How to Set Machine Learning and Artificial Intelligence ExpectationsHow to Set Machine Learning and Artificial Intelligence Expectations
Companies must set reasonable machine learning and artificial intelligence expectations to fully exploit the technologies.
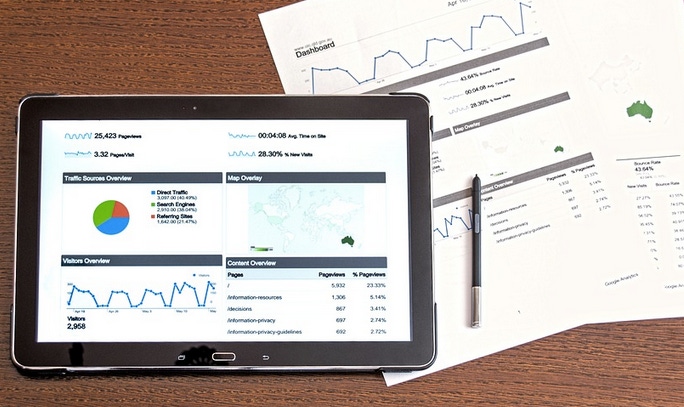
Machine learning and artificial intelligence have been hailed as transformative for a wide variety of industries, and, in the future, they may be. It is true that ML and AI are affecting some enterprise operations in important and significant ways. However, it’s also the case that, in many organizations, ML and AI are not so much changing everything quickly as changing some things, very incrementally. To move forward with the technology, it's important for companies to thoughtfully set machine learning and artificial intelligence expectations.
“AI is transforming business operations by automating workflows and functioning as infrastructure,” said Derek Wang, doctor of computer science and CEO/founder of Stratifyd. “Taking on this role means it handles the mundane tasks humans have traditionally done, such as reading and categorizing data.”
Sheldon Fernandez, CEO of DarwinAI, has a different take: “While AI has accomplished some dazzling things in an academic or research capacity, businesses are still figuring out how to translate its predictive capabilities into more mundane but practical use cases for the enterprise.”
Given that AI has the potential to change business operations by automating workflows and functioning as infrastructure, it’s important to consider the barriers in the way of those changes. Specifically, even for organizations where there is a strong use case for the deployment of AI/ML-based technologies, a lack of knowledge, infrastructure and/or data can hamper implementation.
Lack of Understanding
One barrier to AI adoption in the enterprise is simply a lack of understanding of how related technologies can be put to use for an individual operation. “Understanding the quantum of business benefits of artificial intelligence still poses a significant learning curve for most CIOs,” said Sayid Shabeer, CPO of HighRadius.
Finding the right AI talent and finding use cases for AI applications are challenges, Shabeer said, but, more significantly, many organizations are unsure of how AI can work to make them more agile, to save costs or to drive digital transformation.
One potential solution for this is to isolate the functions or business processes requiring human intervention, and targeting those with the best ROI, Shabeer said.
“We have seen finance and accounting as ... functions that consistently top operations that robotics and artificial intelligence can impact substantially, so much so that [the technologies'] very adoption is defining many as leaders,” he said.y
Hardware Costs
Deploying AI-based solutions at the enterprise level also can be be expensive, in part because it requires specific hardware to do that properly.
“Neural networks and algorithms use GPU servers mainly,” said Alexey Chernyshev, CEO at Odus.ai. “Such equipment costs tens of thousands of dollars.”
Tom Leyden, vice president of corporate marketing at Excelero, agreed: “AI is still in its very early days and requires very substantial hardware investments,” he said.
Those investments aren’t guaranteed to go the distance, either. Things change quickly and hardware can become essentially obsolete in just a couple of years, Leyden said. The result is that smaller enterprises are cautious about deploying AI until they know they can scale the technology efficiently, quickly and affordably, he added.
One option for smaller firms is AI as a service. “New AI as a service models make a lot of sense for smaller enterprises, and, based on our work with a few of them already for scale-out storage, we envision strong growth for AI as a service in short to medium term,” said Leyden.
Data Needs
Larger organizations typically have more money to spend on the hardware required to run ML and AI, but they also arguably have a greater need for the technologies.
“It’s easy to see why large enterprises are the first to implement this tech--they simply have much more data to sort through, and leaving that task up to a handful of analysts would take too much time,” Wang said. “Smaller companies can keep up with the trickle of customer feedback, but larger corporations would be pulled under the current.”
Traditional data analytics have been IT-led, he said: Data is received, parameters are set, the pipeline is built, and results are tested … a year or more later. When that process stays in place, the deployment and scaling of AI is going to be incremental.
“Businesses don’t have the tolerance for this kind of delay anymore, and they need a solution that offers true self service to a wider array of employees,” Wang said. Those solutions can be AI-driven, he said, when the right investments are made and staff are trained appropriately.
“These challenges can easily be overcome by picking a solution that democratizes data and AI, fits seamlessly into existing workflows, and is produced and monitored by a reputable company,” Wang said.
About the Author
You May Also Like