Morgan Stanley Robot Learns by Reading Unreadable Muni Documents
Morgan Stanley, one of Wall Street’s biggest investment banks, experimented with farming out the job of reading 120,000-word bond prospectuses to robots, seeing if the results could yield a sort of CliffsNotes that may separate the signal from the noise.
September 16, 2019
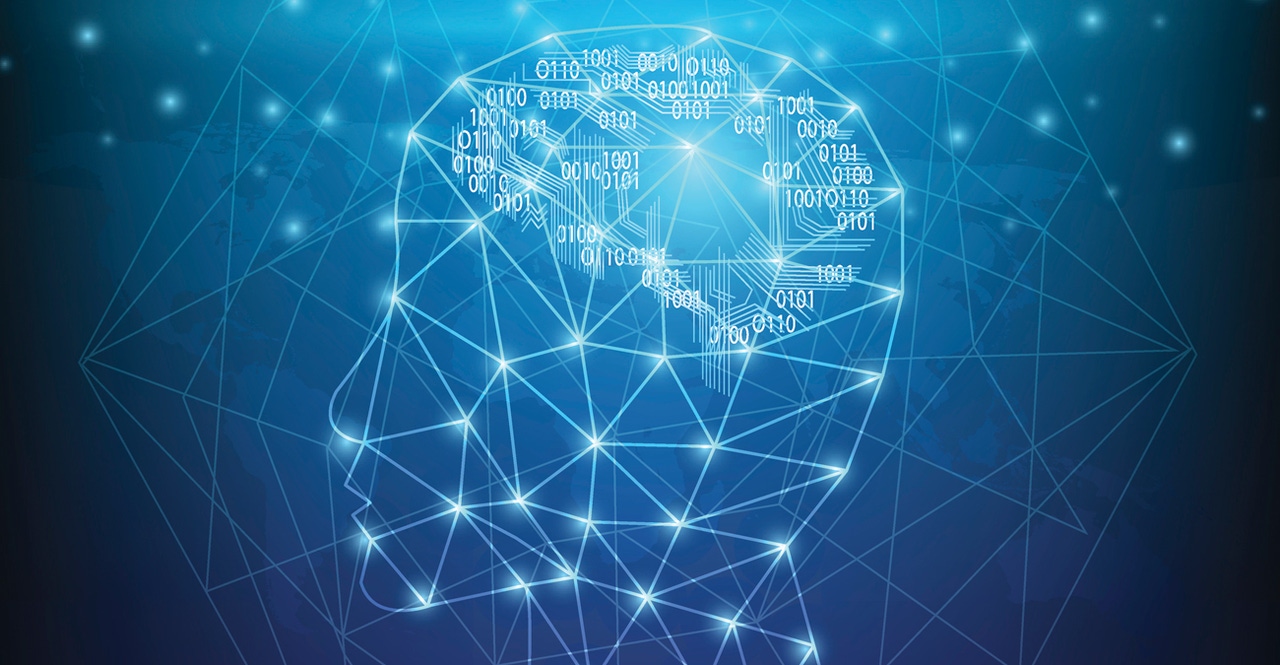
(Bloomberg) -- They’re longer than classics like Henry David Thoreau’s “Walden” and modern hits like J.K. Rowling’s “Harry Potter and the Prisoner of Azkaban” but nowhere nearly as engaging.
Yet each week, American state and local governments crank out the doorstops by the dozens, creating a dismal stack of soporific homework for money managers studying whether or not to buy their bonds.
So Morgan Stanley, one of Wall Street’s biggest investment banks, experimented with farming out the job of reading 120,000-word bond prospectuses to robots, seeing if the results could yield a sort of CliffsNotes that may separate the signal from the noise.
Strategists Michael Zezas and Mark Schmidt ran 150 official statements through a machine-learning program. They said it revealed some patterns that could help investors avoid credit-rating downgrades or defaults without reading through hundreds of pages of reports.
They focused on bonds issued by local agencies that are backed by riskier projects like continuing-care retirement centers, hospitals and speculative real estate developments. That’s where doing close research is most important because local governments almost never default on their own bonds. Here’s some lessons:
More words, better odds: Official statements for continuing-care retirement centers that didn’t default averaged 20,194 words longer than those that did, they found. The tendency also held true for so-called dirt bonds sold for real estate projects.
Executive bios: Speculative developments tend to rely on the word “Mr.” to highlight the management of the project, since the riskier deals need to play up their executives’ skills as a key selling point.
Boring is better: Higher-quality debt tended to have more references to the financial statement than defaulted or downgraded debt. The more “boring” the documents, the better, the strategists said.
It was Morgan Stanley’s first time using natural language processing on municipal-bond issuers’ official statements, Zezas said in an email. The bank reported the results to clients to show how Morgan Stanley takes a quantitative approach to its research.
He said they used relatively new techniques and principles outlined by a Stanford University professor, who experimented with it as a way to sift through the huge amounts of information involved in modern political affairs.
Zezas said the bank plans to further test its conclusions. Their next step is to gather more official statements, get more data and solicit feedback from clients. The bank said the findings could help analysts when they are asked to provide a quick take on a new bond deal, not serve as their computerized replacements.
“We don’t recommend cursory credit analysis,” Zezas and Schmidt said in their report to clients. “However, sometimes a simple rule-of-thumb can help.”
About the Author
You May Also Like