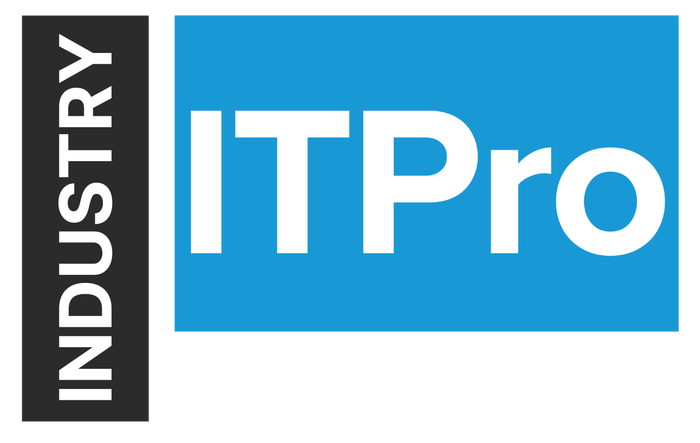
Insight and analysis on the information technology space from industry thought leaders.
The Rise of Generative AI Fuels Focus on Data QualityThe Rise of Generative AI Fuels Focus on Data Quality
As GenAI adoption grows, enterprises face challenges with fragmented, poor-quality data, necessitating new approaches to ensure accurate, compliant, and reliable AI applications.
September 23, 2024
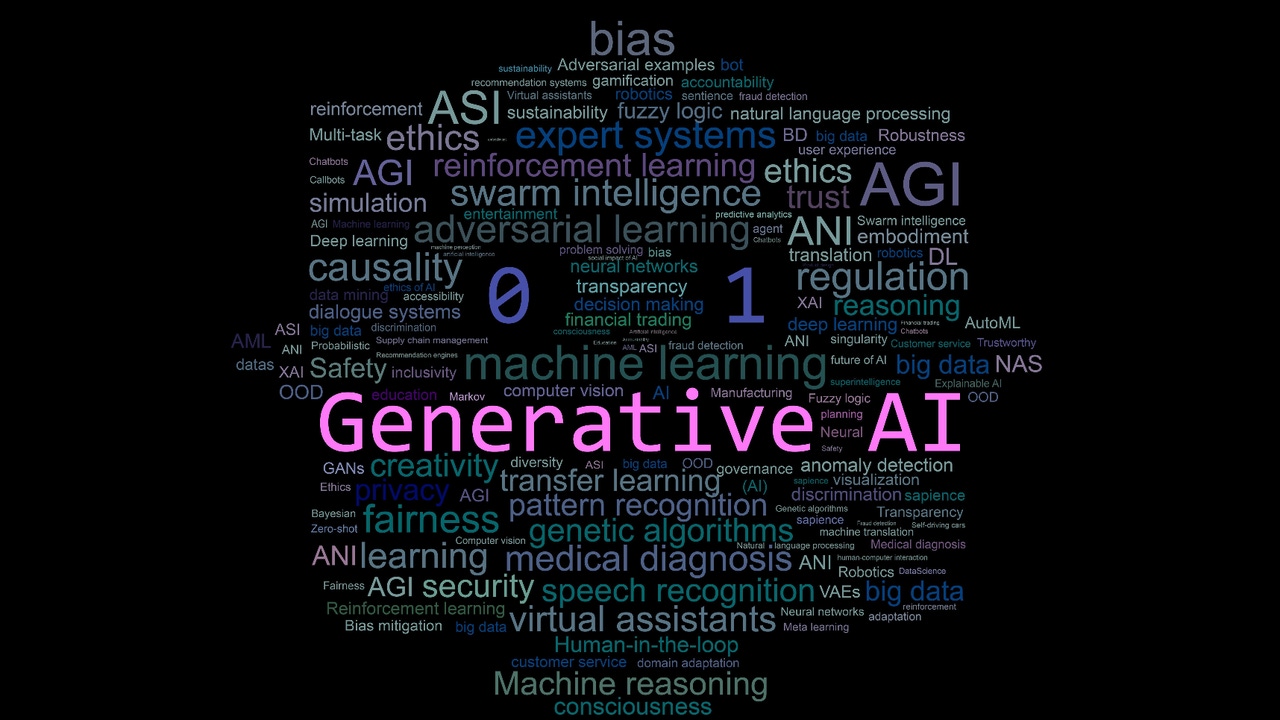
By Yuval Perlov, K2view
Generative artificial intelligence (GenAI) applications are taking center stage, and with them comes a heightened focus on data quality. This isn't surprising. Gartner predicts that 30% of GenAI projects will be abandoned after the proof-of-concept stage by 2025. Why? The root issue lies in the poor data quality, large data gaps, and the fact that enterprise data is not ready for GenAI consumption.
Grounding large language models (LLMs) with reliable internal data and knowledge is essential for reducing hallucinations and improving accuracy, relevancy, and personalization of the LLM. AI and data teams recognize the critical role data plays in building trust for GenAI applications within businesses. However, achieving this ideal requires complete, compliant, and contextual data, presenting significant data quality challenges.
So, what makes data quality such a challenge? Here are the main reasons.
Fragmented Data: The Nemesis of GenAI Applications
Enterprise data is often siloed in dozens of systems. Customer data, for example, is typically fragmented across CRM, billing, customer service, interaction management, call recordings, and the list goes on. This fragmentation makes it incredibly difficult to serve a real-time and reliable customer view to the underlying LLMs powering customer-facing GenAI apps.
To overcome this challenge, organizations need a robust data infrastructure capable of real-time data integration and unification, master data management, data transformation, anonymization, and validation. The more fragmented the data, the steeper the climb toward achieving high-quality data for GenAI applications.
Lost in Translation: The Challenge of Poor-Quality Metadata for GenAI
Imagine a brilliant translator struggling with instructions in a cryptic language. That's essentially what happens when GenAI apps encounter data with sparse metadata. Metadata, the data that describes data, acts as a crucial bridge between an organization's information and the LLMs powering the GenAI apps. Rich metadata provides the context and understanding LLMs need to effectively utilize data for accurate and personalized responses.
Unfortunately, many organizations face the challenge of maintaining stale data catalogs. The dynamic nature of today's data landscape makes it difficult to keep metadata current. The need to constantly track and update information in a dynamic, decentralized, and diverse environment makes this process more complex. This results in a communication gap between the data and the LLMs, ultimately hindering the quality and effectiveness of your GenAI applications.
Data Privacy vs. GenAI Insights: A Balancing Act with Data Quality
Data privacy regulations are a necessary safeguard for sensitive information, but they can damage data quality. While anonymization and access controls are crucial for compliance, these measures can introduce a significant challenge: maintaining referential consistency of the data. Referential consistency refers to the accuracy of relationships between different data points. When anonymization techniques like static or dynamic masking disrupt these relationships, the data quality suffers. The masked data becomes less reliable and meaningful for users and LLMs. Essentially, the very measures designed to protect data privacy can inadvertently undermine the quality of the data itself, hindering the ability of GenAI to extract valuable insights. It's incredibly difficult to ensure that both data quality and privacy compliance are achieved, but it can be done by applying data quality metrics and consistent data compliance audits.
Data Quality in Isolation
Traditionally, data quality initiatives have often been isolated efforts, disconnected from core business goals and strategic initiatives. Some data quality initiatives are compliance-focused, data cleaning, or departmental efforts — all are very important but not directly tied to larger business goals. This makes it difficult to quantify the impact of data quality improvements and secure the necessary investment. As a result, data quality struggles to gain the crucial attention it deserves.
However, the rise of GenAI presents a game-changer for enterprises. GenAI apps rely heavily on high-quality data to generate accurate and reliable results. Customer-facing GenAI applications, like chatbots, can potentially expose data quality issues to the world. If GenAI is a strategic business initiative, fixing data quality issues needs to be a top priority. For enterprises to showcase the ROI on AI investments and reap the benefits, they need to fix data quality issues from pilot to production. It is also necessary to allocate resources for continuous improvement.
What Organizations Should Do About These Lingering Challenges
Traditional approaches to data quality involve batch ingestion of multi-source enterprise data into centralized data lakes, which enforce the necessary data quality and privacy controls. These approaches often fall short when it comes to GenAI because they are compute-intensive and offline, and because expensive cleansing processes and ever-evolving privacy measures don't quite do the job.
Organizations need a new way to organize the data and make it GenAI-ready, making sure it is continuously synced with the source systems, continuously cleansed according to a company's data quality policies, and continuously protected. But the solution extends beyond technology.
Organizations must prioritize data quality by establishing key performance indicators (KPIs) directly linked to GenAI success, such as customer satisfaction, resolution rate, and response time. Building multi-disciplinary GenAI teams that include data quality engineers fosters collaboration and ensures all aspects, from data preparation to application performance, are aligned.
About the author:
Yuval Perlov, CTO of K2view, an AI-driven data management software provider.
About the Author
You May Also Like