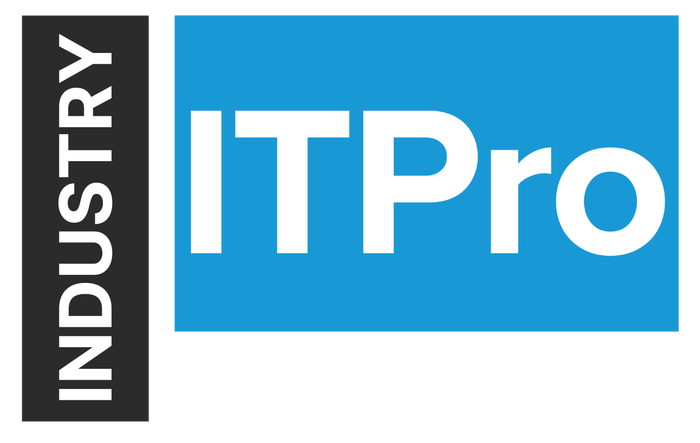
Insight and analysis on the information technology space from industry thought leaders.
Optimizing AI Workflows for Hybrid IT EnvironmentsOptimizing AI Workflows for Hybrid IT Environments
Hybrid IT environments offer businesses the flexibility to optimize AI workflows. Follow these to fully harness the power of AI to drive innovation and business growth.
December 24, 2024
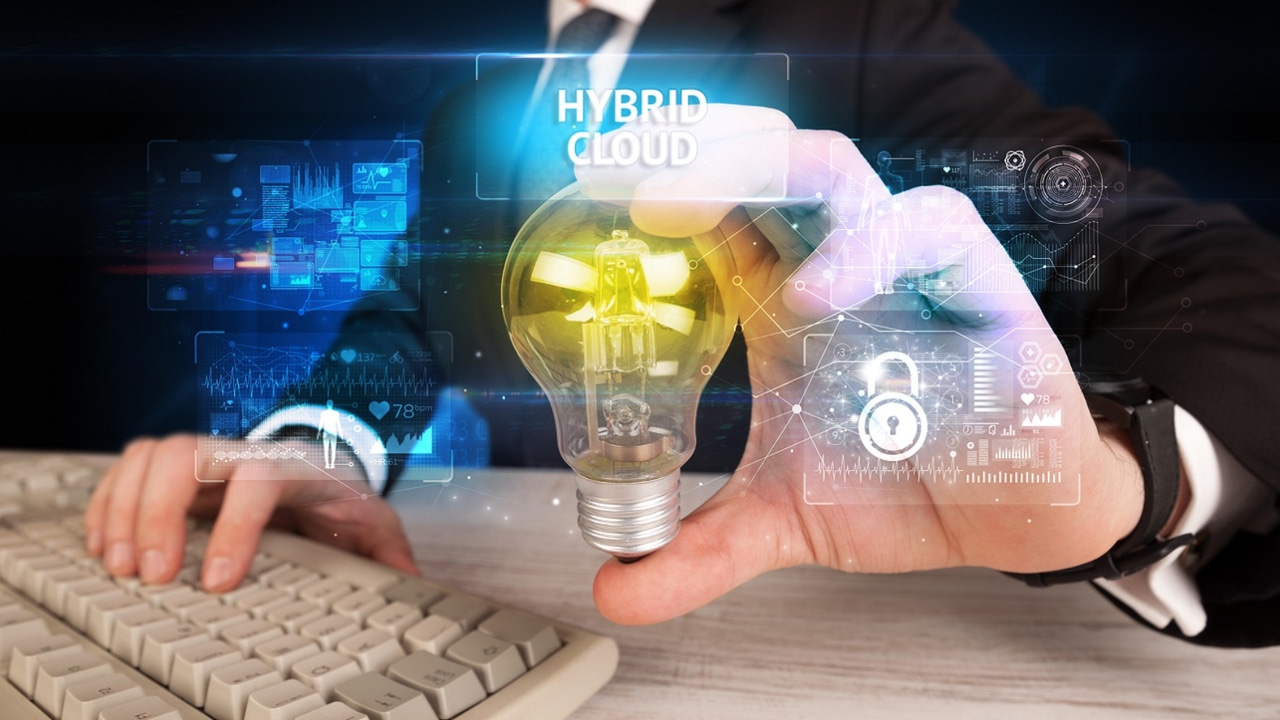
By Gary Espinosa
Hybrid IT environments, which blend on-premises infrastructure with public and private cloud resources, present a new set of challenges and opportunities for businesses aiming to optimize AI workflows and improve their data management practices.
The need to ensure seamless integration across diverse platforms while managing data efficiently and maintaining performance is at the heart of this challenge. Thus, in this article, we'll explore exactly what it takes to establish an AI workflow, optimize it, and integrate it within the context of hybrid IT environments.
Understanding Hybrid IT Complexity
Hybrid IT offers flexibility by combining the scalability of the cloud with the control of on-premises resources, allowing companies to allocate their resources more precisely.
However, this setup also introduces complexity. Managing data flow, ensuring security, and maintaining operational efficiency across such a blended environment can become an overwhelming task if not addressed strategically.
To manage AI workflows effectively in this kind of setup, businesses must focus on harmonizing infrastructure and resources. After all, hybrid IT's advantage is flexibility — being able to decide where to host your workloads for optimal results.
This is particularly important for AI workflows that are often computationally intensive and data-hungry. Ultimately, the decision in which direction to pivot depends on the potency of the AI models in use, as well as any supplementary infrastructure.
Unified Data Management for AI Success
Although 35% of all businesses have adopted AI into their workflows, they're still lacking when it comes to proper data handling. More than anything, effective data management is key to optimizing AI workflows, making the issue even more concerning.
In a hybrid IT environment, data is often spread across various locations — on-premises servers, public cloud instances, and private cloud setups. Ensuring this data is accessible, synchronized, and secure is critical. Of course, the complexities multiply when specific cloud automation workflows are introduced to the fold.
One practical approach is adopting a unified data management strategy. This means implementing tools that enable seamless data integration across all your environments. Data virtualization solutions are particularly effective, allowing access to data without physically moving it. This helps minimize latency, maintain consistency, and support real-time analytics, which is essential for AI models that need to operate on up-to-date information.
AI workflows are data-driven, and inconsistencies can derail a project. Leveraging data orchestration platforms helps automate the process of moving and transforming data across environments, ensuring that the right data is available at the right time for AI model training and inference.
Optimizing Resource Allocation with Hybrid IT
It's high time we addressed the elephant in the room — the sheer price of having access to AI resources. A server with eight flagship Nvidia H200 AI GPUs will set you back in excess of $300,000, while a single H100 is around $30,000.
Don't even get us started about how even consumer-grade cards, such as the RTX 4090, have jumped in price. There's no more denying that AI hardware has gotten prohibitively expensive. So, what's the solution?
Those with a head start have already hoarded plenty of GPU, being fully aware that AI workloads require significant computational power, and resource allocation is crucial for ensuring optimal performance. Hence, more and more companies are deciding to rent GPU servers, both due to the lower price and not needing to set everything up themselves.
Simply put, training and fine-tuning large AI models may be more efficiently handled on cloud-based GPU instances, where computational resources can be scaled up or down depending on demand. However, there's always the issue of third parties having access to your data analytics and AI workflows.
At the same time, inference tasks, which may require lower latency, could be performed on-premises. This mixed strategy allows businesses to optimize costs while also ensuring that the necessary computational resources are available for different stages of the AI workflow.
Streamlining Deployment with Cloud Automation
Cloud automation plays a significant role in optimizing AI workflows. In particular, automation tools help streamline the deployment, management, and scaling of AI models across hybrid environments. With the reduction of manual intervention, businesses can minimize human error, save time, and ensure consistency throughout their workflows.
For instance, using Infrastructure as Code (IaC) tools like Terraform allows for the creation of repeatable, consistent deployment processes that are easily maintained and updated. Automated container orchestration tools like Kubernetes can manage containerized AI workloads across both on-premises and cloud environments, ensuring that resources are utilized efficiently.
At the same time, cloud automation can also improve model retraining cycles. By automatically scaling resources during training and then scaling down once the task is complete, you can optimize your use of both on-premises and cloud infrastructure, effectively managing operational costs.
Security and Compliance Considerations
Security is another major concern in hybrid IT environments, particularly when handling AI workflows that require access to sensitive data. A robust security strategy needs to address data encryption, secure access controls, and compliance requirements across all environments — whether on-premises or in the cloud.
That's why it's essential to adopt a zero-trust security model, as it ensures that each access request is continuously validated, no matter where the workload is hosted. It's even more crucial if you're running closed-source LLMs, as it's impossible to know about all the exploitable vulnerabilities.
This is especially important in hybrid environments where data moves across different networks and systems, making it more vulnerable to breaches. Moreover, hybrid IT environments also benefit from unified monitoring tools that provide real-time insights into potential vulnerabilities across all platforms.
Performance Optimization and Monitoring
Performance optimization is crucial when running AI workloads across hybrid environments. This requires real-time monitoring of both on-premises and cloud systems to identify bottlenecks and inefficiencies. Implementing performance management tools allows for end-to-end visibility of AI workflows, enabling teams to proactively address performance issues before they escalate.
Monitoring tools that integrate with both on-premises infrastructure and cloud services are essential in hybrid setups. They offer insights into workload distribution, resource usage, and system health, which can be used to dynamically adjust resource allocation and improve efficiency.
When it comes to AI workloads, ensuring your infrastructure is continuously optimized can significantly impact model performance and the speed at which insights are generated.
Ensuring Scalability and Agility
One of the primary benefits of hybrid IT is scalability — especially important for AI, where the need for computational power can vary dramatically depending on the specific tasks being executed. Using a combination of both cloud resources and on-premises infrastructure, you can quickly scale up during model training and scale back during periods of lower activity.
Scalability also supports agility, which is crucial for businesses that need to grow and iterate on AI models frequently. Cloud-based services, in particular, allow teams to experiment and test AI models without being constrained by on-premises hardware limitations. This flexibility is essential for staying competitive in fields where AI innovation happens rapidly.
Conclusion
Optimizing AI workflows for hybrid IT environments involves striking a balance between leveraging the benefits of cloud scalability and maintaining the control offered by on-premises systems.
By focusing on unified data management and effective resource allocation with GPU hosting, and leveraging cloud automation, businesses can achieve a seamless AI workflow that maximizes efficiency, reduces costs, and enhances performance.
Hybrid IT offers an ideal infrastructure for modern AI demands, but it requires thoughtful planning, the right tools, and a clear understanding of how to manage and optimize across multiple environments. With these strategies in place, organizations can fully harness the power of AI to drive innovation and business growth.
About the author:
Gary Espinosa is an expert writer with over 10 years of experience in software development, web development, and content strategy. He specializes in creating high-quality, engaging content that drives conversions and builds brand loyalty. He has a passion for crafting stories that captivate and inform audiences, and he's always looking for new ways to engage users.
About the Author
You May Also Like