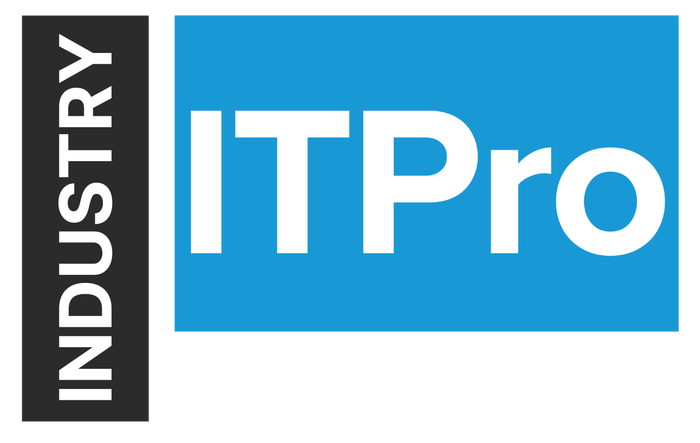
Insight and analysis on the information technology space from industry thought leaders.
GPU Costs Aren't Infinitely Scalable — What Can Be Done?
As AI/ML applications surge, companies must prioritize GPU cost monitoring and optimization strategies to prevent spiraling costs and inefficiencies.
September 13, 2024
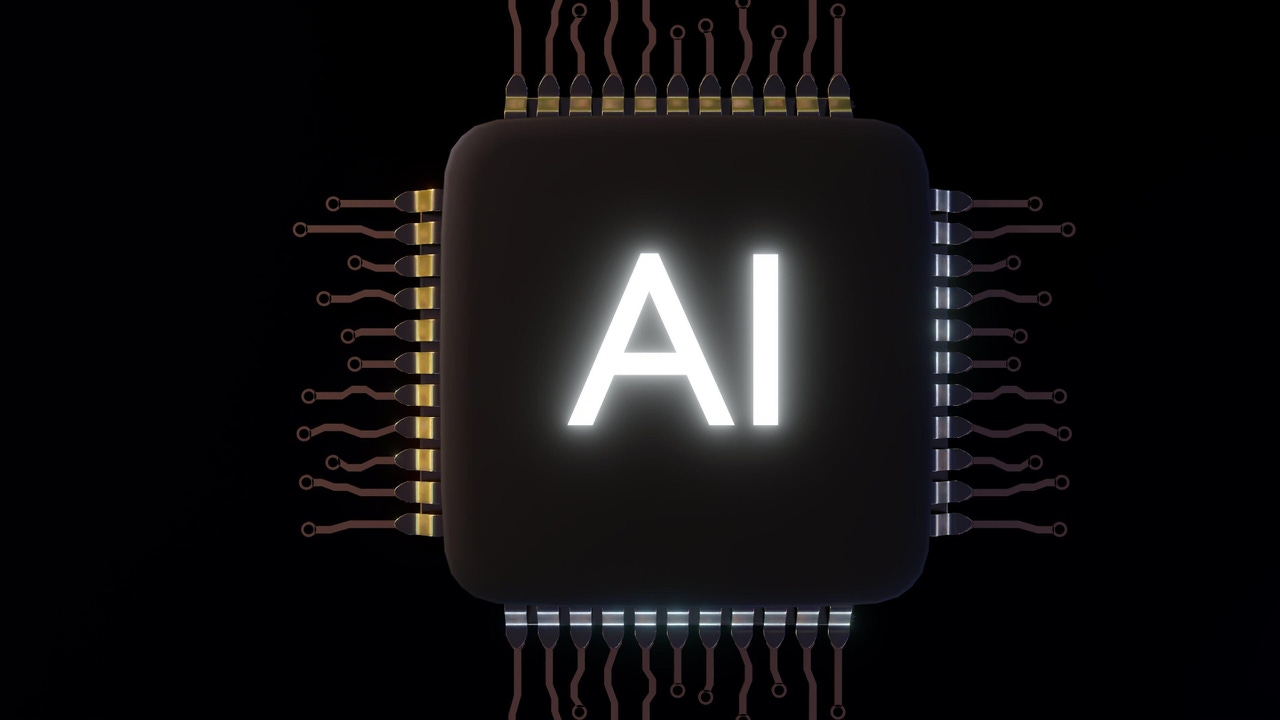
By Kai Wombacher, Kubecost
In the rush to bring AI/ML-powered applications to market and keep pace with competitive pressures, businesses have had to shell out to harness GPUs that massively accelerate AI model training and machine learning operations. The past predicts what happens next.
During the previous industry shift into cloud infrastructure, CPU resources on demand, and tooling like Kubernetes, enterprises were fine putting cost concerns on the back burner while they established a foothold with these new capabilities. That phenomenon is repeating: Until now, most businesses have gladly focused on AI/ML exploration with little thought for GPU optimization. However, that moment when costs matter approaches more quickly than organizations think. Just as businesses find it necessary to rein in their cloud and Kubernetes costs as they scale — lest their balance sheets get crushed under the weight of exponentially rising expenses — organizations will soon need to turn their attention to GPU cost controls.
The GPU Cost Visibility Challenge
Monitoring GPU usage and efficiency is massively more complex than monitoring more familiar CPU and RAM resources. While many organizations have implemented solutions to render their CPU and RAM utilization transparent and optimized, GPU utilization remains a black box for most. Many businesses have no idea what their GPU resources cost, and no idea how well they're using their GPU capacity.
The stakes of achieving GPU cost controls are also much higher because GPU expenses are huge and only going up. For instance, NVIDIA's Hopper-series GPUs carry price tags starting at $30,000. Businesses that throw these resources at their new AI initiatives and massive workloads may wonder if they're wasting six figures a month unnecessarily — but not have the capabilities to check.
The Steep AI/ML Learning Curve Can Mean Steep Costs
I recently spoke with technology leaders at a business that — like many — absolutely had to have an answer on how it was harnessing generative AI. They had jumped in headfirst, invested in a heck of a lot of GPUs, and started throwing AI models at it. It can take hours, days, or weeks to run these models, and when they were done, it was clear that they hadn't accomplished much for what they'd put in. Later, when the team had gained some experience and learned how to optimize their code, they substantially reduced their costs by consolidating GPU usage, while also training their models 10x faster.
Another GPU-centric conversation was with a business hoping to identify when a GPU completed workloads, in order to know when to send it another training job. When a GPU workload is finished, it's free for another training cycle. If it's sitting idle, the business is just eating that cost. However, until organizations gain visibility into GPU costs and optimization opportunities, gaining that efficiency is difficult.
These examples call out just how powerful transparency and insights into GPU usage can be in enabling smarter decisions as businesses steer into the AI/ML learning curve. Rather than flying blind and wasting considerable budget on poorly optimized code or idle resources, businesses can address inefficiencies and likely get to market faster thanks to that guidance.
Going Beyond Observability to Achieve Optimization
Ideally, businesses will go beyond GPU cost monitoring and observability to enable detailed optimization insights. Certainly, teams should have the means to delve into spending on each specific GPU and understand the efficiency of each utilization. But that's just the start.
For example, insights might identify opportunities to replace a certain GPU with a smaller GPU or a different model — handling the same workloads at a lower cost. Insights might also flag workloads that can be paired together to interoperate and share a GPU effectively, allowing teams to consolidate usage. Teams backed by clear insights can also introduce new efficiencies with confidence, and without impacting training or application performance.
Sharing Is Saving
As alluded to in the last example, GPU sharing is a strong cost-saving opportunity. However, few teams have robust GPU sharing in place as of yet. From an infrastructure perspective, GPU utilization is one container to one GPU node. A single $30,000 GPU node is used for a single container.
While AI workloads can and do saturate a full GPU, the issue is that teams don't have the visibility and insights to know how efficiently they're using a GPU, or when they could be more efficient. Usage of hugely expensive GPUs might be 100%, or might be 1%. As the market matures, GPU sharing will certainly be an optimization goal many businesses pursue.
The Carbon Impact
We've all heard the widely shared carbon statistics, such as that creating 1,000 generative AI images is similar to driving a gas-powered car 4.1 miles. GPUs use a lot of power: Those NVIDIA Hopper GPUs are 1-kilowatt cards.
In introducing GPU cost monitoring and new efficiency, many businesses will also like to see the carbon impact of their GPUs, and optimize their efficiency in this area as well.
Rein in GPU Costs Sooner Than Later
Businesses are wise to implement GPU cost monitoring and optimization strategies as soon as they can — and before they encounter exponential cost increases or issues that cause them to fall behind in the market. Introducing efficient and sustainable GPU costs today enables scaling and growth tomorrow, setting the stage for AI/ML initiatives to achieve lasting success.
About the author:
Kai Wombacher is a Product Manager at Kubecost.
About the Author
You May Also Like