expanding into hybrid environments, merging cloud and on-premises data storage seamlessly. The hybrid lakehouse model offers scalability of cloud storage and secure control of on-premises, delivering flexibility and scalability within a unified, accessible framework. — Justin Borgman, co-founder and CEO, Starburst
SQL's Return to the Lake
SQL is experiencing a comeback in the data lake as table formats like Apache Iceberg simplify data access, enabling SQL engines to outpace Spark. SQL's renewed popularity democratizes data across organizations, fostering data-driven decision-making and expanding data literacy across teams. SQL's accessibility will make data insights widely available, supporting data empowerment. — Justin Borgman, co-founder and CEO, Starburst
Modern Data-Driven SaaS Applications Will Be Built on Lakes Rather Than Warehouses
New data applications will be built on the lake rather than traditional databases or data warehouses. The reason is simple: SaaS companies care deeply about gross margins in the products that they offer and data lakes offer significantly better TCO and no vendor lock-in. Building an application on an object storage lake allows companies to leverage open formats like Iceberg for storage and open engines like Trino for compute. The end result is an application stack that won't break the bank and is proven to handle Internet scale. — Justin Borgman, co-founder and CEO, Starburst
Global Data Explosion Threatens to Create a Storage Shortage Crisis
The world is creating data at unprecedented volumes. In 2028, as many as 400 zettabytes will be generated, with a compound annual growth rate (CAGR) of 24%. To make this relatable, according to research by the California Institute of Technology, just one zettabyte is equivalent to as much information as there are grains of sand on all the world's beaches. As AI matures and scales, the value of data will increase, leading us to store more data for longer. However, the storage install base is forecasted to have a 17% CAGR — therefore at a significantly slower pace than the growth in data generated. And it takes a whole year to build a hard drive. This disparity in growth rates will disrupt the global storage supply and demand equilibrium. As organizations become less experimental and more strategic in the use of AI, they will need to build long-term capacity plans to ensure storage supply, and fully monetize investments in AI infrastructure. — B.S. Teh, EVP and chief commercial officer, Seagate Technology
Storage Innovation Will Be Key to Tackling the Data Center Crunch and Protecting the Planet
As the data boom continues unabated, it will eventually reach the point where data centers will become overwhelmed. However, financial, regulatory, and environmental concerns will increasingly challenge the need for greater physical data center space and capacity. According to the UK's National Grid, for example, power demand from commercial data will increase six-fold just within the next 10 years. According to CBRE, AI advancements in particular are projected to significantly drive future data center demand, and high-performance computing will require rapid innovation in data center design and technology to manage rising power density needs. However, it's not just innovation in computing that can help address these issues. Higher areal density hard drives, which expand the amount of data stored on a given unit of storage media, can enable greater data capacity in data centers, avoiding the need to build new sites, driving significant TCO savings and reducing environmental impact. — B.S. Teh, EVP and chief commercial officer, Seagate Technology
2025 Will Be the Year of the AI Agent
Instead of merely producing text or images, this new breed of AI application will be empowered to act. That might mean researching topics on the web, manipulating an application on a PC desktop, or any other task that can be performed via API. We're still a long way from general artificial intelligence, so these early agents will be quite specialized. We'll see the emergence of what might be called "agentic architectures" — focused use cases where AI can deliver immediate value. Likely examples include data modeling, master data management, analytics and data enrichment, where tasks are highly structured and prototypes have already shown promise. We'll see the first case studies in 2025, and then rapid uptake throughout the enterprise as lagging adopters see competitors gaining an edge. — Bob van Luijt, CEO, Weaviate
Data Management Will Fuel Business and Decision Intelligence
In 2025, data management will be defined by its capacity to fuel business and decision intelligence. Achieving this goal relies on a foundation of insights across the application lifecycle built with security, governance, privacy, data residency and regulatory compliance all incorporated. For enterprises, the transition from passive data collection to creating standardized, actionable intelligence leveraging data fabric and data mesh warehousing technologies across interconnected systems will become increasingly pronounced. Legacy infrastructures unable to support cross-system integration and real-time analytics will face significant constraints, pushing IT leadership to prioritize ecosystem improvements. This shift will elevate data literacy from a technical skill to an organizational competency, with IT teams cultivating awareness across functions. This will also energize the use of AI to help mine the data for insights, create decision intelligence, and enable intelligent automation. — Sal Visca, Chief Technology Officer, Vertex
Businesses Reimagining Data as Dynamic Asset Will Thrive
Ultimately, organizations that thrive in 2025 are those that reimagine data not as a static resource, but as a dynamic asset driving innovation, decision-making, productivity, continuous compliance and controls and operational resilience. — Sal Visca, Chief Technology Officer, Vertex
GPU-Centric Data Orchestration Becomes Top Priority
As we head into 2025, one of the challenges in AI and machine learning (ML) architectures continues to be the efficient movement of data to and between GPUs, particularly remote GPUs. GPU access is becoming a critical architectural concern as companies scale their AI/ML workloads across distributed systems. Traditional data orchestration solutions, while valuable, are increasingly inadequate for the demands of GPU-accelerated computing. The bottleneck isn't just about managing data flow — it's specifically about optimizing data transport to GPUs, often to remote locations, to support high-performance computing (HPC) and advanced AI models. As a result, the industry will see a surge in innovation around GPU-centric data orchestration solutions. These new systems will minimize latency, maximize bandwidth, and ensure that data can seamlessly move across local and remote GPUs.
Companies already recognize this as a key issue and are pushing to rethink how they handle data pipelines in GPU-heavy architectures. Expect to see increasing investment in technologies that streamline data movement, prioritize hardware efficiency, and enable scalable AI models that can thrive in distributed and GPU-driven environments. — Molly Presley, SVP of Global Marketing, Hammerspace
Breaking Down Data Silos Will Become a Central Focus for AI and Data Architects
In 2025, breaking down data silos will emerge as a critical architectural concern for data engineers and AI architects. The ability to aggregate and unify disparate data sets across organizations will be essential for driving advanced analytics, AI, and machine learning initiatives. As the volume and diversity of data sources continue to grow, overcoming these silos will be crucial for enabling the holistic insights and decision-making that modern AI systems demand. The focus will shift from the infrastructure toward seamless data integration across various platforms, teams, and geographies. The goal will be to create an ecosystem where data is easily accessible, shareable, and actionable across all domains. Expect to see new tools and frameworks aimed at simplifying data integration and fostering greater collaboration across traditionally siloed environments. — Molly Presley, SVP of Global Marketing, Hammerspace
Enterprise HPC Must Align with Standardized Technologies for Unstructured Data Processing
By 2025, medium to large enterprises will face a pivotal challenge: integrating high-performance computing (HPC) for unstructured data processing while adhering to enterprise standards. As organizations increasingly rely on AI and data analytics to gain a competitive edge, the need to process vast amounts of unstructured data — like text, images, and video — will be unavoidable. However, enterprises have long struggled to adopt HPC at scale due to the complexities of reconciling specialized HPC technologies with enterprise security, compliance, and operational standards.
The solution lies in developing HPC technologies designed to work within enterprise-standard environments. In 2025, we expect to see the rise of enterprise-ready HPC solutions that seamlessly integrate with standard clients, operating systems, networks, and security frameworks. This convergence will enable organizations to finally leverage HPC for large-scale unstructured data processing without compromising enterprise security, compliance, or performance standards. — Molly Presley, SVP of Global Marketing, Hammerspace
2025: The Rise of Collaborative Global Namespaces
In 2025, the importance of how companies manage global namespaces will reshape data-handling strategies across the industry. Not all global namespaces will be created equal: Some will offer only read-only capabilities, while others will enable active read-write functionality. While having a single view of your data sounds efficient, its actual value lies in the ability to act on that data seamlessly. If teams can't collaborate in real time on a single dataset without creating multiple copies that require complex merging, it defeats the purpose of streamlined data management. The challenge of copy proliferation — where multiple users create their versions of the same dataset for individual read-write tasks — can introduce inefficiencies, silos, and data inconsistencies. As companies look to build more collaborative, efficient data environments, they will need to prioritize implementing global namespaces that not only allow for unified data views but also support active read-write capabilities. In this way, businesses can avoid data fragmentation while enabling seamless collaboration, making their data infrastructure elegant and functional for modern workloads. — Molly Presley, SVP of Global Marketing, Hammerspace
Synthetic Data Will Become a Primary Driver of AI-Powered Consumer Insights
With strong foundational models, synthetic data will solve the challenges of 1) gathering enough data to train AI models, and 2) avoiding having to use personal customer data. As more companies build synthetic data into their models, accuracy will continue to improve until wholly AI-powered consumer insights tools become widely trusted among both brands and consumers. — Mike Diolosa, CTO, Qloo
Strategic Use of Synthetic Data Will Be a Friend, Not a Foe
As more organizations discover the incredible potential of synthetic data —data that is statistically congruent with real-world data without resorting to manual collection or purchased third-party data —the perception of this technology will inevitably shift. Making the generation of synthetic data more accessible across a range of industries, from healthcare to manufacturing, will prove to be a significant strategic advantage. The future possibilities for leveraging this type of data are endless. — Susan Haller, Senior Director of Advanced Analytics, SAS
Flaming Data Dumpsters Fuel the AI Divide
2025 will reveal some organizations are thriving with generative AI — outpacing the competition, creating specialized customer experiences, launching innovative products faster. But other organizations are falling behind in the generative AI race. They're abandoning the wave of projects begun in 2023 because they overlooked a critical reality: AI needs good data. Poor data impedes AI performance, and organizations need to be brave enough to step back and fix their pervasive data issues. — Marinela Profi, global GenAI/AI strategy lead, SAS
The Demise of Traditional BI: API-First and GenAI Integrate Analytics into Every App
In 2025, traditional BI tools will become obsolete, as API-first architectures and GenAI seamlessly embed real-time analytics into every application. Data insights will flow directly into CRMs, productivity platforms, and customer tools, empowering employees at all levels to make data-driven decisions instantly—no technical expertise needed. Companies that embrace this shift will unlock unprecedented productivity and customer experiences, leaving static dashboards and siloed systems in the dust. — Ariel Katz, CEO, Sisense
Data Literacy Becomes a Mass Movement — Empowered by Composable Apps
In 2025, a mass data literacy movement will take hold, driven by composable apps that seamlessly integrate real-time analytics into everyday experiences. Consumers will actively engage with data on energy usage, shopping habits, and sustainability through intuitive, user-friendly platforms. Companies that simplify data reporting and empower users will thrive, while those relying on opaque, complex reports will face a consumer backlash demanding transparency. — Ariel Katz, CEO, Sisense
AI as an Upskilling Tool for Analytics Teams
In the coming year, AI will completely redefine analytics teams, enabling even non-technical members to master advanced analytics tasks. AI-driven tech approaches will dismantle traditional skill silos, empowering everyone from product managers to end-users to actively contribute to complex data projects. This shift will not only boost productivity but also foster a culture of seamless collaboration, accelerating innovation across organizations. — Yigal Edery, SVP Product & Strategy, Sisense
No-Code/Pro-Code Analytics Boundaries Will Be Shattered
In 2025, the boundary between no-code and pro-code analytics will dissolve entirely. By empowering product managers to generate 80% of analytics content and enabling developers to fine-tune it, AI-driven platforms will spark a revolution in analytics development, slashing development cycles and maximizing team efficiency. This game-changing approach will redefine how companies integrate analytics, making data-driven decision-making more collaborative and accessible than ever before. — Yigal Edery, SVP Product & Strategy, Sisense
Self-Serve Analytics Will Become the Norm for End Users
The growing demand for user empowerment will drive a shift towards self-serve analytics. Businesses will increasingly enable their customers to build and customize their own dashboards, making data more accessible and valuable at every level of the user experience. — Ronen Rubinfeld, SVP of engineering, Sisense
AI Will Lead to Higher-Quality Data
AI will renew the focus on data quality, for two reasons: First, high-quality data is required for training and fine-tuning models. Second, AI-powered analytics tools will offer a higher-resolution view of data, revealing previously undetected quality issues. — Ryan Janssen, CEO, Zenlytic
The Need for Real-Time Data Observability
Real-time data observability is going to become critical and organizations will need to ensure real-time observability of data in motion. Visibility into dynamic data workflows means teams can continuously optimize data pipelines in the moment. The result is a dramatic enhancement in system responsiveness and overall operational efficiency. Data pipelines don't just run smoothly, they also evolve in tandem with business requirements. — Somesh Saxena, CEO & founder, Pantomath
Data Observability Will Be a Key Trend in 2025
Data observability, when implemented correctly, will be the best tool for an organization to stay on the right track with data. Bringing observability for data and AI together is crucial for any business that wants to fully benefit from AI. Observability will help with security and governance and allow organizations to stay ahead of any issues whether data is at rest, in motion with ETL, used in applications, BI reports or ML/AI pipelines. Observability, however, will need to be active. For example, it won't be sufficient to understand that data freshness has fallen and just see that in a static display. Observability will need to trigger action, either via intelligent automation or via a human who's notified of what needs to be done. — Kunju Kashalikar, senior director of product management, Pentaho
Companies Will Adopt Advanced Data Management Strategies to Fuel AI Production
Companies will begin to deploy better data management strategies — where the data itself is classified, tiered and stored based on value and use — and will be able to fuel AI and GenAI production goals. Retiering and automating data storage based on value and usage will free up both infrastructure and data management costs, giving teams back more time to focus on higher value-added tasks while recouping budget that can help move AI and GenAI out of pilots and into cost-effective production at scale. Strong data classification will also bring significant benefits when handling PII, confidential information and bias when training models. — Kunju Kashalikar, senior director of product management, Pentaho
Data Governance Will Become Priority #1 in the AI Era
Organizations have largely settled on their data repository choices, such as Snowflake and DataBricks. The upcoming focus will shift from simply experimenting with AI utilization to fully operationalizing data governance. This means prioritizing access control, synchronization, translation, documentation, and resolution over mere experimentation. Companies are gearing up for the AI revolution by centralizing their data into these repositories. However, many still operate siloed systems with separate copies of relevant data interpreted through their unique models. This not only increases the threat surface but also heightens the need for transparency and control over data access, especially in an era fraught with data breaches and privacy fines. While 88% of data leaders acknowledge that data security will be a top priority in 2025 — surpassing even AI — many CEOs still prioritize growth. It's a balancing act, but as organizations adopt a "shift left" approach to data governance, they will instead prioritize strong data governance and security. This means extending robust capabilities from cloud data warehouses back to the data as it flows from source systems. — Jonathan Wright, senior sales engineer, MetaRouter
Personalization Will Be AI's No. 1 Role in Digital Wallet Ggrowth
Wallets are revolutionizing traditional application experiences by providing personalized profiles for transactions, preferences, and personal data. Wallet technology is being used to streamline payments, identity, credential management, and more. With the advent of AI, it's clear that the two technologies will be combined to provide hyper-personalized experiences, prevent fraud, and glean insights. Generative AI has been the story of the last 2 years. Personalization of AI will be the story of the next 2 years and data wallet technologies for managing that personal data will be the backbone of these initiatives. — Oz Olivo, VP of product management, Inrupt
Digital Wallets Will Go Beyond Credential and Card Management
Personal data wallets that honor privacy regulations will leverage Web 3.0 protocols to innovate in 2025 beyond simple credential management into predictive life navigation systems, synthesizing data from our wearables, smart home sensors, social connections, and environmental monitors to surface critical insights about our future well-being. These systems will detect subtle patterns — like how your gait has changed over 6 months, combined with vitamin D exposure, sleep quality, and bone density trends — to warn you about impending health issues months before traditional diagnostics would catch them. The same wallets will augment strides made in your professional network interactions, skill development patterns, and industry trends to identify career opportunities or risks that are invisible to the human eye alone — like how the technology of eyeglasses so clearly revolutionized human intelligence. Most importantly, these systems won't just present data — they'll understand the complex interplay between your physical, social, and professional life spheres to make holistic recommendations, like suggesting you move offices to reduce exposure to air pollution that's affecting your cognitive performance, or highlighting how your child's recent sleep disruption correlates with their falling test scores and your household's changed evening routine. — Davi Ottenheimer, VP of trust and digital ethics, Inrupt
AI Powers Next-Gen QR Code Analytics
AI integration in QR code platforms will focus heavily on data analytics and insights generation rather than code creation. Platforms will deploy AI as a co-pilot to analyze the vast amounts of behavioral data collected through QR code interactions, helping businesses understand scanning patterns, frequency and customer journey flows across multiple locations. This capability will enable companies to process and derive actionable insights from QR code data at scale, eliminating the need for manual analysis of weekly and monthly reports. — Ravi Pratap, co-founder and CTO, Uniqode
The Dawn of Real-Time RAG for Dynamic Insights in 2025
We'll see the emergence of real-time Retrieval-Augmented Generation (RAG) as organizations push beyond batch processing limitations. Today's RAG implementations primarily rely on static large language models (LLMs) paired with batch vector databases, which augment responses with preprocessed, stale data. While effective for many applications, this approach falls short for dynamic use cases that require real-time information updates, such as logistics optimization, personalized video game assistants, or financial risk monitoring. Real-time RAG will bridge this gap by integrating LLMs with real-time data streams and event-driven architectures, enabling models to access and leverage the freshest data during generation. This shift will unlock powerful, timely insights in scenarios where up-to-the-second context is critical, making 2025 a pivotal year for real-time augmented intelligence. — Kishore Gopalakrishna, co-founder and CEO, StarTree
From Streams to Insights: 2025 Marks the Real-Time Analytics Revolution
Real-time analytics will finally hit its stride as organizations complete the "last mile" of their data architecture. Over the past few years, businesses have focused heavily on building out event streaming systems like Apache Kafka, ensuring that data flows smoothly in real-time. However, many are now realizing that traditional analytic endpoints, such as data warehouses and batch-based solutions, are unable to fully harness the potential of these streams. These legacy systems simply can't deliver the instant insights needed in today's fast-paced environment. In 2025, organizations will prioritize real-time analytics platforms that can process, analyze, and act on data instantly, closing the loop and unlocking the true value of their streaming architectures. This shift will enable innovative use cases such as hyper-personalized customer experiences, real-time external-facing data products, and adaptive risk management systems—far beyond the capabilities of traditional solutions. — Kishore Gopalakrishna, co-founder and CEO, StarTree
Knowledge Graphs Will Make Data Smarter, Connecting the Dots Between GenAI and Users
Knowledge graphs provide a semantic layer that describes enterprise data ecosystems in human terms and simultaneously create new logical connections between previously disconnected data sources. As genAI models think in more human terms, knowledge graphs allow both the models and business users to "understand" the data available and subsequently produce real insights about it. — Christian Buckner, SVP, analytics and IoT, Altair
Knowledge Graphs Will Revolutionize How We Interact with Data
As organizations seek to democratize their data for competitive advantage, knowledge graphs provide a simpler way for business users to access and leverage that data. Acting like a smart assistant, knowledge graphs organize scattered data into a format that both humans and AI can easily understand and utilize. This streamlines gen AI's ability to deliver valuable insights and empowers people to make more informed decisions similar to how a virtual assistant can help plan your road trip. — Christian Buckner, SVP, analytics and IoT, Altair
Agentic AI Will Transform Data Analytics
Today, many business leaders struggle with knowing what questions to ask their data or where to find the answers. AI Agents are changing that by automatically delivering insights and recommendations, without the need for anyone to ask. This level of automation will be crucial for helping organizations unlock deeper understanding and connections within their data and empowering them to make more strategic decisions for business advantage. it's important for businesses to establish guardrails to control AI-driven suggestions and maintain trust in the results. — Christian Buckner, SVP, analytics and IoT, Altair
Conversational AI as a Business Transformation Tool
By 2025, conversational AI will emerge as the predominant real-world application of GenAI, significantly driving growth in self-service analytics for banking, financial services and insurance industries. Organizations will increasingly implement this technology internally enabling employees to quickly interpret data across their enterprise for enhanced decision-making, more efficient transactions and full transformation of how businesses are leveraging their data resources for competitive advantage. — Dylan Tancill, global head of BFSI, Altair
The Regulatory Imperative for Self-Service Analytics Tools in Finance
The proliferation of self-service analytics and end user computing (EUC) tools, many of which were built in-house, will lead to the establishment of more robust governance and guardrails within organizations and the financial industry as a whole. As regulators heighten their focus on risk management, businesses, particularly banks will begin to identify the riskiest use cases associated with these tools. In response, they will implement structured oversight to define where EUC can take place and what tools can be used. This will lead to a thorough evaluation of current toolsets to ensure compliance and reduce potential risks. — Dylan Tancill, global head of BFSI, Altair
No-Code/Low-Code Tools Offer Lifeline for Resource-Strapped Manufacturers
The manufacturing space will continue to face significant challenges, including supply chain issues, labor shortages, and intense global competition. In this climate, improving efficiency through technology will be crucial. With tight budgets, manufacturers will be selective in their technology investments. Low-code and no-code AI tools will provide significant value by enabling engineers to leverage data analytics without needing extensive coding skills. This shift will allow manufacturers to innovate and enhance processes without the added cost of hiring data scientists, helping them remain agile and competitive despite resource constraints. — Scott Genzer, specialist data scientist, Altair
Relying on Human Labeling as Key Teaching Source
Training AI is like teaching a toddler. While toddlers can be smart and take in new information, they also get confused very easily. Whatever information is fed into the AI is synthesized and interpreted, and the AI responds based on those inputs. If that data includes misinformation, harmful content, or biases, the responses can include the same. Overall, this becomes a challenge of data labeling and establishing definitions of a base truth. That's one reason teams should rely on human labeling as key teaching sources vs. relying on general data sources (for example, social media). Looking forward, solutions in training AI will become more nuanced with distinctions made in labeling data as facts, conjecture, theory, hypothesis, etc. — Michael Armstrong, chief technology officer, Authenticx
How CDOs Will Redefine Data Strategies in 2025
The acceleration of AI has brought an opportunity for advanced analytics, enabling organizations to exploit the data that is available to them. This is especially significant given the growing global data landscape, adding complexity, and requiring organizations to evolve their approach to data fusion and analysis so they are equipped to meet their strategic goals. Utilizing available and accessible data can also point organizations to new insights, drive more efficient operations, create new market opportunities, and meet employee training needs. Yet, the potential of any strategy is predicated on having a sound data environment. In 2025, Chief Data Officers (CDOs) must bring new value to their roles by ensuring their organizations are capitalizing on their accessible data. While the CDO position has largely been about compliance and risk management, it must now evolve to accomplish its traditional data management responsibilities while also demonstrating to leadership the opportunities data analytics can hold if the right strategies are put in place. There's a significant contrast between a CDO's defensive approach to compliance and their capacity to enhance business and mission results. A CDO must work to close this gap in the new year. — Chris Jones, CTO and CDO, Nightwing
Organizations Shift Toward Unified Platforms to Protect and Manage Data
In 2025, there will be a lot of focus on data, especially with further adoption of cloud platforms and the use of artificial intelligence. The ability to discover data and making sure the right people and models are accessing it will be crucial as organizations deal with data being accessed intentionally and unintentionally even by their own resources. Data security used to be a combination of point products for discovery, classification, labelling and loss prevention, but now the market is converging into platforms that can provide more than one of these capabilities at a time. The trend toward platformization, having one platform to pull all your needs together, will accelerate but many organizations will still find themselves struggling to properly classify and protect their data from threat actors as well as unintended access from insiders with good intentions. — Justin Flynn, senior director, Stratascale
Overcoming Data Access Challenges Becomes Critical for AI Success
In 2025, organizations will face increasing pressure to solve data access challenges as AI workloads become more demanding and distributed. The explosion of data across multiple clouds, regions, and storage systems has created significant bottlenecks in data availability and movement, particularly for compute-intensive AI training. Organizations will need to efficiently manage data access across their distributed environments while minimizing data movement and duplication. We'll see an increased focus on technologies that can provide fast, concurrent access to data regardless of its location while maintaining data locality for performance. The ability to overcome these data access challenges will become a key differentiator for organizations scaling their AI initiatives. — Haoyuan Li, founder and CEO, Alluxio
Real-Time Failover for AI-Powered Security Analytics
As AI-driven security analytics tools become standard for detecting and responding to threats, organizations will prioritize high availability to ensure these applications operate without downtime. Failover clustering will play a crucial role in maintaining continuous, real-time threat detection and response, preventing gaps in security coverage that could leave the enterprise vulnerable. By leveraging failover clustering, enterprises will mitigate risks and enable uninterrupted operation of their critical security monitoring and analytics tools. — Cassius Rhue, vice president, customer experience, SIOS Technology
Cloud-Native Solutions to Shape the Future of Data Security
With data spread across diverse cloud-native architectures, adaptive, data-centric security is essential. Cloud-native solutions now provide dynamic protection across data lifecycles, securing data at rest, in motion, and in use. This will be critical in 2025 as stricter compliance standards and more data-centric attacks demand robust, consistent security for data everywhere. In 2025, cloud-native solutions will be crucial for staying resilient, adapting to new regulations, and navigating an ever-evolving threat landscape. — Moshe Weis, CISO, Aqua Security
Energy Availability Will Become Key Limiting Factor for Quantum, AI, and Data Analytics Growth
As the demand for quantum computing, AI and very large scale data analytics continues to rise, the industry will hit a significant barrier: energy scarcity. By 2030, data centers could consume up to 10% of global energy, and many key regions, such as Virginia and Texas, are already nearing capacity limits. Illinois is one of the few places with available power, but even that supply is being rapidly consumed. Future growth in these technologies will depend on securing vast amounts of clean energy, a shift in enterprise priorities towards energy efficiency, and sustainable power sources to stay competitive. — Chris Gladwin, CEO and founder, Ocient
Cloud-Only Analytics Fade as Enterprises Shift to Cost-Effective, Predictable Solutions
Enterprises are on the verge of a major shift in their approach to data analytics, as cloud-only solutions face increasing scrutiny due to opaque billing practices from providers, often leading to unexpected expenses that undermine financial planning. Currently, more than half of companies identify cloud spend as a top concern, yet lack the visibility needed to truly control or optimize these costs. Without clear insights into actual usage and application requirements, businesses are essentially "driving blind," much like driving without a gauge for fuel mileage. In the next 12-18 months, this lack of transparency is likely to drive a substantial movement toward hybrid and alternative models that promise greater predictability and control. Until cloud providers deliver the transparency needed for accurate spending oversight, cloud-only models will take a back seat as businesses seek sustainable, controlled solutions that allow them to manage and optimize their usage effectively. — Chris Gladwin, CEO and founder, Ocient
Organizations Must Refocus on Data
While generative AI is garnering all the attention, most organizations are missing the prerequisite to unlocking its value — the underlying data. The large language models (LLMS) that feed generative AI are wholly dependent on the quality of an organization's data. It's no surprise the enterprises are currently struggling to get their GenAI projects beyond pilot phases without a modern data strategy. In order to capture a return on their generative AI investments in 2025, expect organizations to focus heavily on technologies that can effectively gather and govern massive amounts of unstructured data. — Drew Firment, chief cloud strategist, Pluralsight
Retaining Extensive Data Sets Will Become Essential
Generative AI depends on a wide range of structured, unstructured, internal, and external data. Its potential relies on a strong data ecosystem that supports training, fine-tuning, and Retrieval-Augmented Generation (RAG). For industry-specific models, organizations must retain large volumes of data over time. As the world changes, relevant data becomes apparent only in hindsight, revealing inefficiencies and opportunities. By retaining historical data and integrating it with real-time insights, businesses can turn AI from an experimental tool into a strategic asset, driving tangible value across the organization. — Lenley Hensarling, technical advisor, Aerospike
Instant Data Gratification
Businesses will prioritize real-time analytics, delivering insights within minutes to keep pace with intensifying customer and market demand and competition. This shift will enable faster decision-making across departments, from marketing to customer service, giving organizations a competitive edge. Real-time data will become essential for companies aiming to act on insights immediately, transforming analytics from an ad hoc, retrospective tool to a proactive business driver. — Justin Borgman, co-founder and CEO, Starburst
Accelerating and Scaling AI with Data Products
Well-defined data products become a prerequisite for scaling AI workflows like RAG. We all know that your AI is only as good as the data you feed it, and the importance of quality and governance will become more important than ever. Furthermore, data products INCLUDE business context, which is so critical to your AI applications. — Justin Borgman, co-founder and CEO, Starburst
Automated Data Pipelines
2025 will usher in truly automated data pipelines that eliminate manual intervention in data workflows. This breakthrough will free technical teams to focus on innovation while ensuring consistent, high-quality data delivery at scale. Real-time processing capabilities will become standard, enabling responsive applications that were previously impractical. — Anil Inamdar, Head of Consulting Services, NetApp Instaclustr
Unstructured Data Governance Processes for AI Will Mature
Protecting corporate data from leakage and misuse and preventing unwanted, erroneous results of AI are top of mind for executives today. A lack of agreed-upon standards, guidelines and regulations in North America is making the task more difficult. IT leaders can get started by using data management technology to get visibility on all their unstructured data across storage. This visibility is the starting point to understanding this growing volume of data better so that it can be governed and managed properly for AI. Data classification is another key step in AI data governance, and it involves enriching file metadata with tags to identify sensitive data that cannot be used in AI programs. Metadata enrichment is also available for aiding researchers and data scientists who need to quickly curate data sets for their projects by searching on keywords that identify file contents. With automated processes for data classification, IT can create workflows to continually send protected data sets to secure locations and, separately, send AI-ready data sets to object storage where it can be ingested by AI tools. Automated data workflow orchestration tools will be important for efficiently managing these tasks across petabyte-scale data estates. AI-ready unstructured data management solutions will also deliver a means to monitor workflows in progress and audit outcomes for risk. — Krishna Subramanian, co-founder and COO, Komprise
Role of Storage Administrator Evolves to Embrace Security and AI Data Governance
Pressing demands on both the data security and AI fronts are changing the roles of storage IT professionals. The job of managing storage has evolved, with technologies now more automated and self-healing, cloud-based and easier to manage. At the same time, there is increasing overlap and interdependency between cybersecurity, data privacy, storage and AI. Storage pros will need to make data easily accessible and classified for AI, while working across functions to create data governance programs that combat ransomware and prevent against the misuse of corporate data in AI. Storage teams will need to know where sensitive data lurks and have tools to develop auditable data workflows that prevent sensitive data leakage. — Krishna Subramanian, co-founder and COO, Komprise
Centralized Data Will Be Key to Better Decision-Making and End of 'Over the Wall' Mentality
Centralized access to information will no longer be a luxury but a necessity for seamless data flow, enabling real-time decision-making and seamless cross-disciplinary collaboration. As product designs evolve from basic mechanical structures to complex, integrated systems that combine electronics and software, collaboration across engineering, design, and manufacturing teams will become critical. A unified view of real-time data will break down silos, enabling faster, more informed decisions and driving innovation in product development. Prioritizing centralized access to data will alleviate constraints derived from the outdated "over the wall" mentality, where teams worked in isolation and passed incomplete information between departments. Instead, we will see a unified source of truth for all product data. This shift will allow engineering and manufacturing teams to collaborate from the earliest design stages, streamlining communication and accelerating decision-making. Research has shown that nearly half of manufacturers still struggle with poor decision-making, often due to fragmented and siloed data. We need to dramatically lower that number in 2025. With integrated tools, real-time, centralized data access, and cross-disciplinary collaboration, companies can reduce time-to-market, enhance innovation, and avoid the costly mistakes caused by fragmented information — leading the way in the future of design and manufacturing. — Manish Kumar, CEO and R&D vice president, SOLIDWORKS
Autonomous AI Agents and Large Quantitative Models Make Their Mark
Companies will adopt a more AI-centric, agentic strategy for problem-solving, which means creating AI systems which can make decisions based on interactions with their environment. But behind these agents we will need more than just language models — this is where Large Quantitative Models (LQMs) come in. LQMs will harness vast amounts of quantitative data, combined with physics-aware architectures, to tackle a diverse range of use cases. Expect revolutions in areas like drug discovery, materials design, healthcare diagnostics, financial modeling, and industrial optimization. — Dr. Stefan Leichenauer, VP of engineering, SandboxAQ
Generative AI Unlocks Unstructured Data to Drive Smarter, Faster Business Decisions
A major focus will be on leveraging generative AI to unlock the value of unstructured data, which makes up the bulk of enterprise information. By transforming this data into actionable insights, AI will enable businesses to make informed decisions more efficiently, reducing reliance on human intervention for complex analysis. — Michael Curry, president of data modernization, Rocket Software
Data Quality Will Be Key to AI-Enabled Service Roles
Rapid AI tool innovation and adoption are transforming service-based roles, with the most immediate impact visible in customer support, IT support and marketing functions. As organizations move from experimentation to more established processes, these service roles will evolve and move to more solidified and usable processes in 2025. IT organizations will see wider implementation and standardization of AI-enabled services, and IT support roles will expand beyond help desk functions into AI tool deployment and optimization. Success in these evolved service roles will depend heavily on high-quality data. A focus on maintaining well-organized and clean data will be critical to ensuring AI tools can effectively assist with customer inquiries and service delivery. — Julie Irish, SVP and CIO, Couchbase
GenAI Will Transform Data Graveyards into AI Goldmines
Organizations are sitting on "data graveyards" — repositories of historical information that became too resource-intensive to maintain or analyze. This is largely because it can be expensive to tag data and keep track of it. Many companies defaulted to "store everything, analyze little" approaches due to the complexity and high costs related to data management. Yet valuable insights remain buried in emails, documents, customer interactions and operational data from years past. With GenAI tooling, there's an opportunity to efficiently process and analyze unstructured data at unprecedented scale. Organizations can uncover historical trends, customer behaviors and business patterns that were too complex to analyze before. Previously unusable unstructured data will become a valuable asset for training domain-specific AI models. — Haseeb Budhani, co-founder and CEO, Rafay Systems
Analytics and Operations Will Merge in 2025
The artificial divide between analytics and operations will dissolve in 2025 as enterprises recognize that real business value emerges from their fusion. This integration will manifest in real-time operational optimization and data products that directly enhance customer experience, such as sophisticated recommendation engines that adapt to changing business conditions. — Anil Inamdar, head of consulting services, NetApp Instaclustr
2025 Will Accelerate Supply Chain Innovation with ML and Synthetic Data
Going into 2025, machine learning is pretty exciting for the supply chain. For a while, we've been able to interpret visual data from camera feeds, sensors and IoT devices, but with the addition of synthetic data, we can create diverse, high-quality training sets and refine models long before they go live. Consider, for example, an autonomous forklift in a warehouse that needs to navigate around potential hazards. Synthetic data can be used to simulate a vehicle crashing into a storage rack or even a group of people. You then end up with a more robust model going into to production, which knows how to prevent damage and injury without ever having to experience these situations in real life. — James Brenan, global head of advisory, supply chain & manufacturing, Endava
Revenue Data Will Become the Most Valuable Enterprise Asset
In 2025, revenue data will become the ultimate enterprise asset. The ability to aggregate, analyze, and act on internal and external data streams will define market leaders. In an era where insight drives action, companies that fail to harness the potential of their data — whether through advanced analytics or AI — will be left behind. Harnessing revenue data to fuel go-to-market strategy and execution will be the decisive edge for the next generation of disruptors. — Andy Byrne, CEO, Clari
Data Protection Strategies Will Evolve to Secure Data in Use
By 2025, data protection strategies will shift from solely securing data at rest or in transit to securing data in use. Privacy preserving technologies like homomorphic encryption and confidential computing will see widespread adoption, driven by compliance requirements and the need for real-time collaboration without compromising sensitive data. Sectors like healthcare and education will embrace AI-based anomaly detection to safeguard their treasure troves of personal and organizational data, addressing attackers increasing focus on these industries. Incident response will move from annual table-top exercises to continuous testing through simulated attack platforms, enabling organizations to measure readiness in real time. — Adam Khan, VP, Global Security Operations, Barracuda
AI Shifts from Insights to Strategic Business Value
As we head into 2025, AI will evolve from merely providing insights to driving strategic business value through contextualization. As data and analytics become even more integrated over the next year, workflows will be able to connect data from organizations' real-world events, operations and people to understand the specific roles of employees and teams. This means moving beyond broad data outputs to creating actionable insights. Employees at all levels will be empowered through personalized information to make better decisions and deliver stronger results for customers. Businesses that harness contextual AI will see productivity skyrocket. The ability to customize approaches based on real-time data and insights will be the key to thriving in an increasingly competitive landscape. — John Licata, innovation officer, ServiceNow
Microscopic Lens on the Source of Data Labeling
In technical circles, there are constant discussions around how to get the right dataset — and in turn, how to label that dataset. The reality is that this labeling is outsourced on a global scale: In many cases, it's happening internationally, and often in developing countries, with questionable conditions and levels of pay. You may have task-based workers assessing hundreds of thousands of images and being paid for the number accurately sorted. While AI engineers may be highly in demand and paid well above the market rate, there are questions about this subeconomy. — Gordon Van Huizen, SVP of Strategy, Mendix
Digital Transformation in Healthcare Will Boost Data Quality
Over the coming year, digital transformation will take a stronger hold in healthcare, ultimately improving overall data quality and expanding the use cases for AI within the industry. Already, healthcare organizations are leveraging AI to streamline workflows, reduce administrative burdens on staff, and optimize manual tasks like scheduling. As organizations continue to adopt new digital tools and improve upon those already within their tech stack, however, they will be better equipped to manage, analyze, and clean valuable data on a much larger scale. — Dr. Hugh Cassidy, head of artificial intelligence and chief data scientist, LeanTaaS
The Ability to Navigate, Analyze, and Action Unstructured Data Will Define Your Business
Just like its name implies, unstructured data is often the hardest to make sense of and yet it's also some of the most valuable information within a company. Eighty percent of enterprise data is unstructured data, according to Gartner. 2025 will distinguish the haves from the have nots: the businesses that have the tools and tech to process unstructured data and make it AI-ready will come out on top in this new agentic era. These companies will be better positioned to not only generate stronger business insights — but their teams and agents will be better equipped to make decisions and take action — from analyzing customer sentiments to generating blog posts and creating competitive plans. — Sarah Walker, COO, Slack
We're Talking the Data Race Versus the Arms Race
In the last year, there has been a frenzy around AI, with investors and organizations throwing cash at the buzzy technology. But the real winners are those who saw past the "buzz" and focused on actionable takeaways and what will actually help their organization. We're finding now that the gold rush isn't the technology itself, it's the data that feeds AI and the value it presents. In 2025, organizations that take a more pragmatic approach to AI — and its underlying data infrastructure — will be best prepared to fuel new insights and power discovery. Those who are leading the data race are the ones who are not only leveraging every scrap of their collected data for differentiated AI outcomes, but those who have an infrastructure and process in place for effectively doing so — managing, organizing, indexing, and cataloging every piece of it. They'll produce more, faster, and better results than their competitors. In 2025, we'll start to see who leaps ahead in this new "data and algorithm arms race." — Skip Levens, product leader & AI strategist, Media & Entertainment, Quantum
Moving into Our Digitalization and Data Hygiene Era
As more organizations deploy GenAI in 2025, they'll embrace data hygiene and digitalization to increase ROI and better business outcomes. Data hygiene is foundational to the success of generative AI applications because it ensures that AI systems function optimally and provide useful, unbiased insights. It also helps organizations navigate the ethical, legal, and operational challenges associated with deploying AI. However, according to Appian's report there's been a 10% year-over-year increase in bottlenecks related to sourcing, cleaning, and labeling data. These challenges are directly impacting the ability of companies to successfully deploy AI projects. Companies will also recognize the importance of digitalization to the success of GenAI deployments because it allows companies to standardize data, making it more consistent and enabling employees and various stakeholders to work with the same datasets, facilitating collaboration and informed decision-making. These advancements, along with the adoption of AI governance platforms and strategies, will help businesses drive more value from their GenAI applications in 2025 and beyond. — Scott Francis, technology evangelist, PFU America
Increased Automation in Data Observability
Now that data observability has reached a level of market maturity, automation will be essential to maximizing its value. Observability tools will increasingly focus on reducing user time in the platform by automating workflows for deployment, issue identification, triage, and resolution. As best practices become standardized, speeding up these processes will be key to delivering real ROI and enabling teams to resolve data issues with minimal manual intervention. — Egor Gryaznov, chief technology officer, Bigeye
AI Will Drive Renewed Emphasis on Data Quality for Model Training, Enhanced Analytics
AI will renew the focus on data quality, for two reasons: First, high quality data is required for training and fine-tuning models. Second, AI-powered analytics tools will offer a higher-resolution view of data, revealing previously undetected quality issues. — Ryan Janssen, CEO, Zenlytic
Unstructured Data Management Solutions Broaden to Serve AI Data Governance and Monitoring Needs
The Komprise 2024 State of Unstructured Data Management report uncovered that IT leaders are prioritizing AI data governance and security as the top future capability for solutions. AI data governance covers protecting data from breaches or misuse, maintaining compliance with industry regulations, managing biases in data, and ensuring that AI does not lead to false, misleading or libelous results. Monitoring and alerting for capacity issues or anomalies, last year's top pick, remains high again along with analytics and reporting. IT and storage directors will look for unstructured data management solutions that offer automated capabilities to protect, segment and audit sensitive and internal data use in AI—a use case that is bound to expand as AI matures. — Krishna Subramanian, co-founder and COO, Komprise
Hybrid Cloud Persists, Mandating Deep Intelligence on Data and Costs
After years of ping-ponging between cloud-first strategies, then cloud repatriation and back again, the survey says: hybrid cloud is here to stay for the foreseeable future. IT leaders have realized that a mix of on-premises, edge and cloud computing is a sensible, risk-averse strategy to satisfy the needs of different workloads and departments. Storage and cloud vendors will adapt to this reality while IT will need to get intelligence on their data assets so they can move data into the optimal storage over its lifecycle. Optimizing a hybrid cloud storage environment will be a moving target dependent upon real-time analytics on data types, growth and access patterns and the flexibility to move data to secondary or cloud storage tiers as needed. Storage professionals can amp up their career by adopting an analytics mindset in everything they do. — Krishna Subramanian, co-founder and COO, Komprise
No Single Global Namespace Will Win
Unstructured data is trapped in many places. These silos make it difficult to manage and extract value from it. Many storage vendors are attempting to address the silo issue by saying if customers would only move to their storage vendor and away from other silos, they could have a unified solution with a single global namespace. This is a simplistic assumption that will not come to pass. Customers use a variety of storage vendors and storage architectures because their data has different demands throughout its lifecycle. Furthermore, the last decade has shown us that customers want to remain hybrid and leverage a mix of on-premises and cloud offerings. The answer to the silo problem is not to eliminate silos but rather, to get a single pane of glass across all silos and be able to move data from file to object and vice-versa while extending the primary namespace. With Flash prices rising and the introduction of new GPU-optimized storage plus the expansion of capacity storage offerings such as immutable object storage, IT organizations have more options than ever before. Storage-agnostic data management with transparency will be favored over proprietary single-vendor global namespace solutions. — Krishna Subramanian, co-founder and COO, Komprise
Data Tools Will Better Align with Diverse Business Needs
When businesses first began deploying data management tools, their main goal was often to centralize and bring order to the vast quantities of data that they owned but were struggling to manage. They wanted to be able to track their data from a central hub, which is why data lakes and data warehouses entered the picture. But now that many organizations have tamed that chaos, they're turning to data management tools for a different, more sophisticated purpose. They want to be able to give each unit within the business access to the data it needs, on the terms it needs. This requires a more complex and decentralized approach to data management — one powered by tools like data meshes and data marts. Central data repositories won't go away, but they'll increasingly be accompanied by data tools and platforms that better align data with diverse business needs. — Matheus Dellagnelo, co-founder and CEO, Indicium
Increased Focus on Data Transformation
Businesses that have established data infrastructures in place are increasingly expecting their infrastructures to do more than just store data and make it available for analysis and reporting. They also want to be able to transform data — which means restructuring, cleaning, validating or otherwise processing it in ways that improve its quality and increase its value. For this reason, expect to see data management tools offer more complex data transformation capabilities in 2025 and beyond. We're already seeing this from vendors like dbt, and this trend is likely to extend to others as well in the coming year. — Matheus Dellagnelo, co-founder and CEO, Indicium
A Practical Approach to Data Quality
"Data quality" has long been a buzzword. Most businesses with mature data management strategies in place understand the importance of ensuring that the data they use for analytics, or to power AI apps and services, must be high in quality. You don't need a Ph.D. in data science to understand the "garbage in, garbage out" concept. That said, traditional approaches to data quality have focused largely on implementing governance policies, not actually automating data quality policies. Companies have established rules about which data quality standards they expect engineers to uphold, but they've left it to the engineers to figure out how to apply those standards.
I'm starting to see this changing, however, as data management tools become more adept at enforcing data quality rules. This is due partly to the data transformation capabilities I mentioned above, since improving data quality is often one goal of data transformation. But it also reflects a growing awareness that automating data management, including data quality assurance processes, is critical for getting the most from data management tools. — Matheus Dellagnelo, co-founder and CEO, Indicium
Data Management Tool Consolidation
Traditionally, businesses have used different tools for each step of the data management process. They used one solution to warehouse data, another to prepare it, another to analyze it and so on. In other words, they took a "point" approach rather than a "platform" approach. But we're now seeing a greater focus on consolidation. Businesses are placing increasing value on data management platforms that provide all of the capabilities they need without requiring them to purchase and manage disparate tools. That said, it's important to keep in mind that flexibility and modularity will always be important components of a modern approach to data management. Organizations may appreciate the simplicity of consolidated data management platforms, but they'll still expect to be able to deploy the tools of their choosing when necessary, and they will resist being locked into a single vendor's platform or ecosystem. — Matheus Dellagnelo, co-founder and CEO, Indicium
A Multicloud-Friendly Approach to Data Management
Gone are the days when the typical business used just one cloud or other IT platform. Today, companies of significant size almost inevitably rely on multiple clouds, particularly because different units within the business might prefer different solutions or find more value in one cloud than another. For this reason, data management tools will increasingly need to friendly toward a multicloud approach. Solutions that only work with AWS or only with GCP, for example, will struggle to remain competitive in 2025 as businesses seek more flexibility. — Matheus Dellagnelo, co-founder and CEO, Indicium
Connect AI Models to Business Data
When businesses first began moving to take advantage of generative AI technology a couple of years ago, many focused on implementing "off the shelf" solutions that were pretrained on generic data, and that could accomplish generic tasks like populating word processor documents or presentation slides. That made sense at the time because connecting AI models to custom data is complicated, and many companies didn't have the data infrastructure, data quality or data management tools in place to train models extensively on their own data. So they settled for more basic solutions. Today, however, models trained on generic data are no longer enough to deliver a competitive edge. Businesses must also be able to connect models to their own business data so that the models can understand their unique business context and offer solutions tailored to it.
Some companies may go a step further by training their own models using custom data, too — although that practice is likely to become common only for larger businesses with particularly complex and specialized AI needs. Either way, expect 2025 to be defined in part by efforts to connect models to business data in ways that weren't important during earlier stages of AI adoption, when "off the shelf" tools sufficed. — Matheus Dellagnelo, co-founder and CEO, Indicium
Align Data with Business Needs
In most companies, data management is a task that falls to technical personnel. But it shouldn't be technical teams alone who are capable of working with data. Every business unit — from engineering, to accounting, to sales and marketing and beyond — should be able to leverage data to assist in decision-making and to help automate processes. To this end, businesses in 2025 should seek ways to align data with diverse business needs and use cases. Tools (such as no-code analytics solutions) exist to help with this process, but tools alone won't solve the challenge. Businesses also need to establish methodologies that allow them to transform and organize their data in ways that make it available to diverse stakeholders. — Matheus Dellagnelo, co-founder and CEO, Indicium
Simplify Data Access for Non-technical Stakeholders
Deriving the greatest value from data requires everyone in the business — including those without technical skills — to be capable of interacting with data. Here as well, the technology to "democratize" data access in this way exists. For example, generative AI and natural language processing tools make it possible for anyone to ask detailed questions about a data set and receive answers. You don't need to be able to write SQL queries to interact with data. Likewise, data mesh can help to simplify access to data for diverse stakeholders within a business. However, these approaches to data access only work if businesses have the data management processes in place to ensure that every stakeholder can find the data they need, and that the data is of sufficient quality to support their use cases. So, democratizing data is not just a matter of deploying new types of data analysis and reporting tooling; it's also about doubling-down on data management and quality. For these reasons, expect to see businesses increasingly investing in new approaches to data analysis and management in 2025 as they seek to place the power of data in the hands of all of their employees. — Matheus Dellagnelo, co-founder and CEO, Indicium
Real-Time Data Observability Becomes Essential
Real-time data observability is going to become critical and organizations will need to ensure real-time observability of data in motion. Visibility into dynamic data workflows means teams can continuously optimize data pipelines in the moment. The result is a dramatic enhancement in system responsiveness and overall operational efficiency. Data pipelines don't just run smoothly, they also evolve in tandem with business requirements. — Somesh Saxena, CEO & founder, Panomath
Digital Behavioral Data Will Be Hottest Trend in Large Datasets Behind GenAI
In 2025, enterprises will increasingly embrace their digital behavioral data to fuel revenue growth. In a landscape where every investment must show measurable impact, digital behavioral data uniquely fills gaps left by traditional analytics, offering richer insights into customer preferences, engagement patterns, and pain points. Collected from user interactions — like website views, newsletter sign-ups, shopping cart actions, and signals of frustration, such as "rage clicks" — this data will empower companies to make more precise, user-focused decisions. I expect we'll see continued innovation in how behavioral data is applied, fundamentally reshaping the ways organizations understand and engage with their audiences. — Scott Voigt, CEO and founder, Fullstory
Digital Behavioral Data Will Fuel Enterprise AI Algorithms
As enterprise AI advances, one thing is clear: the quality of data drives its success. Digital behavioral data offers deep insights into user preferences, patterns, and pain points, helping organizations predict behavior, personalize experiences, and detect threats. To unlock its potential, companies must ensure their data is accurate, unbiased, and AI-ready. With tools like ChatGPT thriving and data scarcity becoming a challenge, the focus must shift to harnessing meaningful behavioral insights. The future of AI depends on how effectively we use this data to deliver intelligent, transformative value for businesses and customers. — Scott Voigt, CEO and founder, Fullstory
Enterprises That Ready Their Data for AI Will Pull Ahead Competitively
In 2025, companies will focus on building an organized, high-quality data ecosystem to maximize AI's effectiveness and to pull ahead of their competition. This includes managing metadata through structured data catalogs, ensuring data accuracy with rigorous cleansing and validation, and establishing robust governance practices to safeguard data privacy and security. By implementing clear, ethical guidelines, organizations will create a trustworthy AI framework, empowering data scientists with easy access to reliable data for generating precise, impactful insights across business functions. Enterprises that do this will be hard to compete with. — Scott Voigt, CEO and founder, Fullstory
Making Data Readiness Central to AI Success
As we look toward 2025, data will no longer just support AI — it will shape and limit the scope of what AI can achieve. A robust data management strategy will be essential, especially as AI continues advancing into unstructured data. For years, companies have successfully leveraged structured data for insights, but unstructured data—such as documents, images, and embedded files — has remained largely untapped. The continued advancements in AI's ability to process the different types of unstructured data that reside within an enterprise are exciting, but they also require organizations to know what data they have and how and where it's being used. 2025 will mark the era of "data readiness" for AI. Companies that strategically curate and manage their data assets will see the most AI-driven value, while those lacking a clear data strategy may struggle to move beyond the basics. A data-ready strategy is the first step for any enterprise looking to maximize AI's full potential in the coming years. — Jim Liddle, chief innovation officer, Data Intelligence and AI, Nasuni
Streaming Data Platforms Will Become Essential for Real-Time Security and Observability
In 2025, streaming data platforms will become indispensable for managing the exponential growth of observability and security data. Organizations will increasingly adopt streaming data platforms to process vast volumes of logs, metrics, and events in real time, enabling faster threat detection, anomaly resolution, and system optimization to meet the demands of ever-evolving infrastructure and cyber threats. — Bipin Singh, senior director of product marketing, Redpanda
Streaming Data Platforms Will Power Agentic AI, RAG, and Sovereign AI Apps
In 2025, streaming data platforms will serve as the backbone for agentic AI, RAG AI and sovereign AI applications, providing the low-latency, high-throughput capabilities required to power autonomous decision-making systems and ensuring compliance with data sovereignty requirements. — Bipin Singh, senior director of product marketing, Redpanda
Expanded Emphasis on Data Sovereignty and Localization
Knowing exactly where your data is stored, a practice known as data sovereignty, has long been important for some businesses — especially as a means of complying with data privacy or security regulations that apply to certain regions or geopolitical jurisdictions. However, data sovereignty is assuming even greater importance. The main reason is that as more and more companies invest in AI, they're storing and processing vast quantities of data to train AI models. Controlling where all of that data is stored, as well as who within the company can access it, has become absolutely paramount. — Scott Wheeler, cloud practice lead, Asperitas
Systematic Data Ingestion for AI Will Be First Data Storage Mandate
AI mania is overwhelming, but so far, enterprise participation has been largely led by employees who are using GenAI tools to assist with daily tasks such as writing, research and basic analysis. AI model training has been primarily the responsibility of specialists, and storage IT has not been involved with AI. But this will change swiftly in the coming year. Business leaders know that if they get left behind in the AI Gold Rush, they may lose market share, customers and relevance. Corporate data will be used with AI for RAG and inferencing, which will constitute 90% of AI investment over time. Everyone touching data and infrastructure will need to step up to the plate as everyday employees start sending company data to AI. Storage IT will need to create systematic ways for users to search across corporate data stores, curate the right data, check for sensitive data and move data to AI with audit reporting. Storage managers will need to get clear on the requirements to support their business and IT counterparts. — Krishna Subramanian, co-founder and COO, Komprise
About the Author
You May Also Like