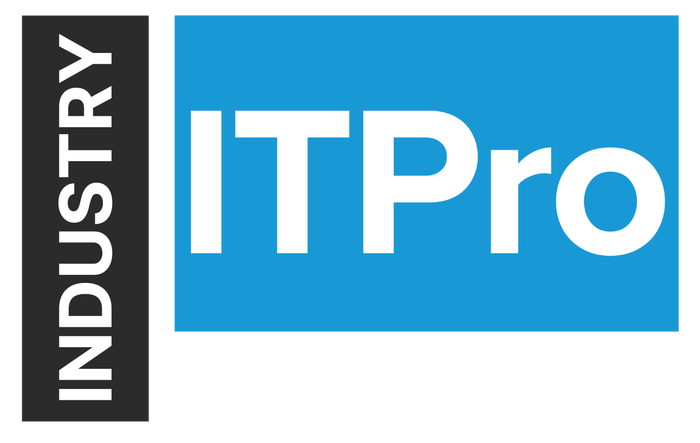
Insight and analysis on the information technology space from industry thought leaders.
How to Supercharge Data Analytics with AIHow to Supercharge Data Analytics with AI
Artificial intelligence is revolutionizing data analytics by reducing human error and enabling faster, more accurate insights, though it still requires careful oversight to avoid biases.
July 25, 2024
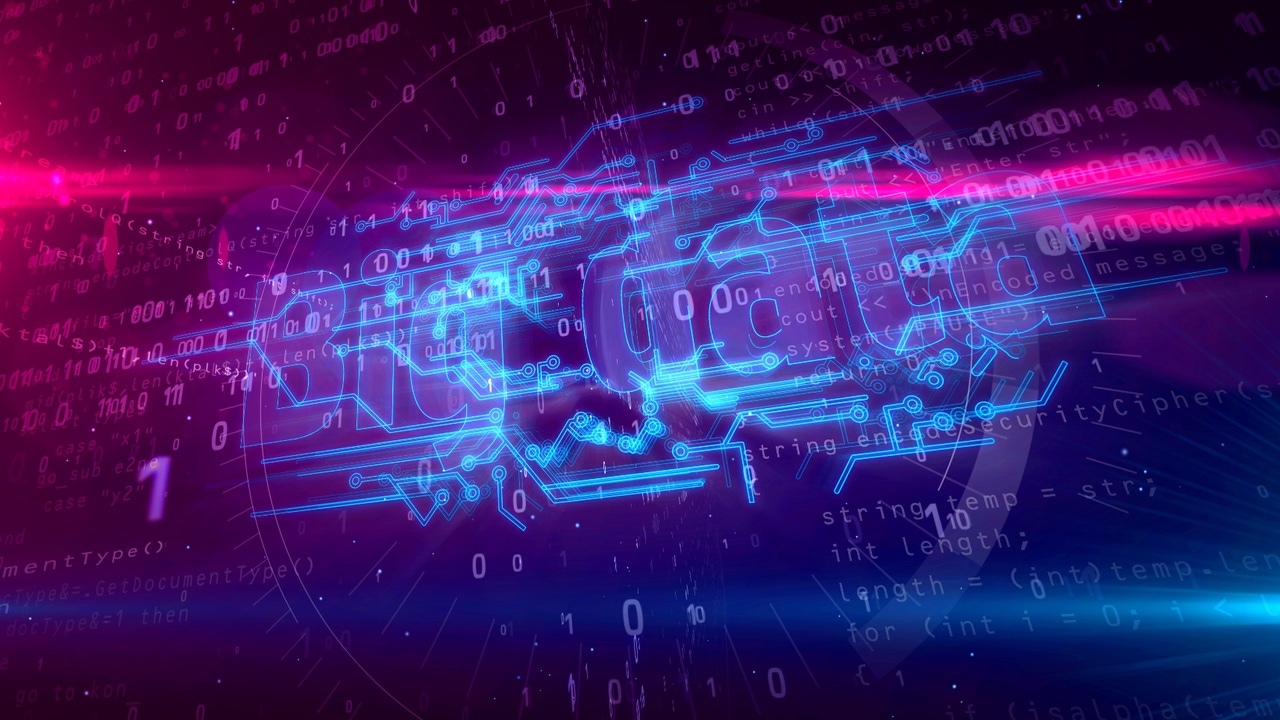
By Satyendra Kumar
Artificial intelligence and data analytics appear to be the perfect marriage. With AI, the need to scrutinize spreadsheets and reports from various organizational silos to tie together all the salient points and evidence of red flags is reduced. In addition to saving valuable time, human error is mitigated as well. AI helps to program the proper algorithms, access almost unlimited data points, take advantage of cloud storage, and produce the most relevant information needed to spot trends and issues to correct.
The future is here, and organizations that move early to adopt this approach while providing the enhanced employee training needed will stay one step ahead of the competition.
The Case for AI in Data Analytics
With the vast amounts of data that can be stored, especially as cloud services have become ubiquitous, traditional analytics tools may fall short. The inability to scale up as demand grows and a lack of accurate real-time analysis and insight derived from AI and machine learning (ML) via programmed algorithms are disadvantages in a competitive marketplace. That's why it is critical to prepare for the long haul. The constant drive to collect more data points in the era of big data means the demand for enhanced analysis will continue to ramp up, as will the need for more AI and ML to be employed.
Rather than using a data analyst to spend hours reviewing reports and spreadsheets, utilizing AI frees employees and allows them to focus more sharply on what an AI-generated report reveals regarding patterns or trends. That leads to better real-time decisions when a course correction is evident, which increases productivity.
The actionable insights derived from AI-fueled predictive analytics help drive future growth on a shorter timeline. Algorithms can be programmed to establish key performance indicators (KPIs), with alerts built in when a specific situation or an apparent anomaly must be addressed quickly. Snowflake's AI Data Cloud and Generative AI from AWS both tout scalable, customized services that help deliver better experiences for customers and employees.
The Downside of AI-Driven Analytics
Data analytics derived from AI are only as good as the algorithms programmed to monitor, flag, and collate that information. Any biased decision-making based on inherently biased training data or algorithmic biases can skew the analytics process. Skilled data architects and engineers are still in the mix, and data governance guidelines can help determine how algorithms are programmed to eliminate biases. There is also a concern, especially in the next generation of data analysts, that a loss of critical thinking may evolve and that an overreliance on AL and ML may mean a loss of human oversight.
Reports that once took hours to assemble manually can be organized in significantly less time by algorithms programmed to ask the right questions after accessing the ideal data points. Tempting as that might be, it is imperative for the human factor to always be in the mix when companies want to reduce IT overhead. Companies like IBM Cloud, Google Cloud, AWS, and Salesforce were identified as the top 10 AI-driven data analytics companies for 2024. Google Cloud was highlighted for its vertical integration model across data analytics, AI, ML, and cloud infrastructure, which allows for more seamless end-to-end solutions.
Establishing Standards for How to Use AI in Data Analysis
As with other nascent industries in the past, there are best practices, standards, and government regulations on data access and privacy. The Data & Trust Alliance, established in 2020 by some of the world's most prominent players (American Express, Meta, the NFL, UPS, and IBM, among others), has worked to create data provenance standards "to help organizations determine if data is suitable and trusted for use." The first data provenance standards from the Alliance were released late last year.
Another report states that a 2022 online survey revealed data professionals spent more than a third of their time preparing and cleansing data — more than they spend on reporting and visualization. This is where AI may be able to reduce that prepping and cleaning timeline.
Harnessing Big Data for Future Growth
AI can be incorporated into a modern cloud architecture and utilized with other reporting tools, such as PowerBI or Tableau that analyze data and create visual representations. As the algorithms "learn" more about the scanned data, AI and ML provide opportunities to remove some of the duplicated layers, which produces further cost savings and improved analytic capabilities. Taming big data in this fashion can mean faster response times, both in-house and for end-user queries.
The demand for data collection, management, and analysis is constantly increasing. This is fueled partly by businesses seeking a competitive edge by running their companies more efficiently (including automated decision-making via AI and ML) or spotting trends sooner, which makes the incorporation of still-emerging AI technologies very timely.
Those organizations come in various sectors, such as how professional sports are now employing AI for data analytics to study tendencies in preparation for an opponent or how to get an athlete back on track by reviewing the numbers to look for any slippage. Retail businesses can spot consumer trends faster and send personalized offers based on their digital history.
While there is a learning curve for data analysts, algorithm programmers, and other IT professionals, organizations that master the AI and data analytics dynamic to quickly identify "pain points" and stay current with the latest industry trends will have a leg up on the future.
About the Author:
Satyendra Kumar is a data architect with Cogent Data Solutions and has more than 15 years of leadership experience across multiple industries, including pharmaceuticals, finance, and manufacturing. He has helped organizations reduce IT costs and modernize IT infrastructure by collaborating with cross-functional stakeholders. Satyendra is an industry expert judge in Technology Awards by Globee and a member of the IEEE Society for Technology Professionals. Connect with Satyendra on LinkedIn.
About the Author
You May Also Like