Unlocking DataOps: New Report Highlights Gaps and Opportunities
A new study finds enterprises underutilize emergent data, face skills gaps, and need better DataOps. Improved strategies can unlock value from AI and emerging tech.
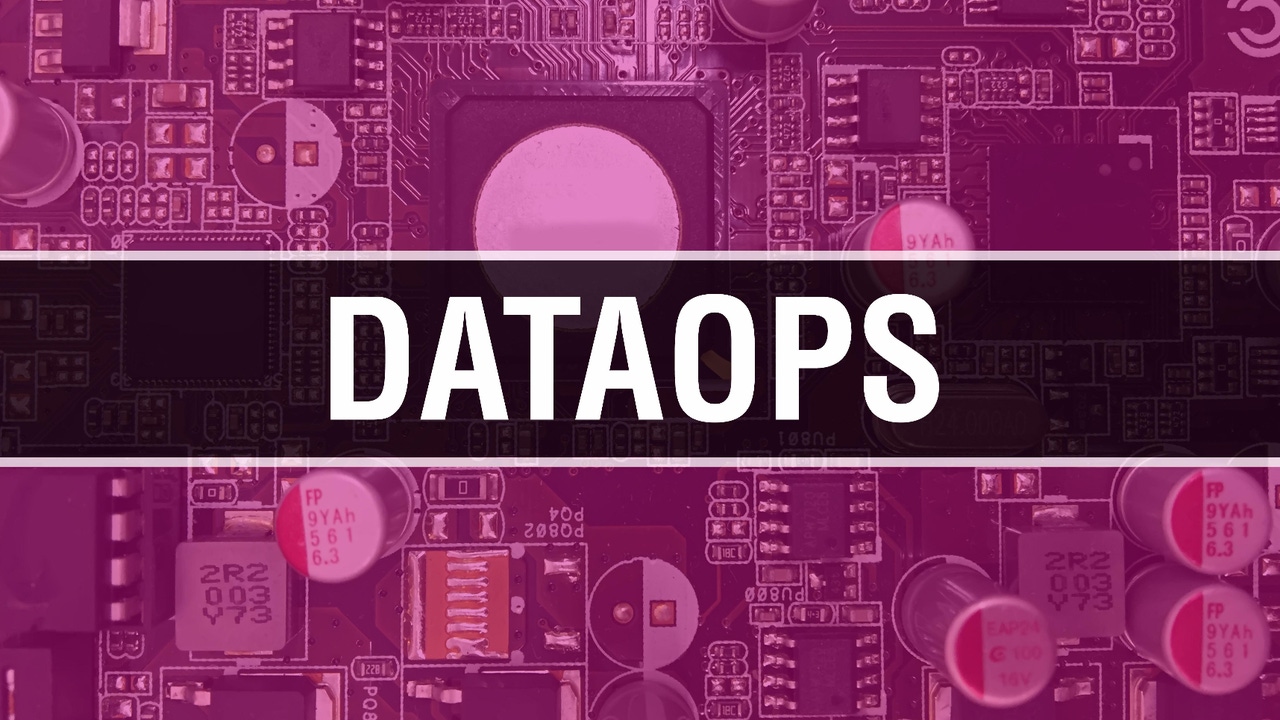
The current state of DataOps leaves a lot to be desired according to a new report.
A comprehensive study titled "Putting the 'Ops' in DataOps: Success Factors for Operationalizing Data" sheds new light on the state of data management and DataOps in large enterprises worldwide. Commissioned by BMC and conducted by 451 Research, part of S&P Global Market Intelligence, the survey involved 1,100 IT, data, and business professionals from large enterprises across 11 countries.
Key findings reveal DataOps opportunities and challenges, including:
Emergent data underutilization: Only 17% of data ingested consists of emergent data types, with a mere 9% being processed or analyzed.
Skills gap: 48% of respondents cite lack of skills as a major challenge in data management.
Human error and automation deficit: 43% report both human error and lack of technology automation as key obstacles.
Pipeline orchestration lag: Even among organizations with exceptional data maturity, only 41% report high maturity in data pipeline and application workflow orchestration.
DataOps maturity impact: 75% of organizations with mature DataOps practices have a chief data officer, compared with 54% with less mature practices.
Large enterprise advantage: 75% of organizations with 5,000+ employees actively manage data for generative AI initiatives, versus 50% of smaller organizations.
"One surprising finding from the survey was how few organizations are analyzing data of emergent data types," Jennifer Glenski, director of product management at BMC, told ITPro Today.
Glenski noted that even though organizations are looking to capitalize on the ever-growing data coming from new sources and technology, the study found that only 9% of data processed and analyzed is of emergent data types.
"This newer data could hold great value for organizations in initiatives like generative AI, LLMs, FinOps, or sustainability, if they are able to tap into it," she said.
Why Is DataOps So Hard?
There are numerous reasons to adopt a DataOps strategy — but unfortunately also many obstacles to doing so.
Glenski noted that for organizations looking to implement DataOps to get more value from their data, the biggest obstacles tend to be a lack of both skills and technology automation.
Awareness is also a big issue.
Raising awareness of the numerous advantages that DataOps offers — such as using automation to enhance existing skills and reduce human errors — can help organizations prioritize developing a DataOps strategy tailored to their needs, she said.
The study defines four levels of data management maturity: developing, functional, proficient, and exceptional. Organizations at higher maturity levels tend to achieve better success in data-driven activities.
However, the research reveals a curious trend: Proficiently mature organizations often experience a dip in self-reported performance, likely due to increased awareness of their challenges as they grapple with growing complexity.
How to Improve DataOps
The report offers several key strategies for organizations looking to improve their DataOps practices:
Implement automation: Increase the use of technology automation to address human error and skills shortages.
Enhance data pipeline orchestration: Focus on improving data pipeline and application workflow orchestration, even for organizations with high data maturity.
Invest in skills development: Address the skills gap through training and recruitment of data management professionals.
Align DataOps with business outcomes: Ensure DataOps practices are directly linked to critical business outcomes.
Adopt a tailored approach: Implement strategies based on your organization's current maturity level, as outlined in the report.
Leverage emergent data: Increase efforts to ingest, process, and analyze emergent data types to support innovative initiatives.
Establish strong leadership: Consider appointing a chief data officer to drive DataOps maturity across your organization.
"To improve, organizations should strive to link their data management strategy, practices, and architecture to critical business outcomes," Glenski said. "It also helps to have an individual or group responsible for driving DataOps strategy and practices across an organization to help unlock value at scale."
About the Author
You May Also Like