Data Profiling to the Rescue by Pushing Metadata Boundaries
Don’t let bad data sneak up on you when and where you least expect it. Ferret out bad data with Melissa Data’s newest Profiling Component for SSIS. Learn how to take control of your data using knowledge-base-driven metadata. The truth shall set you free!
February 25, 2015
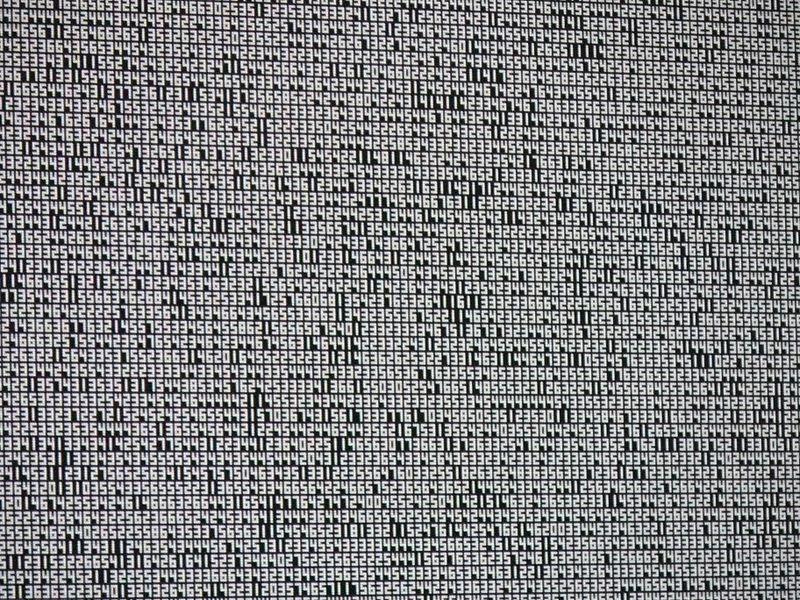
Two truths about data: Data is always changing, and data will always have problems. The two truths become one reality: bad data. Elusive by nature, bad data manifests itself in ways we wouldn’t consider and conceals itself where we least expect it. Compromised data integrity can be saved with a comprehensive understanding of the structure and contents of data. Enter data profiling.
Throw off the mantle of complacency and take an aggressive approach to data quality, leaving no opening for data contamination. How? Profiling.
More truths: Profiling is knowledge. Knowledge is understanding. That understanding extends to discovering what the problems are and what needs to be done to fix it.
Armed with Metadata
Metadata is data about your data. The analysis of gathered metadata with profiling exposes all the possible issues to its structure and contents, giving you the information--knowledge and understanding--needed to implement data quality regimens.
Here are only a few of the main types of generic profiling metadata and the purpose of each:
1. Column Structure: Maximum/Minimum Lengths and Inferred Data Type
These types of metadata provide information on proper table formatting for a target database. It is considered problematic, for example, when an incoming table has values which exceed the maximum allowed length.
2. Missing Information: NULLs and Blanks
Missing data can be synonymous with bad data. This applies, for example, to where an Address Line is Blank or Null, which in most cases is considered a required element.
3. Duplication: Unique and Distinct Counts
This allows for the indication of duplicate records. De-duplication is a standard practice in data quality and is commonly considered problematic. Ideally, there should only be a single golden record representation for each entity in the data.
Other equally important types of generic profiling metadata include Statistics for trends data; Patterns (ReqEx), which allow for identifying deviations from formatting rules; Ranges (Date, Time, String and Numbers); Spaces (Leading/Training Spaces and Max Spaces between Words); Casing and Character Sets (Upper/Lower Casing and Foreign, Alpha Numeric, Non UTF-8) Frequencies for an overview of the distribution of records for report generation on demographics and more.
Metadata Revolution & New Face of Profiling
Right now the most powerful profiling tool for gathering Metadata is the Melissa Data Profiler Component for SSIS. This system is used at the data flow level, allowing you to profile any data type that SSIS can connect with (unlike the stock Microsoft Profiling Component, which is only for SQL Server databases).
More importantly, the Melissa Data Profiler offers more than 100 types of metadata, including all the generic profiling Metadata mentioned here.
The innovative Melissa Data’s Profiler Component gathers data-driven metadata, which goes beyond the standard set of profiling categories. By combining our extensive knowledge on contact data, this allows us to get information that is not simply based on rules, norms and proper formatting. Rather, it provides metadata with the aid of a back-end knowledge base. We can gather unique types of metadata--such as postal code, state and postal code mismatch, invalid country, email metadata, phone and names.
Take Control
The secret to possessing good data goes back to a simple truth: understanding and knowledge of your data through profiling. The release of Melissa Data’s Profiler for SSIS allows you to take control of your data through use of knowledge base-driven metadata. The truth shall set you free!
A Data Quality Analyst at Melissa Data, Joseph Vertido is an expert in the field of data quality. He has worked with numerous clients in understanding their business needs for data quality, analyzing their architecture and environment, and recommending strategic solutions for how to successfully integrate data quality within their infrastructure. He has also written several articles for implementing data quality solutions and techniques. Joseph holds a degree in Computer Science from the University of California, Irvine.
About the Author
You May Also Like