The End of the Full-Stack Data Scientist
Organizations should start to rethink how data science experts collaborate and communicate with each other, but a tight IT market means offering opportunities other businesses won't.
February 2, 2023
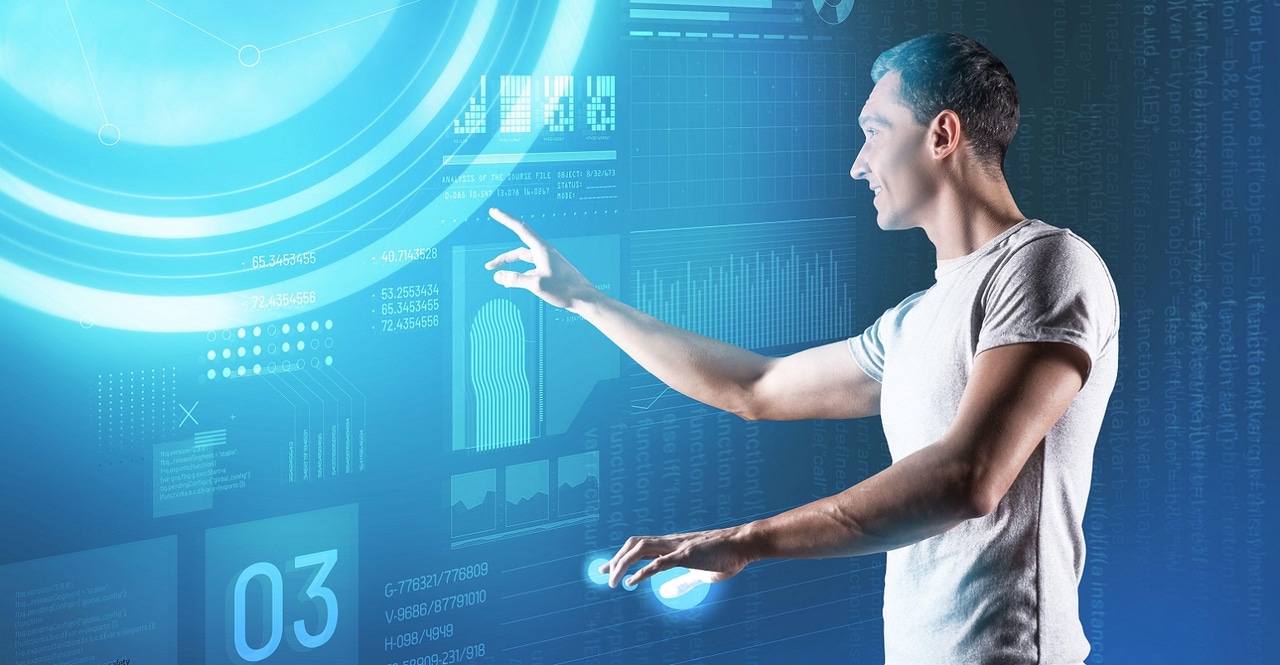
The notion of a full-stack data scientist who executes on all aspects of the data science model lifecycle — from building the data pipelines to developing the machine learning model to deployment and subsequent monitoring of the model — is quickly becoming outdated.
The idea of a "full-stack" data scientist comes from the fact that it requires so many different skill sets to drive business value from data science, and it's also a concept that originates from the courses currently being offered by universities.
As part of their degrees, budding data scientists studying for their master's or Ph.D. would often have to find their own data sets, engineer them for use, and then implement their own data science methods before finally presenting the results to their supervisors.
Related:Data Scientist Careers Are Evolving — Here's Why
Essentially, the whole thrust of a degree in data science was to create a full-stack data scientist.
Subsequently, when it came to hiring, data leaders saw an opportunity to recruit all-in-one/full-stack data scientists.
"I refer to [full-stack data scientists] as 'chimeras' both because they are combinations of many unique roles, but also because they are rare, bordering mythical. To the extent they do exist, they are hard to find, expensive, hard to retain, and usually not very productive across the domains."
— Kjell Carlsson, head of data science strategy and evangelism, Domino Data Lab
This was seen as too good an opportunity to pass up as it solves two important problems: Firstly, data engineers who sit in the IT department are often unable to engineer data in the way the data department wants or at the speed data leaders need it.
Secondly, at the other end of the stack, data visualization is often carried out by non-technical employees using business intelligence (BI) software, which doesn't always present data in the way data scientists envisioned it.
A full-stack data scientist, therefore, can take both tasks "in-house" into the data department.
Data Science Projects Stumble on Integration
As Peter Jackson, chief data and product officer for Outra, points out, a very high number of data science projects never actually get into operation, primarily because of the lack of integration with the wider business.
"This is often because of full-stack data scientists trying to complete projects wholly within their own department, segregating them from the rest of the business," he said. "To improve data operations, organizations have realized that they need to integrate data projects from engineering right through to sales."
Traditionally, a data project has several layers, starting with data engineers, working its way through to data scientists, then product owners, then the marketing team who work out how to sell the data product, and finally the sales team, according to Jackson.
"These tend to work in silos, so if an organization is specializing its data scientists to give projects a better chance of getting off the ground, it needs to ensure all of these areas work together to understand what each stage of the project needs from each other," he said.
It was never feasible for one individual — a "full-stack" data scientist — to perform all these different roles, let alone scale data science efforts by relying on these types of individuals, said Kjell Carlsson, Domino Data Lab's head of data science strategy and evangelism.
"I refer to these folks as 'chimeras' both because they are combinations of many unique roles, but also because they are rare, bordering mythical," he said. "To the extent they do exist, they are hard to find, expensive, hard to retain, and usually not very productive across the domains."
As enterprises, and the industry, have matured, folks have realized that they need a new paradigm, one more akin to industrial-scale production versus the "artisanal" model of data science still seen at companies just getting started, Carlsson said.
Leadership Is Essential to Transform Data Science Approach
Outra has flipped the traditional data operations pyramid on its side by putting together teams for any kind of data project in individual project groups, Jackson said.
This involves creating working groups that include everybody working on an individual data product, from the data engineers right up to the sales teams, so that they can collaborate on what it is they need from each other to create the best end-product.
"Essentially, instead of the bottom-up approach of feeding data to the products team and then feeding that product to the sales team, you create groups where data consumers can dictate to data producers what it is that they need," he said.
Those data producers can see the final product to refine how they engineer the foundational data used.
From Carlsson's perspective, core to any form of business transformation is leadership.
"You need it to align different parts of the business to develop new ideas into feasible solutions, change existing business processes, and develop new ones," he said. "Data science is no different but, arguably, harder because so few leaders have meaningful experiences with data science."
In addition, organizations do not have a history of data science leaders — there aren't established leadership roles and career paths at most enterprises.
"Thankfully this is changing, and increasingly organizations are putting in place C-suite executives, often new CDOs [chief data officers], who have previously been data science leaders, as well as creating a leadership hierarchy — and the associated career path for growing executive data science talent," Carlsson said.
Keys to Effective Communication and Collaboration
For any data team to be successful, collaboration is key, and the most important thing any organization can do is to get the entire data stack into a single working group to collaborate, according to Jackson.
For data science teams, that means working with engineers to explain what they need from the data, and with product owners and sales teams to understand what it is that the market wants from them.
"The most effective way of doing this is in-person meetings," Jackson said. "Project management tools are all well and good, but to properly break down silos it's important the different layers are able to collaborate as a single team sitting next to each other in a single space."
Carlsson said that while there is technology that helps with communication — results sharing, goals/project tracking, commenting — most of the communication challenge requires the creation of new roles like the data science product manager and leadership roles empowered to align different parts of the business.
Related: 5 Ways for Data Scientists to Improve Communication Skills
"The reverse is true when it comes to collaboration," he said. "While it is important to develop better processes, it's even more important that organizations invest in integrated platforms that provide a system of record for the activities of different data scientists, across different teams, different tools, and different environments, for example, on-prem or different clouds."
If the organization cannot even track the different data science activities and results, let alone share, govern, and monitor them, it will be impossible for data teams to collaborate at scale.
The key, Jackson said, is to implement platforms that span the range of data science tools that teams use today and that are modular and extensible enough to incorporate and track the tools that data scientists will be using in the future.
Building a Data Science Team in a Tight IT Labor Market
"Data scientists want to feel both that they are an integral part of the business and that their products will go into operation," Jackson said.
"Organizations can attract better data scientists by improving their DEI offering to reflect the diversity of the data science talent pool and ensure new hires feel comfortable in their new business environment."
— Peter Jackson, chief data and product officer, Outra
From his perspective, organizations must do three things to attract the best data scientists: First, they should set up their operating model to allow data scientists to impact the business by breaking down silos and integrating data teams with the rest of the organization.
"Second, organizations can attract better data scientists by improving their DEI [diversity, equity, and inclusion] offering to reflect the diversity of the data science talent pool and ensure new hires feel comfortable in their new business environment," he explained.
Finally, organizations should ensure that the business has data credibility.
"We've all heard of greenwashing, but a lot of businesses engage in 'data science-washing' where they talk the talk about data but don't actually put it into action," Jackson said. "Data scientists can spot this from a mile off, so ensure your data operation is actually credible if you want the best data professionals on your team."
Far too many companies set themselves up for failure when it comes to hiring and retaining data scientists by setting out to hire chimeras — the so-called "full-stack" data scientists — and then not providing them with the tools and leadership they need to deliver business impact, Carlsson said.
"The only way for organizations to scale their data science teams and their impact is to support the needs of a diverse range of data scientists, enable them to be productive, lead and govern their activities, and accelerate the lifecycle of data science projects so that they deliver value quickly," he said.
This requires supporting a wide range of methods and tools that data scientists get trained on — whether open source tools like R and Python or proprietary tools like SAS and MATLAB — and automating the DevOps so that they can get access to distributed compute to develop effective models in a reasonable amount of time.
Organizations must also minimize the friction across the model lifecycle — from development through deployment, monitoring, and continuous improvement.
Carlsson said that by supporting a broad range of tools, businesses can hire from a much broader talent pool, and by enabling them to deliver business impact, they will have the ability to learn and achieve in a way they cannot at many, if not most, competitors.
"If you then also have a platform that enables them to use the latest and greatest data science methods in their regular work and give them access to cutting-edge hardware, you will have a hiring advantage that others can only dream of," he said.
About the Author
You May Also Like