Maturing Your Cloud-Based Data Lake Architecture
There are many challenges that must be considered if you want your organization’s data lake architecture to be a valuable asset instead of a “data dumping ground.”
October 31, 2019
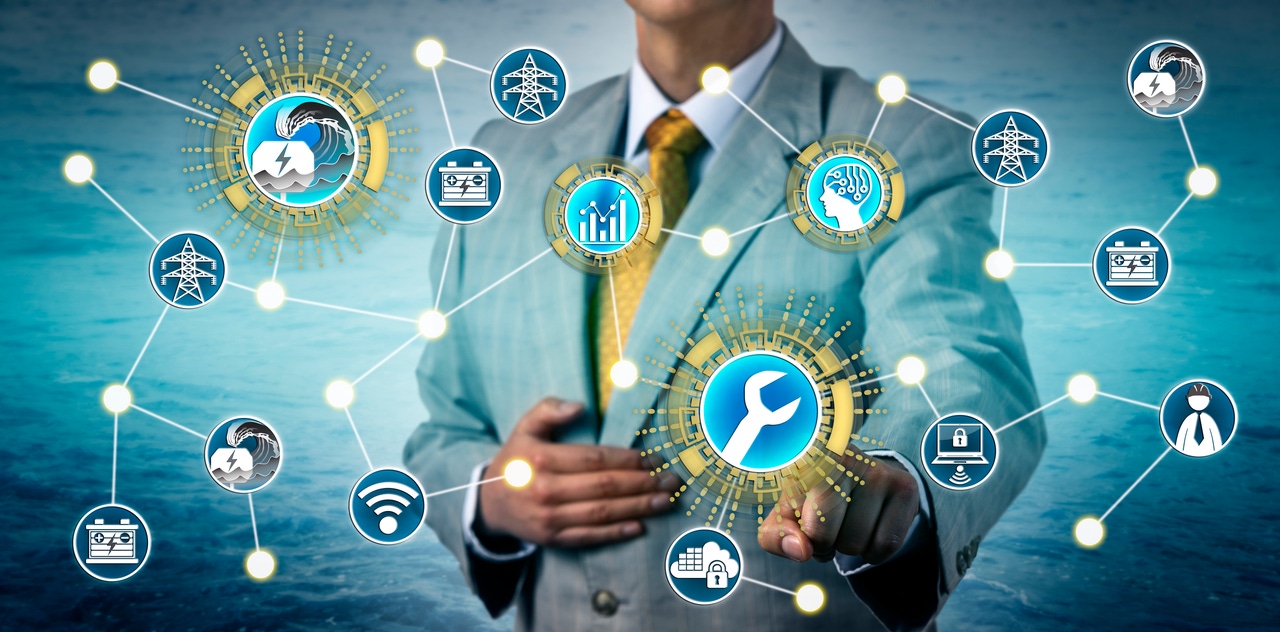
Early on in the development and evolution of the Apache Hadoop ecosystem, system developers recognized that the scalability of the distributed file system (namely the Hadoop Distributed File System, or HDFS) provided a reasonable repository for file storage, management, and ultimately, sharing and use. The concept of the data lake (which emerged around 2010) is defined by Gartner as "a collection of storage instances of various data assets. These assets are stored in a near-exact, or even exact, copy of the source format and are in addition to the originating data stores."
Early-generation data lakes relied on HDFS, often using commodity hardware in an on-premises environment, as an architectural framework to support scalable storage of a growing repository of shared data assets. Yet despite the reliance on open source software using commodity parts, these on-premise HDFS data lakes still require significant resource investment in terms of platform architecture and design, the cost of acquired hardware, development costs, as well as ongoing costs for space/power/cooling and ongoing maintenance and management.
At the same time, cloud vendors have launched object storage services that are equally amenable to scalable data management yet alleviate many of these resource demands. Instead of acquiring and managing hardware, organizations pay for the cloud storage space (and associated computing resources for data preparation and data delivery) they use. These cost benefits have inspired a number of organizations to begin migrating their data lakes to the cloud.
Conceptually, data lakes are intended to improve data sharing and data reuse. Yet, whether the data lake is deployed on-premise or in the cloud, there are a number of challenges that must be considered if you want your organization’s data lake to be a valuable asset instead of just a “data dumping ground,” including;
Data sprawl: The availability of an unbounded scalable data object repository effectively begs data owners to push their data assets into the data lake. Data sprawl refers to a growing amount and variety of data assets to be managed within the data lake. The concern is that without any type of governance and control, it is difficult to distinguish what information exists within the data assets, making them increasingly unusable.
Data awareness: Unorganized data lakes provide little or no guidance for coordination and administration of shared data assets. The result is that it is difficult to find the data assets that contain the information that data analysts and data scientists want. Even if they could find the data sets, there might not be any methods or services for gaining access.
Data sharing: The data lake is intended to be a platform for publishing data to be shared among the different data consumer communities (data analysts, data scientists, business analysts, etc.). But when it is difficult to know what data assets are in the data lake, there is a risk that different parties will extract data from the same sources and replicate the data sets that are moved into the data lake. Aside from wasting space, this also poses issues for data consistency and trustworthiness.
Data sensitivity: Data assets made available in a data lake are more “open” than closely governed data extracts and exchanges, raising the concern that private, personal or other types of sensitive data may be exposed to unauthorized parties.
A mature data lake must be outfitted with the right practices and tools that help address these concerns.
For example, consider data curation, which is a process of assembling, organizing, managing and ensuring the usability of a collection of data assets for the purpose of expanding data accessibility and sharing among a community of data producers and consumers. Data curation relies on data asset surveillance and classification to distinguish the types of data an asset contains (for example, structured vs. semi-structured), object metadata (such as the data set’s owner, when it was produced and where it is located in the data lake’s hierarchy), data lineage, access methods and services, and whether the data asset contains sensitive information and what types of sensitive information.
This collected information can be maintained within a data catalog that is visible to the members of the different data consumer communities and enables searchability and recommendations to data consumers as to the best data assets that meet their needs. Finally, organizations can adopt data protection methodologies to prevent unauthorized data exposure.
While each of these methods and technologies is necessary, ensuring that they are properly integrated and controlled requires data governance. Data governance provides a framework for defining and implementing compliance with information management policies. Combining governance with the right tools and techniques can radically transform a “data dump” into an accessible and exploitable enterprise information repository.
About the Author
You May Also Like