Testing for AI Bias: What Enterprises Need to Know
The meteoric growth of artificial intelligence is increasing concerns about ethics, leading to tools and procedures to ensure responsible development.
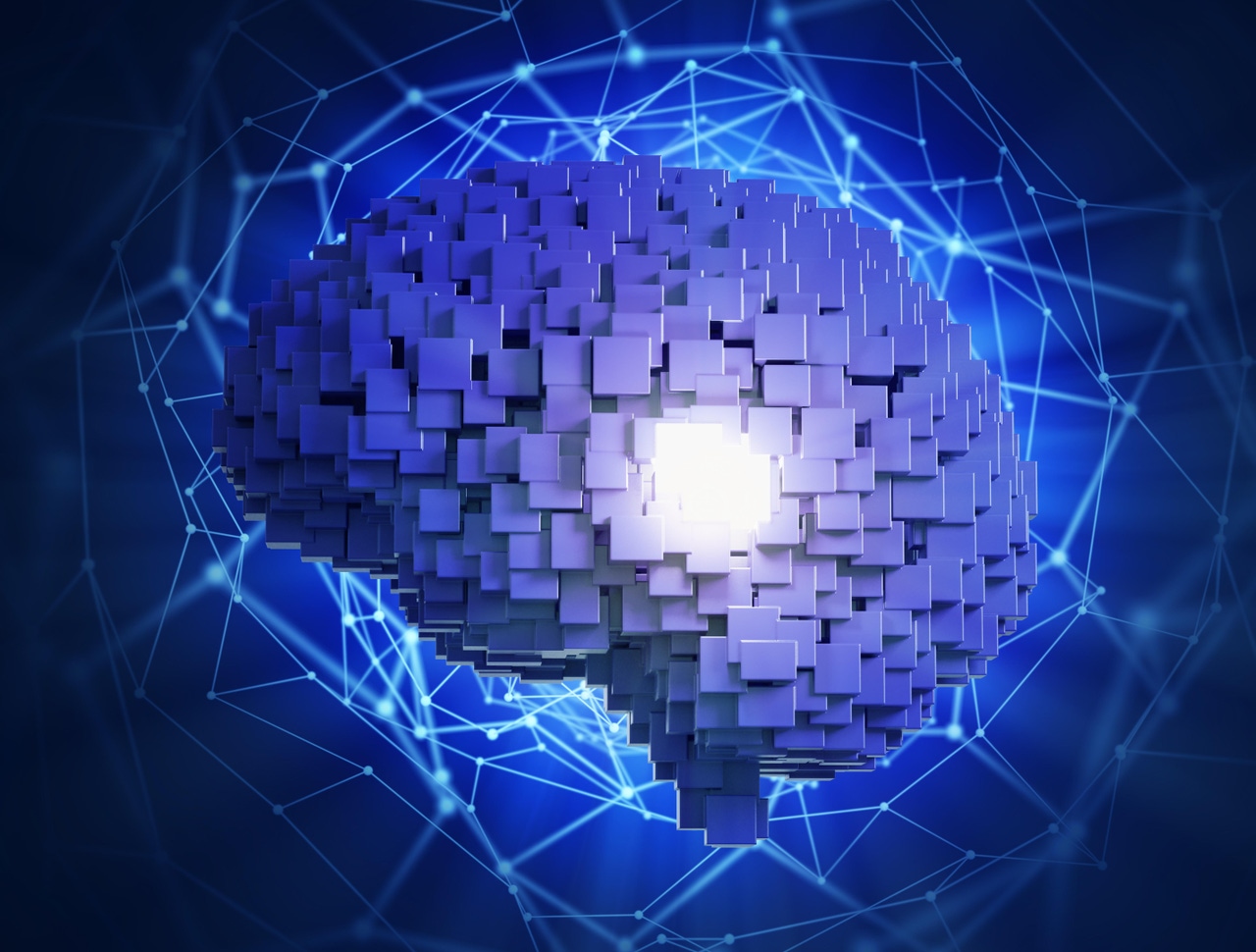
As the adoption of artificial intelligence increases, so does the call for more explainable systems that are free of bias. To pass the sniff test for AI ethics, enterprises are adopting frameworks and using software to ensure that the datasets used in AI models – and the results they generate – are free from bias.
The growing complexity of AI models and their integration with enterprise architecture are creating many failure points, said Balakrishna DR, Infosys head of AI and automation. New “AI assurance” platforms are gaining traction to cater to this critical challenge. These platforms come with specialized procedures and automation frameworks to provide model assurance, test data needed for model testing, and evaluate performance and security.
3 Things To Know About AI Ethics Testing
Here are a few aspects of testing for ethics and bias to consider.
1. Testing for AI bias is a complicated can of worms
While quality control testing for software is pretty routine, especially because testers know what to look for, AI bias testing is not so straightforward.
“Testing for AI bias is subjective and depends on the context and domain characteristics,” DR said. “Bias can be introduced by datasets, algorithms, or the data scientists themselves. This means that testing has to be domain-specific and reflective of various scenarios and paths.”
A common misconception is that responsible AI “entails adopting a single piece of code or adopting a single standard,” noted Steven Karan, vice president and head of insights and data for Capgemini Canada. “In actuality, responsible AI is a framework or set of guidelines that if holistically adopted greatly minimizes the risk of bias in AI-based solutions.”
2. You must keep an eye out for pitfalls
AI can be designed by data engineers and scientists to process data in a manner that affirms pre-existing beliefs. “For example, a model builder that builds a resume-screening tool for a bank may believe that the best fund managers may only come from four schools in the greater Boston area," Karan said. "As a result, the model builder could unconsciously build an algorithm that reinforces this belief."
Another pitfall to look for: internal bias. “It is difficult to go against the grain and look for edge scenarios or minority datasets,” DR explained. “Testing should be independent of the analysis and results carried out by the data science teams.”
3. A variety of tools are available
Organizations typically follow a framework for software development that functions as a guardrail so the results can be unbiased. A data protection impact assessment, or DPIA, allows organizations to assess and minimize the project risks involved with working with specific types of data.
Tools such as the open source What-If Tool aim to help organizations detect bias in machine learning models. “There needs to be tools to audit the output of the AI," said Goh Ser Yoong, CIO of Jewel Paymentech, a financial risk technology company. He pointed to the IBM’s open source toolkit AI Fairness 360, which can be useful for the engineering team to test against a set of AI ethics principles.
Other ethics software testing tools include Bias Analyzer from PwC, Trusted AI from DataRobot, and IBM’s Watson OpenScale.
Organizations should choose tools based on the class of the algorithm and the development stage, DR added.
Thorough Testing and Adjustments
To be the most effective in detecting bias, testing should be performed across the lifecycle of AI development and implementation: pre-training, model validation, model selection, finetuning, performance, and security validation and integration checks.
“It is key to remember that there is no one magic potion to making AI more responsible,” Karan said. “Rather, think of the problem as requiring changes to processes, governance, and even culture.”
About the Author
You May Also Like