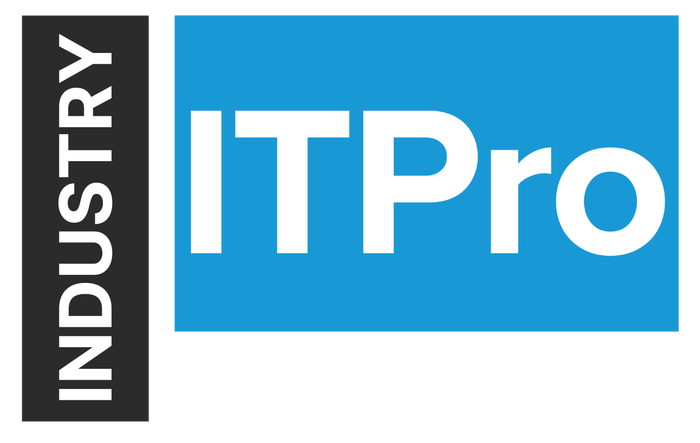
Insight and analysis on the information technology space from industry thought leaders.
How Silicon Diversity Optimizes Cost, Performance Across the AI LifecycleHow Silicon Diversity Optimizes Cost, Performance Across the AI Lifecycle
Strategically matching AI workloads to the right compute resources — through training, inference, and serverless inference — helps organizations control costs while maximizing performance.
February 7, 2025
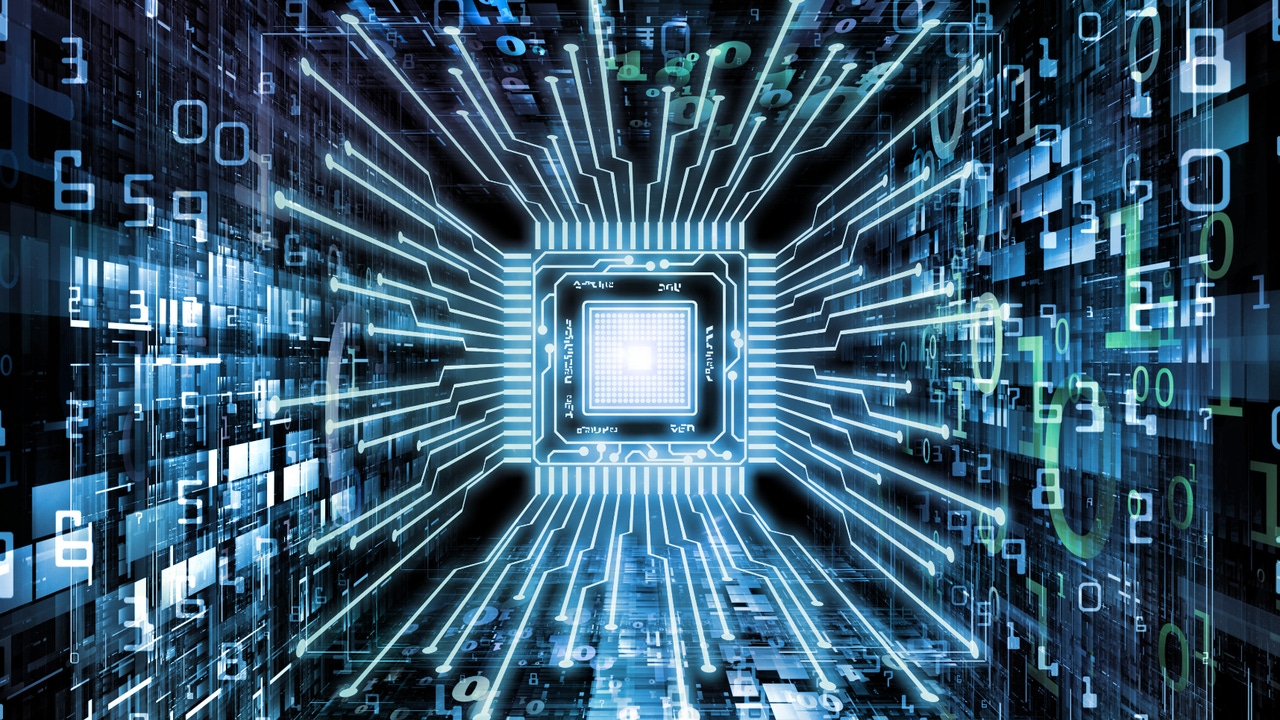
By Kevin Cochrane, Vultr
As AI operations (AIOps) continue to mature, organizations are discovering the true value of enterprise AI. In a 2024 S&P Global Market Intelligence study commissioned by Vultr, 58% of the most mature AI adopters expected moderate or significant cost reductions and margin improvements, with similar expected improvements across other key business metrics. These positive results are only poised to accelerate as generative AI evolves into agentic AI, moving from merely answering prompts to executing tasks autonomously.
However, this success doesn’t come free: Organizations in the most advanced stage of AI maturity also reported spending 24% of their IT budget on AI infrastructure, with 53% of these respondents expecting further increases in AI infrastructure spending. How can enterprise leaders be sure that the benefits outweigh the costs?
There’s no magic bullet to realizing ROI with AI, but there are certainly proven strategies for mitigating runaway costs for AI and optimizing its performance for your desired business outcomes. One important concept to understand is that optimizing AI costs isn't just about software — it's about matching the right AI workloads to the right compute resources.
Taking a strategic approach to hardware deployment addresses a fundamental truth in AI operations: Training and inference have vastly different computational requirements, and using the right resources for each can tame costs and optimize performance at each stage.
The Divide Between Training and Inference
AI model development and training represent the research and development phase of AI, demanding massive computational resources for relatively short periods. These workloads benefit from centralization in AI centers of excellence, where state-of-the-art GPU clusters can be fully utilized across multiple projects. These environments typically feature high-end GPUs like NVIDIA's H100 or AMD's MI300X, optimized for the parallel processing demands of training large language models and other complex AI systems. Unsurprisingly, this intensive stage is often the costliest part of the AI lifecycle.
In contrast, inference — the deployment phase where trained models interact with their end users — requires a different approach entirely. Inference workloads need to be distributed closer to data sources and users, often in edge data centers, where real-time processing is crucial to providing operational insights on demand.
For inference, the priority is to optimize for latency, performance, and cost — in sharp contrast to the training phase, where raw computational power is paramount. The distinct needs of each phase are best served with different computational resources, demanding a more diverse silicon strategy across the AI lifecycle to control costs and optimize performance.
Silicon Diversity: The Key to Efficiency
The concept of silicon diversity — using different types of processors for different AI tasks — has emerged as a crucial strategy for both performance optimization and cost control. While high-end GPUs excel at training, they may be overkill for inference workloads, where specialized inference processors or lower-end GPUs might provide better performance per watt and dollar.
Organizations are increasingly adopting a hybrid approach:
Training clusters utilize high-end GPUs optimized for parallel processing and high memory bandwidth.
Inference endpoints leverage a mix of CPUs, GPUs, and specialized AI accelerators.
Edge deployments might use compact, energy-efficient system-on-chip (SoC) solutions that combine CPU and GPU capabilities.
Serverless Inference Delivers Silicon Diversity
So, what does silicon diversity look like in practice within the enterprise AI infrastructure stack? The truth is that few enterprises can afford to procure the array of GPU and CPU resources needed to support generative AI or agentic AI at scale. Even if they did, their hardware investments would quickly become obsolete given the accelerated pace of innovation in AI infrastructure.
In reality, a serverless approach is the best way to effectively implement silicon diversity to control the costs of AI. Serverless inference leverages cloud-provider-managed resources to automatically manage and scale compute resources based on the AI workload and use case requirements, making it easier to deploy AI capabilities across distributed edge locations.
In other words, infrastructure concerns are delegated to the people who deal with this and only this every day, optimizing resource allocation for cost and performance. This approach liberates organizations to focus on building effective AI applications for their desired business outcomes, rather than wasting resources on server maintenance.
Looking Ahead
As AI continues to evolve, the importance of matching silicon to specific AI workloads will only grow. Organizations that master the art of silicon diversity — deploying the right computational resources for each phase of the AI lifecycle — will be better positioned to deliver efficient, cost-effective AI solutions. The future of AI infrastructure lies not in a one-size-fits-all approach, but in the thoughtful orchestration of diverse computing resources, from the data center to the edge.
About the author:
Kevin Cochrane is the Chief Marketing Officer at Vultr. He is a pioneer with over 25 years in the digital marketing and digital experience space. Kevin works to build Vultr's global brand presence as a leader in the independent cloud platform market and composable infrastructure for organizations worldwide.
About the Author
You May Also Like