How BlaBlaCar Reached Its Data Quality Management Destination
BlaBlaCar hit the brakes on planned business expansion due to data quality management issues. Learn how the Monte Carlo platform fueled BlaBlaCar’s data-focused journey.
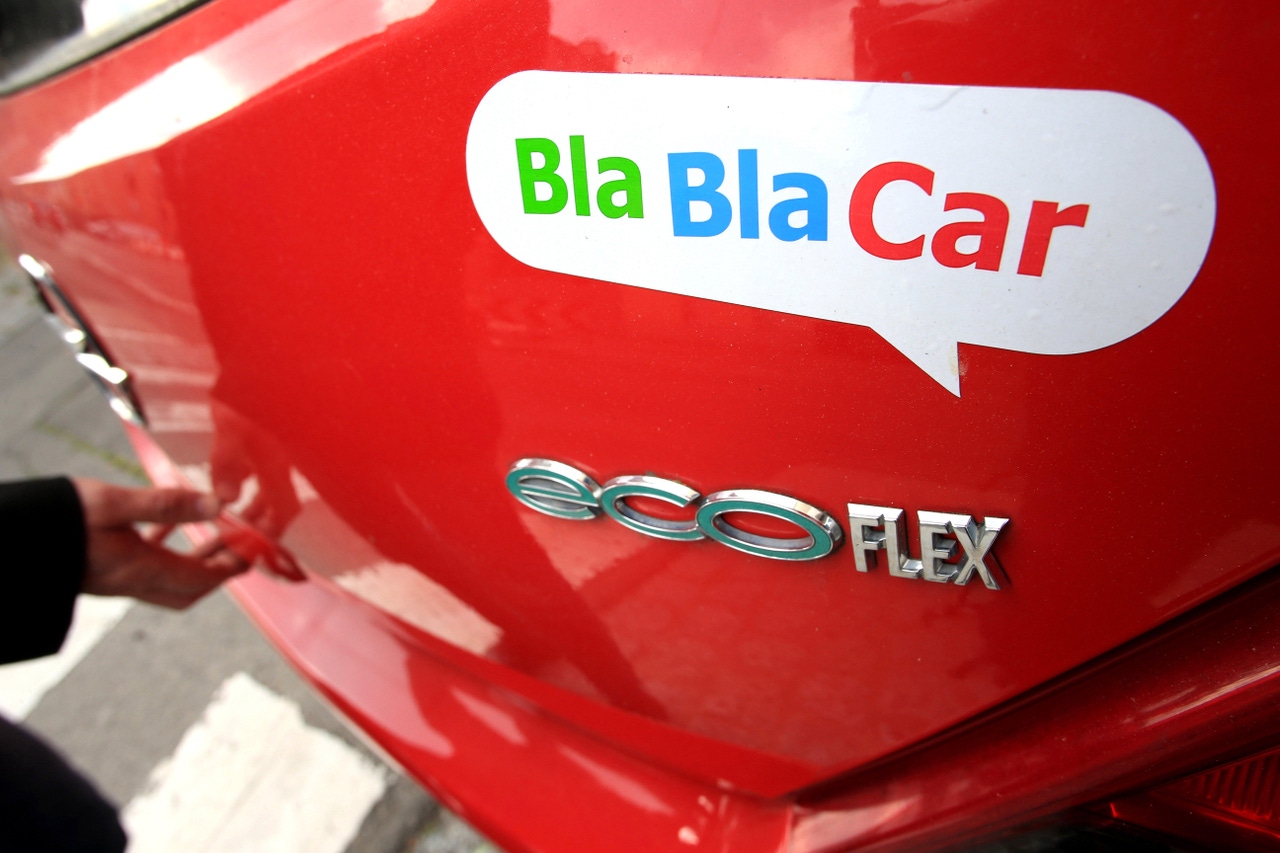
Just like “Uber” has become a verb for ride-sharing in the U.S., in Europe and other parts of the world, people have a similar term: “BlaBlaCar.” BlaBlaCar is a ride-sharing app that has grown into a major player during the past 15 years. Last year alone, more than 65 million carpoolers used the app in 22 countries, traveling an average of 200 miles per trip.
As BlaBlaCar became increasingly popular, its leaders worked hard to keep up with demand. For example, the company had always relied on a centralized data warehouse, but over time it ran into scalability and data management issues, which called for a new approach. A few years ago, BlaBlaCar vice president of data Emmanuel Martin-Chave spearheaded a switch to Google BigQuery, a cloud data warehouse that enabled the company to store and analyze data without worrying about scalability. As the company expanded its options for users, like it did when it added buses to its carpooling options, the team would simply build additional data models on the same BigQuery platform.
While the move to BigQuery was beneficial, Martin-Chave soon realized that BigQuery couldn’t take the company much further on its own. A constant stream of mergers and acquisitions designed to grow BlaBlaCar meant finding a way to incorporate different datasets. Company growth overwhelmed efforts to keep data clean and accessible.
Roadblocks to Data Quality Management
Data quality management was a critical problem. The company found that it spent 200 hours per quarter searching for the root cause of data quality issues. About half of the data incidents were reported by data consumers, not staff.
Coordination was another major issue. “Sometimes we learned about changes made by backend teams after those changes were made. Other times, we found that data was incomplete, and by trying to fix the problem, we actually created more problems,” Martin-Chave explained. “So even though data-informed decisions create the best outcomes, it was a blind spot for us, because we didn’t have a lot of data on the quality of what we were producing.”
blablacar bus in parking lot
Martin-Chave also knew that without resolving these data quality management and inefficiency issues, BlaBlaCar would struggle to expand operations. For example, company leaders wanted to embrace AI and machine learning for better decision-making but couldn’t do so while hamstrung by data problems.
The solution, BlaBlaCar determined, was to break down the data warehouse’s monolithic structure. Martin-Chave wanted delineated domains with a single owner each within the BigQuery environment. He also wanted each owner to have full authority to make changes. But at the beginning of 2022, the team was still dealing with a huge spaghetti plate of relationships that needed to be untangled.
In addition, Martin-Chave also wanted to implement a data quality index (essentially a confidence index that would improve data quality so users could trust it) and gain a better understanding of the relationships between tables. “If we needed to add a column or remove a process from a table, we needed to know if it would create trouble down the road in different parts of the data warehouse,” he said.
Destination: Data Mesh
Those requirements led Martin-Chave to choose a data mesh-based technology that organizes data by specific business domain, which would promote better data quality, access, and self-service.
After benchmarking a few options, the company settled on Monte Carlo, a young company that provides a data observability platform. Not only does Monte Carlo’s technology let each domain control its data, but each domain owner can also define and track custom metrics.
Certain features of the Monte Carlo data platform influenced Martin-Chave’s decision. He noted the product’s out-of-the-box observability, which would provide BlaBlaCar with a comprehensive view of its data’s health, improving data reliability. He also liked Monte Carlo’s approach to alerting, which uses machine learning to automatically generate alerts based on historical data. The platform sends alerts to BlaBlaCar via Slack.
e0688b0-Screen_Shot_2022-04-11_at_7.45.23_PM
Another plus was how Monte Carlo handles data lineage. The platform traces root causes so users can understand why errors occur. “If I want to change table XYZ and I have 15 other tables that depend on that table, I want to make sure I’m not breaking anything before I make the change,” Martin-Chave said. With this feature, users can easily examine more than just basic lineage relationships to understand the relationships between fields and tables.
The Road Ahead
Since implementing the Monte Carlo data platform, BlaBlaCar has improved internal users’ perceptions of the data quality.
“We used to have data consumers telling us that something was broken or that a data source was giving them a strange result. Now our data team can detect and fix something before our data consumers even realize there was a problem,” Martin-Chave said. “And we can now refer to an objective source of truth.”
With the data mesh and data warehouse connected, BlaBlaCar can get on with growing the business. BlaBlaCar recently introduced an AI/ML component into its data stack to help with decision-making and analytics. On the consumer end, the AI/ML component will help users choose the right stopover on a journey to maximize the number of passengers. Internally, the component will help engineers develop a bus network and find the optimal number of buses.
“We want to get to the point where the system can make hundreds or thousands of automated decisions per second, and that couldn’t happen with poor data quality,” Martin-Chave said. “We had to invest in data quality to bring us to the point where we could advance.”
About the Author
You May Also Like