A Guide to Storage for AI WorkloadsA Guide to Storage for AI Workloads
Learn how AI-optimized storage solutions can enhance performance, reliability, and cost-efficiency for AI workloads by providing the right mix of scalability, speed, and data flexibility.
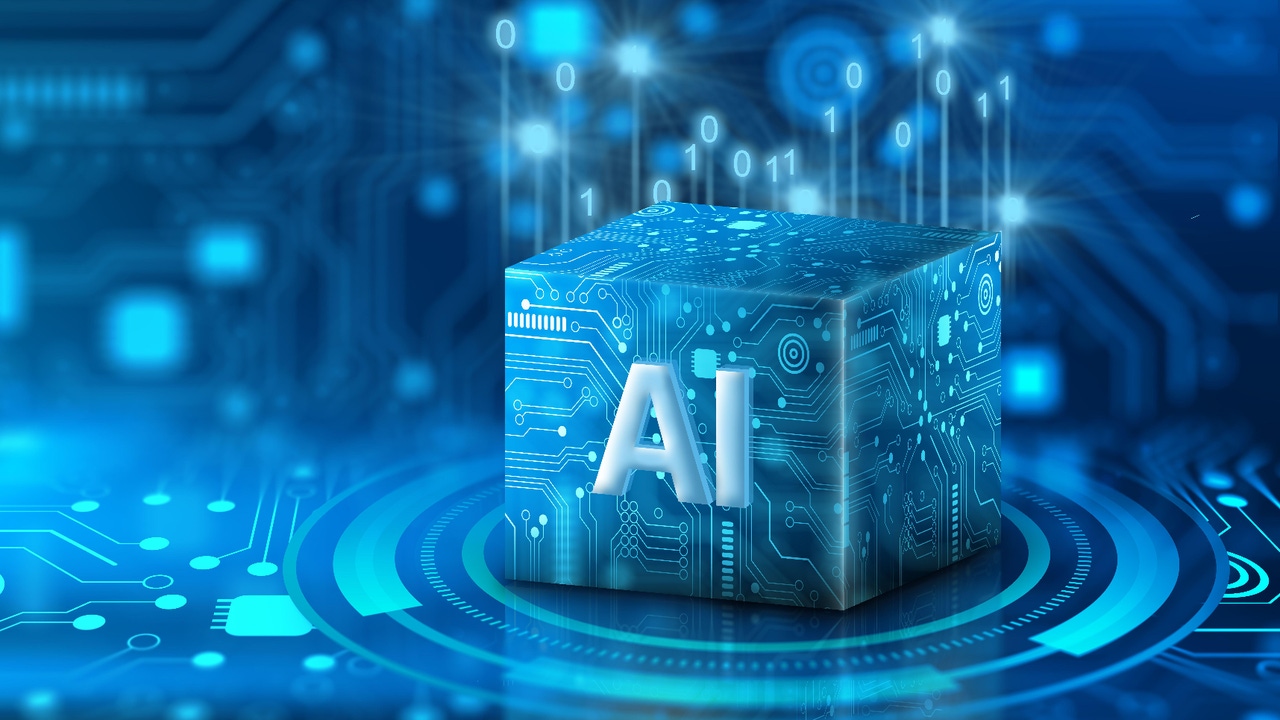
You may know that AI workloads can benefit from specialized compute resources, like GPUs or FPGAs. But did you know that AI-optimized storage, too, is a major consideration for deploying cost-effective, high-performing AI workloads?
If not, this article's for you. Read on for guidance on the role of storage in AI workloads and which storage options can optimize AI performance, reliability, and costs.
AI and Storage: The Basics
Like all workloads that store data persistently, AI needs storage — although the way AI uses storage can vary between different types of AI workloads. Common examples of storage needs for AI include:
Generative AI workloads need to store training data while models train.
Once trained, generative AI models may temporarily need to store data while performing inference (the process through which models generate content based on novel input).
Descriptive and predictive AI models rely on storage to house the data they analyze (such as log files or documents) when looking for patterns.
On balance, it's important to note that not all AI workloads require significant quantities of persistent storage. For example, you could deploy a descriptive AI tool that ingests real-time streaming data, analyzes it in real time, and then writes the results to volatile memory (i.e., RAM). In this case, the AI wouldn't need to store any information persistently and so it wouldn't need storage.
Most AI workloads, however, are likely to require substantial storage resources.
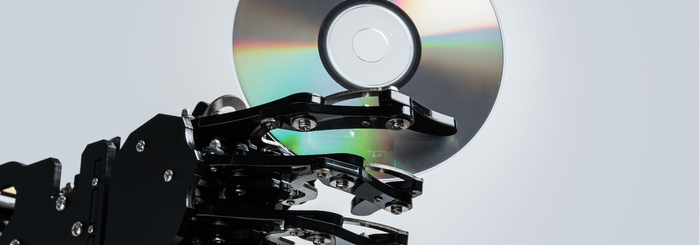
CREDIT: ALAMY
Optimizing Storage for AI
In general, any storage type can support AI workloads. To perform optimally, however, AI tools and services benefit from storage that delivers the following capabilities:
High I/O rates, which allow AI workloads to process data faster.
Scalability, so that AI workloads don't stop running due to lack of storage space.
Support for diverse types of data (such as structured and unstructured data) since some AI workloads will need to access multiple types of information.
The ability to compress data where feasible to reduce storage costs.
Support for data backup and recovery to mitigate the risk of data loss.
Here again, specific storage requirements will vary between different AI workloads. For example, high I/O is less important for generative AI model training than for real-time AI analytics because delays are typically acceptable when training models.
But the point is that, in general, AI workloads work best when they can access specialized types of storage.
AI Storage Options
Options for provisioning AI workloads with storage vary widely. Here's a look at common approaches.
Cloud storage
Cloud storage, like the object storage services provided by all the major public clouds, offers the benefit of massive scalability. They are also low in cost, and in the case of object storage, they can accommodate virtually any type of data. (File-based cloud storage services are less flexible in this respect, as noted below.)
However, reading and writing data may take longer due to network latency and bandwidth limitations — so cloud storage is not ideal for AI workloads that require the highest possible I/O rates.
On-premises commodity storage
An on-premises storage array that consists of conventional hard disks is relatively inexpensive to build and maintain. It's also relatively high-performing because it provides AI workloads with direct access to storage resources. The major downside is that scalability is limited because you can't quickly add more storage resources.
Solid-state storage
Storage arrays built using solid-state disks offer better performance than conventional hard drives, but they're more expensive. They make sense for AI workloads where I/O is a top priority.
Choosing Object vs. File-Based Storage for AI
In addition to selecting storage infrastructure, organizations that deploy AI must choose the type of storage system to use. Object storage — available in the cloud but configurable for on-prem using solutions like OpenStack Swift — is typically the most flexible because it lets you store any data type. Object storage is ideal when you don't know which data types you'll expose to AI services. For instance, object storage is likely your best option if you train a generative AI model using a vast trove of information of varying types.
However, file storage typically makes more sense for AI workloads that need to store data in a structured or ordered way. For example, file storage may be most appropriate if you are deploying a predictive AI tool whose main job is to analyze structured log files and write the results to other files.
Conclusion: The Future of AI Storage
To date, storage optimization for AI has not been a major focus of storage vendors. But as AI deployments continue to grow, expect to see more investment in storage solutions designed specifically for AI. The diverse storage needs of AI workloads mean that there is no one-size-fits-all solution, but storage options that cater to the needs of AI will make it easier to deploy AI workloads without letting storage become the weakest link.
About the Author
You May Also Like