Secret to AI Profitability Is Hiring a Lot More Doctorates
From spotting weeds in cotton fields to scanning the bush for signs of poachers, AI startups are recruiting scores of experts for highly specialized tasks.
December 10, 2024
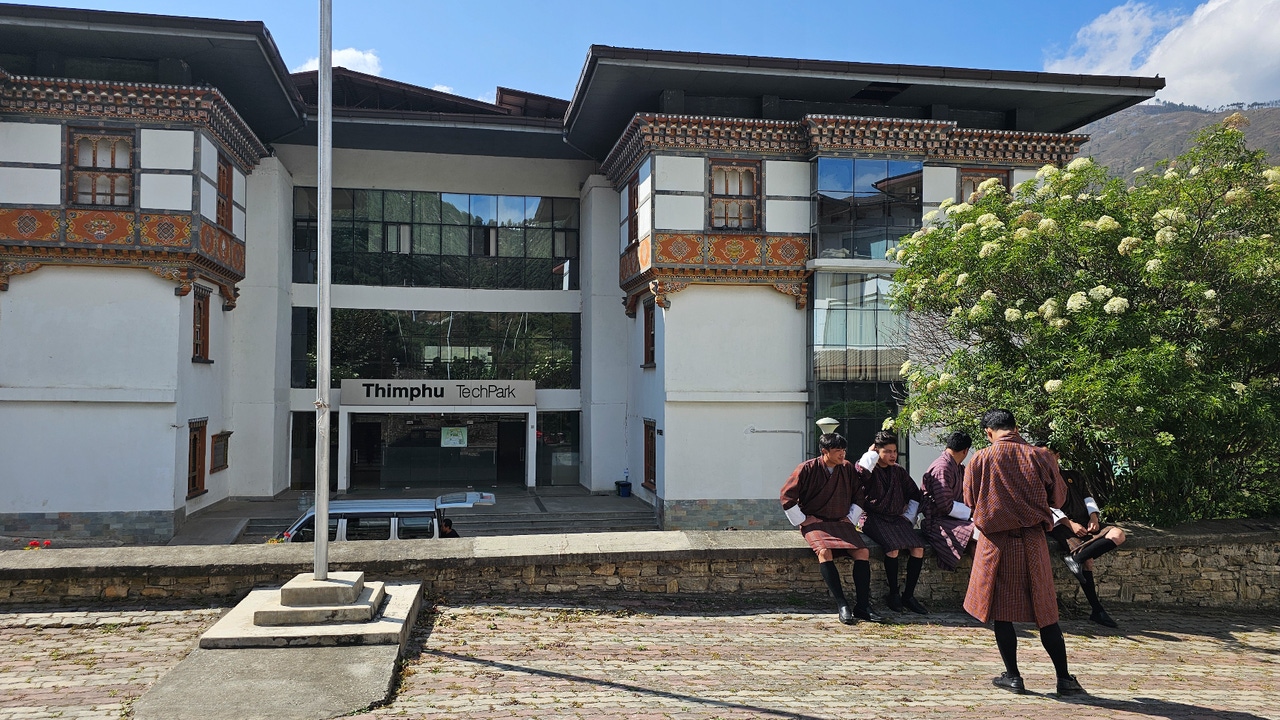
(Bloomberg) -- In the tiny kingdom of Bhutan, dozens of data experts perfect artificial intelligence models from offices framed by majestic Himalayan peaks. The employees at iMerit aren’t there to train AI in rudimentary tasks like recognizing “brown cat on a windowsill” in an image. Instead, they’re teaching algorithms the anatomy of the human eye or how to detect changes in geospatial maps.
Backed by three Silicon Valley billionaires, iMerit is part of a growing cohort of companies building a more sophisticated, monetizable and reliable version of AI, an industry on track to add nearly $20 trillion to the global economy by 2030. As models become smarter, big business is increasingly looking to harness their power for highly specialized tasks, spawning dozens of data services startups devoted to customizing applications across sectors like finance, health care and defense.
There’s a lot at stake. Even as AI fervor has swept through Silicon Valley, nagging questions persist about whether the technology will actually prove useful enough for businesses around the world to pay up for it and ensure that AI model developers can turn a profit. Of course, Nvidia Corp. has become the most valuable company in the world by selling AI chips. But the firm’s biggest customers, including Microsoft Corp. and Alphabet Inc., are still losing money from the immense cost of building more advanced AI systems.
Radha Basu, the founder and chief executive officer of iMerit, drew a parallel to software coders who built the internet, mobile phones and other modern tech platforms. “We’re coder equivalents of the AI revolution,” said the gray-haired entrepreneur, who’s preparing to raise her next round of funding.
Getting AI to advanced proficiency in unrelated, sensitive and sometimes dangerous industries won’t be easy. The undertaking requires a deep bench of human experts willing to add to their day jobs by training and improving models in technical fields.
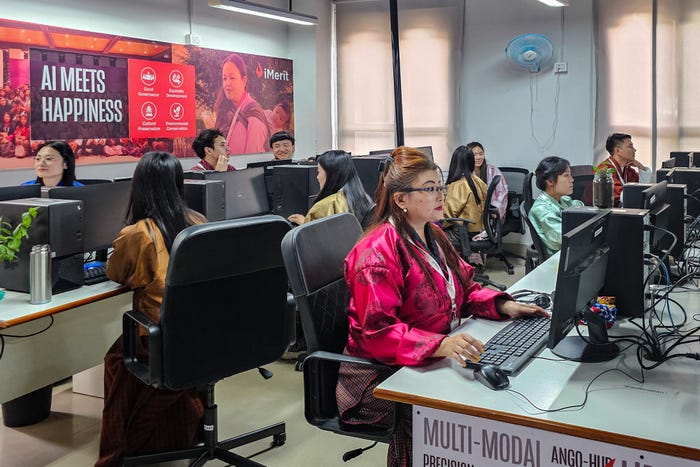
iMerit office in Thimphu, Bhutan, in October. Photographer: Saritha Rai/Bloomberg
In Kenya, a startup is developing technology to scan the bush for signs of poachers. In Kazakhstan, medical experts are teaching models to identify the early stages of lung cancer. In India, Korea, Vietnam and elsewhere, linguists earning $65 an hour are helping models become proficient in languages other than English.
At iMerit, which employs 5,000 people in Bhutan, India and New Orleans, Yeshi Wangmo, 23, who hails from a family of farmers, has spent years mastering a single task: correctly identifying weeds and debris in images of vast fields of corn and cotton. Wangmo and her colleagues, dressed in colorful Bhutanese gho and kira wraps, help companies like Blue River Technology, a subsidiary of Deere & Co., build algorithms that improve accuracy when spraying pesticides and fertilizers, reducing use by as much as 90%.
“We are seeing companies tackle more advanced but also increasingly niche problems,” said Ivan Lee, founder and CEO of data labeling solutions firm Datasaur Inc., whose customers include Netflix Inc. and the FBI. “Clients may need dentists who grew up in Tanzania or architects from France,” said Lee, whose teams mainly work out of Indonesia.
Data accuracy is the lodestar of their work. When ChatGPT was launched two years ago, critics were quick to pick apart the platform’s flaws and lapses. Since then, scores of human experts have been hired for quality control. The work is painstaking. Data labelers like Wangmo pore over scans, photos, video and text to ready AI models. The goal is to improve generative AI systems that are trained on vast data sets to analyze or create new content. Perfecting them removes the discrepancy between potential capabilities of AI and its actual performance in the real world.
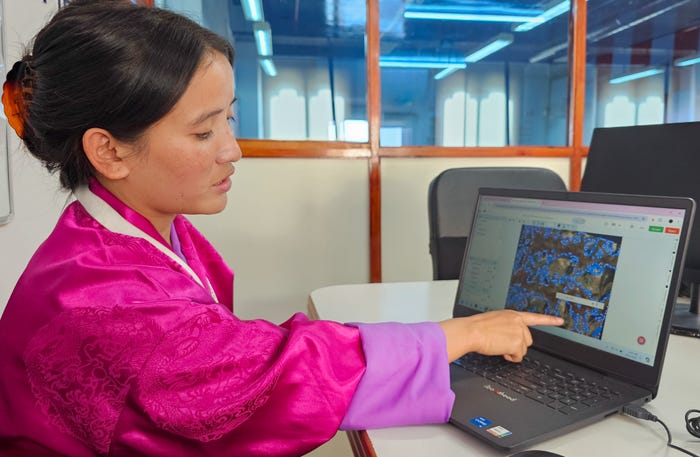
Yeshi Wangmo has spent years mastering correctly identifying weeds and debris in images of vast fields of corn and cotton. Photographer: Saritha Rai/Bloomberg
Such specialization is increasingly key in high-stakes sectors like those that deal with military intelligence, according to Kathleen Walch, director and general manager at the research firm PMI Cognilytica.
Lower-level versions of this work aren’t new. The data services industry began about two decades ago. Back then, labelers living in places like the Philippines and India primarily tagged small data sets that underpinned, for instance, speech recognition for voice assistants or search engines on shopping websites. Critics worry that AI has created an exploitable underclass, pointing to salaries that hover around a few dollars a day in some pockets of the industry.
But over the years, as AI has improved, much of the simpler stuff is now automated. Demand has shifted to recruiting specialists and paying higher salaries and rates, though they’re still considerably lower than compensation packages for data scientists in Silicon Valley.
In India, a radiologist training AI models might earn pay of 100,000 rupees ($1,200) for a few hours of work, said Hardik Dave, founder and chief executive of Indika AI, a popular data labeling firm. The average contractor makes about a third of that a month, he said.
Today, startups selling labeling services attract marquee investors. This summer, the largest player, Scale AI, raised money from Meta Platforms Inc. and Amazon.com Inc.. With a nearly $14 billion valuation, the company has vaulted past the figures for prominent AI model builders like Mistral and Cohere. In 2023, Sequoia’s list of the top 50 AI companies featured four labeling startups, up from just one the previous year. One firm, Labelbox, is backed by Andreessen Horowitz and Kleiner Perkins. Another, Snorkel AI, is funded by Alphabet Inc.’s venture arm at a valuation of $1 billion.
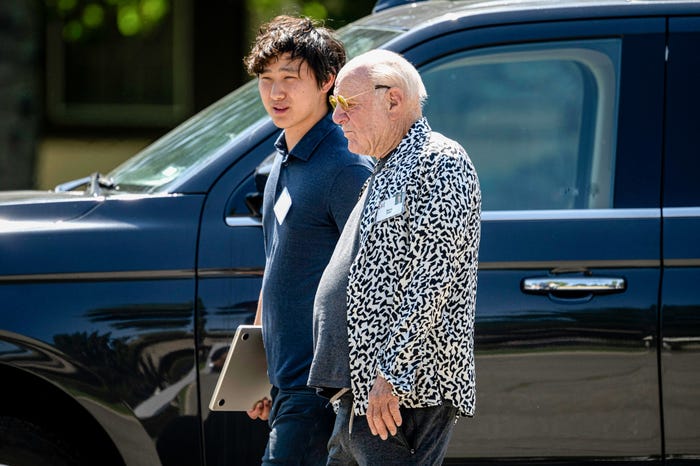
Alex Wang, co-founder of Scale AI, left, at Allen & Co.’s Sun Valley Conference in July. Scale AI raised money from Meta Inc. and Amazon.com Inc. Photographer: David Paul Morris/Bloomberg
More broadly, the market for data labelers, valued at nearly $20 billion in 2024, is projected to grow around 20% annually until 2030, according to Grand View Research, a market research firm based in San Francisco.
The consequences of a misstep are also weightier. A mislabeled frame could cost a business millions of dollars, inviting lawsuits or even causing death. Cancer-scanning AI tools or self-driving cars are two sensitive areas.
“Less accurate AI can go off the rails,” said Wendy Gonzalez, CEO of the Los Gatos-based Sama, whose clients include Ford Motor Co. and Walmart Inc. “Businesses can’t afford that.”
Consider the tie-up between Massachusetts General Hospital and Centaur Labs, a data labeling startup with 50,000 freelancers based in countries including the US, Kazakhstan and Vietnam.
In recent years, Boston-based Centaur Labs has improved products used in the hospital, gradually bringing in higher-skilled data experts. Some are related to everyday maladies. (The startup is working on a snore-detection algorithm and an app for sleep apnea.) Others stray into heavier topics like developing AI that can more precisely identify lung nodules in CT scans. Last month, the startup announced an injection of capital from Accel, Y Combinator and others.
Polina Pilius, a radiologist in Kazakhstan who oversees teams for a contractor of Centaur Labs, said the work keeps getting narrower. Today, she said, it’s not enough to merely detect lung nodules. Clients increasingly want specialized features that reduce the number of false positives and track the growth of nodules over time. Reducing risk without cutting corners is the sweet spot.
“Medical data annotation is a complex process that cannot tolerate haste, incompetence, inattention or excessive cost-cutting,” Pilius said.
Despite all that can go wrong, AI’s proponents argue that training models to tackle complex issues in risky sectors is preferable to doing nothing. In many instances, there’s only upside, they say.
Labelbox, the San Francisco-based startup, works with a client that sells dash cam analysis to companies overseeing hundreds of thousands of trucks. Over the past year, Labelbox data specialists have trained AI bots to become even more expert at monitoring whether a driver is drowsy or inebriated. Once detected, fleet operators are alerted and the driver is contacted.
Manu Sharma, the CEO of Labelbox, said this is just one example where models are doing more than simply cutting costs or improving efficiency. The best technology is life-saving, he said, and data labelers are on the front-line of advancing AI’s capabilities.
They’re “creating a world in which their expertise is more accessible and can be applied to benefit society,” he said.
About the Author
You May Also Like