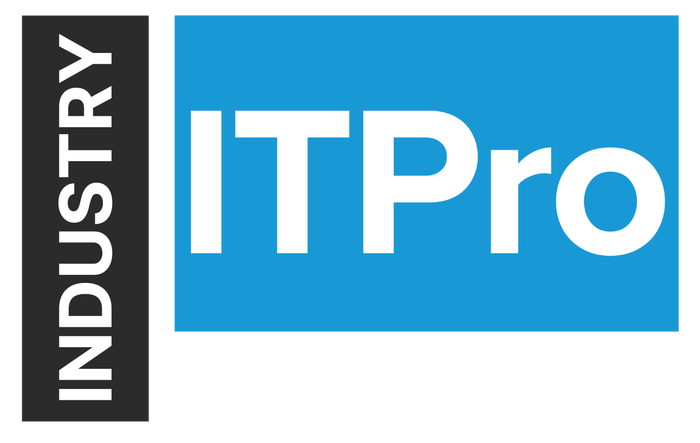
Insight and analysis on the information technology space from industry thought leaders.
Here's Why Your Data Science Project Failed (and How to Succeed Next Time)Here's Why Your Data Science Project Failed (and How to Succeed Next Time)
Here's a list of primary reasons why data science projects fail — and strategies for forging success in the future.
January 13, 2023
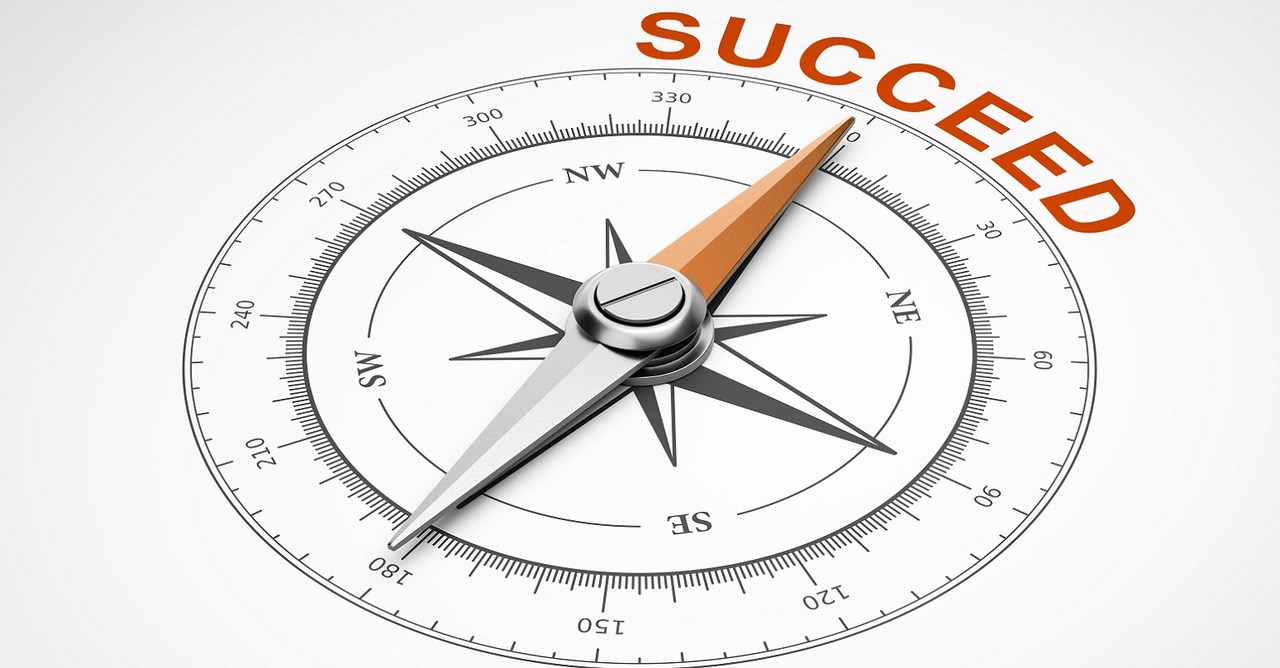
Eighty-seven percent of data science projects never make it beyond the initial vision into any stage of production. Even some that pass through discovery, deployment, implementation, and general adoption fail to yield the intended outcomes. After investing all that time and money into a data science project, it's not uncommon to feel a little crushed when you realize the windfall results you expected are not coming.
Yet even though there are hurdles to implementing data science projects, the ROI is unparalleled — when it's done right. For example, Coca-Cola has used data from social media to identify its products or competitors' products in images, increasing the depth of consumer demographics and hyper-targeting them with well-timed ads. GE has used artificial intelligence to cut product design times in half. Data scientists have trained algorithms to evaluate millions of design variations, narrowing down potential options within 15 minutes.
With all of that potential, don't let your first failed attempt turn you off to the entire practice of data science. Here's a list of primary reasons why data science projects fail — and a few strategies for forging success in the future — to help you avoid similar mistakes.
You Lack Analytical Maturity
Many organizations are antsy to predict events or decipher buyer motivations without having first developed the proper structure, data quality, and data-driven culture. And that overzealousness is a recipe for disaster. While a successful data science project will take some time, a well-thought-out data science strategy can ensure you will see value along the way to your end goal.
Effective analytics only happens through analytical maturity. That's why organizations should conduct a thorough current state analysis before they embark on any data science project. In addition to evaluating the state of their data ecosystem, they can determine where their analytics falls along the following spectrum:
Descriptive Analytics: This type of analytics is concerned with what happened in the past. It mainly depends on reporting and is often limited to a single or narrow source of data. It's the ground floor of potential analysis.
Diagnostic Analytics: Organizations at this stage are able to determine why something happened. This level of analytics delves into the early phases of data science but lacks the insight to make predictions or offer actionable insight.
Predictive Analytics: At this level, organizations are finally able to determine what could happen in the future. By using statistical models and forecasting techniques, they can begin to look beyond the present into the future. Data science projects can get you into this territory.
Prescriptive Analytics: This is the ultimate goal of data science. When organizations reach this stage, they can determine what they should do based on historical data, forecasts, and the projections of simulation algorithms.
Your Project Doesn't Align With Your Goals
Data science, removed from your business objectives, always falls short of expectations. Yet in spite of that reality, many organizations attempt to harness machine learning, predictive analytics, or any other data science capability without a clear goal in mind. In my experience, this happens for one of two reasons:
1. Stakeholders want the promised results of data science but don't understand how to customize the technologies to their goals. This leads them to pursue a data-driven framework that's prevailed for other organizations while ignoring their own unique context.
2. Internal data scientists geek out over theoretical potential and explore capabilities that are stunning but fail to offer practical value to the organization.
Outside of research institutes or skunkworks programs, exploratory or extravagant data science projects have a limited immediate ROI for your organization. In fact, the odds are very low that they'll pay off. It's only through a clear vision and practical use cases that these projects are able to garner actionable insights into products, services, consumers, or larger market conditions.
Every data science project needs to start with an evaluation of your primary goals. What opportunities are there to improve your core competency? Are there any specific questions you have about your products, services, customers, or operations? And is there a small and easy proof of concept you can launch to gain traction and master the technology?
The above use case from GE is a prime example of having a clear goal in mind. The multinational company was in the middle of restructuring, re-emphasizing its focus on aero engines and power equipment. With the goal of reducing their six- to 12-month design process, they decided to pursue a machine learning project capable of increasing the efficiency of product design within their core verticals. As a result, this project promises to decrease design time and budget allocated for R&D.
Organizations that embody GE's strategy will face fewer false starts with their data science projects. For those that are still unsure about how to adapt data-driven thinking to their business, an outsourced partner can simplify the selection process and optimize your outcomes.
Your Solution Isn't User-Friendly
The user experience is often an overlooked aspect of viable data science projects. Organizations do all the right things to create an analytics powerhouse customized to solve a key business problem, but if the end users can't figure out how to use the tool, the ROI will always be weak. Frustrated users will either continue to rely upon other platforms that provided them with limited but comprehensible reporting capabilities, or they will stumble through the tool without unlocking its full potential.
Your organization can avoid this outcome by involving a range of end users in the early stages of project development. This means interviewing both average users and extreme users. What are their day-to-day needs? What data are they already using? What insight do they want but currently can't obtain?
An equally important task is to determine your target user's data literacy. The average user doesn't have the ability to derive complete insights from the represented data. They need visualizations that present a clear-cut course of action. If the data scientists are only thinking about how to analyze complex webs of disparate data sources and not whether end users will be able to decipher the final results, the project is bound to struggle.
You Don't Have Data Scientists Who Know Your Industry
Even if your organization has taken all of the above considerations into mind, there's still a chance you'll be dissatisfied with the end results. Most often, it's because you aren't working with a data science consulting firm that comprehends the challenges, trends, and primary objectives of your industry. The right partner will have a full grasp of the data sources, industry use cases, and what target end users will need. They can address your pain points and already know how to extract full value for your organization.
Ryan Lewis is the managing consultant for Data Insights at 2nd Watch.
About the Author
You May Also Like