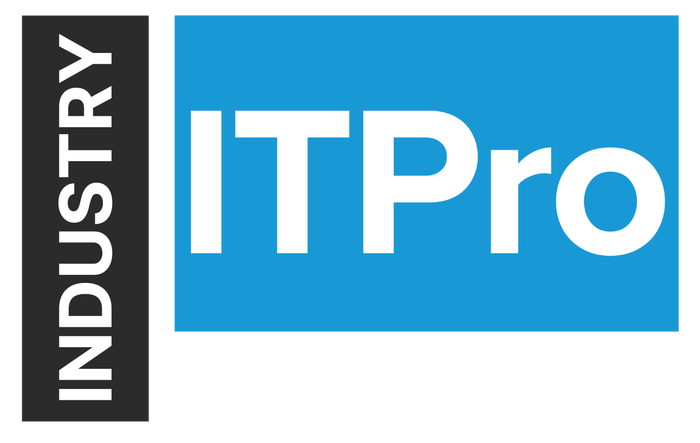
Insight and analysis on the information technology space from industry thought leaders.
What Makes Data Science Teams ThriveWhat Makes Data Science Teams Thrive
Data science has the power to transform your business. Here are some best practices to make sure your data science team is able to truly shine.
March 4, 2022
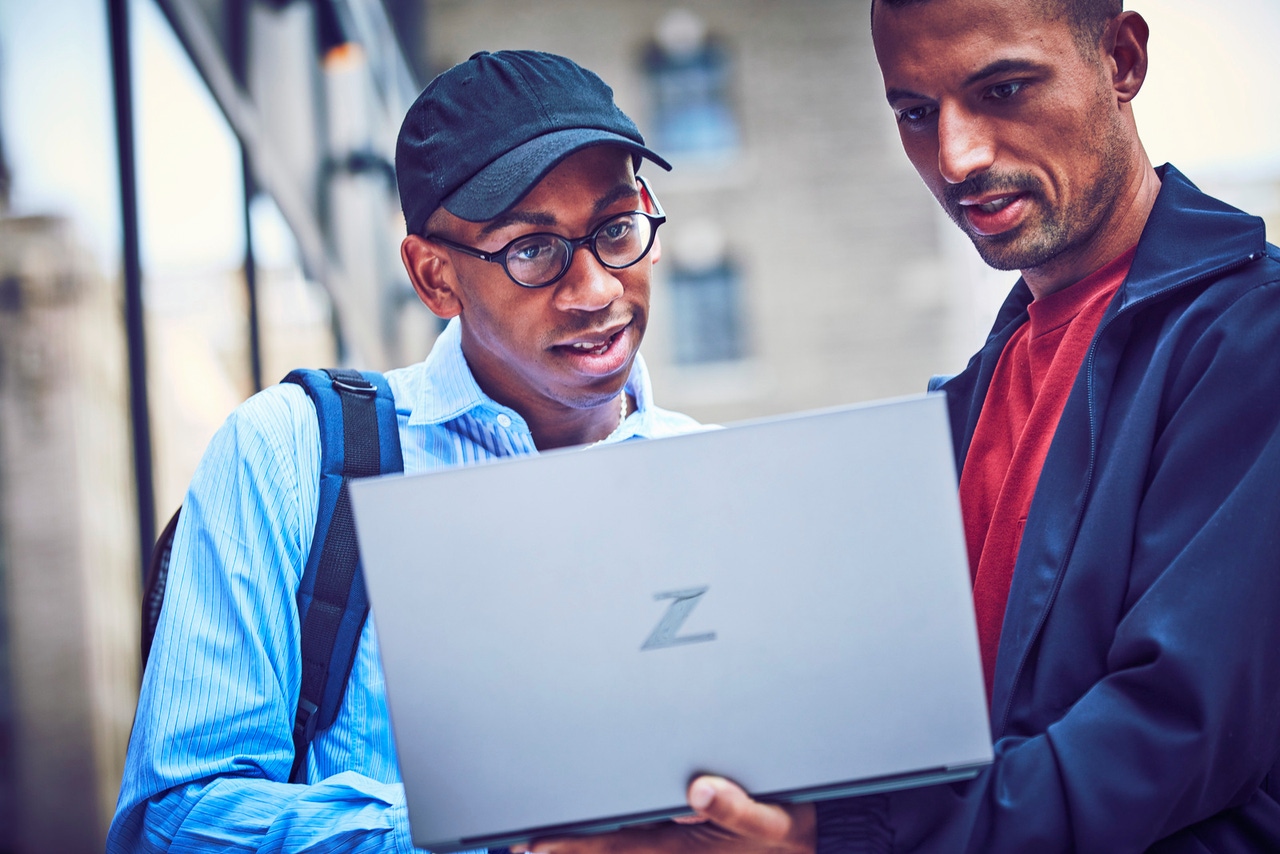
Sponsored Content
Z by HP makes products and solutions tailored to boost data science workflows. To celebrate and showcase the work of data scientists, Z recently released “Unlocked,” a 35-minute film blending Hollywood-caliber production value, interactive storytelling and data challenges. The film and companion website present data scientists with the opportunity to participate in a series of problem-solving challenges while showcasing the value of data science to non-technical stakeholders with a compelling narrative.
Whether you’re an experienced data scientist, or just getting into the industry, engage with the community by visiting www.hp.com/unlocked, and consider the following best practices for optimizing your business, operations, processes or workflow using data science.
Wouldn’t the workflow of a data scientist improve if others really knew what it takes to produce valuable insights and overall, knew what they could do to be a better collaborator? According to a study conducted by Z by HP, nearly 40% of data scientists find it difficult to explain their work to non-technical stakeholders[1].
You need the data before the scientist
The old expression, “garbage in, garbage out”, conveys that the final results can be no better than the initial input. This expression is equally relevant in data science. It’s impossible to build good models and generate actionable, relevant recommendations without investing in the underlying data infrastructure first.
Companies should build out the data infrastructure before investing in data scientists. To allow data scientists to do their best work, there are some prerequisites to keep in mind: creating data capture systems, data engineering systems, and pipelines. Without these systems in place, data scientists are unable to complete the work they were hired to do.
Stakeholders don’t need to be experts, but should understand the basics
An effective partnership goes both ways. Data scientists are consistently making sure to understand the business context of their work, so it only makes sense that leadership and other stakeholders do their best to understand the work that the data scientists are doing.
Give data scientists a seat at the table
A common theme among all data scientists is that communication with leadership is key. Inviting the leadership team to provide details about the business problem helps data scientists scope and prioritize work, as well as understand the data needs for the model. According to a survey, 40% of data scientists state that they often get started on a project prior to fully understanding the business objectives.[2] Instead, data scientists often benefit from having an open conversation with their non-technical partners: Is this a model that's going to go under production, that's going to be making decisions effectively, autonomously, with a little bit of human oversight or no oversight? Understanding the bigger picture and risk tolerance of the business is key to getting the most out of data science projects.
Data science is about probability, not prediction
As much as data science has the power to make incredibly accurate forecasts, it’s important to keep in mind that no single model is a silver bullet. Stakeholders must understand that data science is about calculating probabilities – an expectation of 100% precision is unlikely seeing as imperfections are part of every model. The idea is to train and adapt models in such a way where they improve incrementally over time – but even the best data science work is never perfect.
Every experiment is productive–even the failed ones
The nature of data science is experimental and iterative. When leadership is understanding of this and provides data science teams leeway (especially at the start of a project) it can lead to substantially better results later on.
Projects are often the story of backtracking and trying different approaches. This means there may be mistakes along the way and some misses along with the wins. In fact, there are times when a specific project might need to be abandoned because the available data and modeling methods simply aren’t yielding the desired results.
Data science isn’t software engineering
Although software engineering and data science are often conflated, they only share a few similarities: coding, creating pipelines, and getting data from one place to another. However, the similarities end here. Where other disciplines often have a finite ending to a project, data scientists devise a model and continually retrain it so that it remains relevant based on new data coming in.
Ultimately, when leadership and peers are aware of what data scientists wish others to know, it creates a more productive, successful atmosphere for all.
[1] HP proprietary research: Understanding Data Scientists, November 2021.
[2] HP proprietary research: Understanding Data Scientists, November 2021.
About the Author
You May Also Like