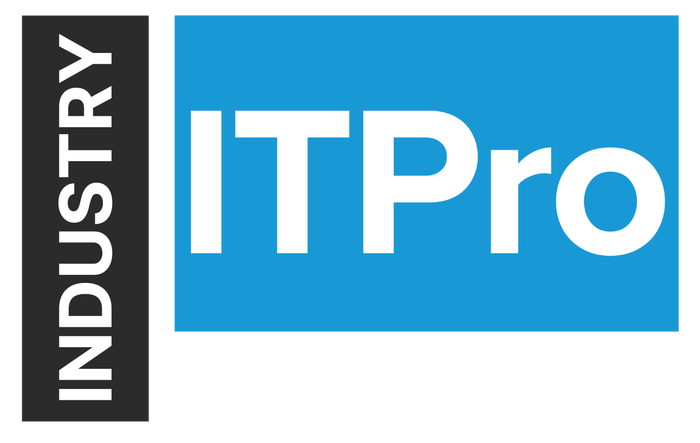
Insight and analysis on the information technology space from industry thought leaders.
AI for Data Management: An Old Idea with New Potential
Organizations should go beyond just using proven methods for leveraging AI to streamline data management by taking advantage of novel techniques that are just now emerging.
August 15, 2023
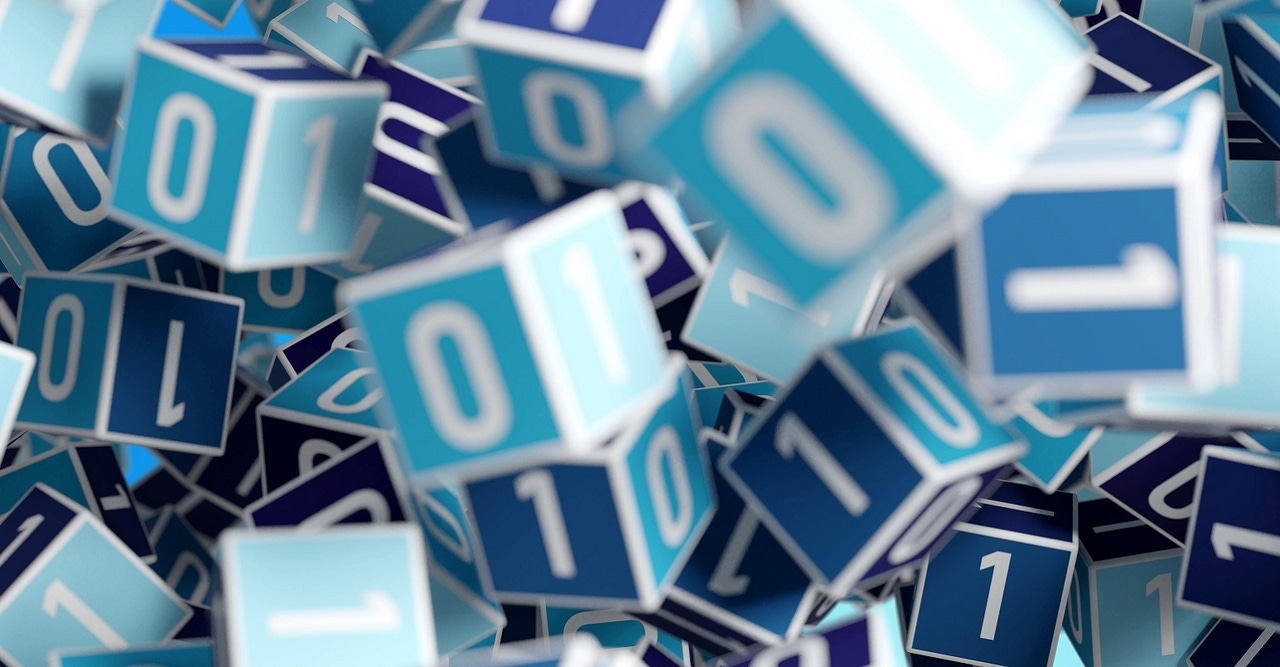
To say that AI is the dominant theme of 2023 is an understatement. Everyone's talking about AI these days and making all manner of predictions about how AI tools will transform tasks of all types — from how we cook to how we manage cybersecurity risks.
In the world of data engineering and management, however, AI is arguably less buzzworthy than it is within other corners of the tech industry. The reason why is that smart data engineering teams have been leveraging AI for years. There remain plenty of good reasons to use AI to accelerate data workflows, but many AI-based data management methods are not actually that new.
That said, there are some novel ways to leverage AI in the data engineering and management space. So, while it would be hyperbolic to say that AI is going to transform the way we work with data, it would also be a mistake to ignore the innovations that AI offers in this realm.
Allow me to explain by discussing the state of AI for data engineering and management and distinguishing what's actually novel from tried-and-true AI techniques.
Why Use AI for Data Management and Engineering
No matter how you choose to leverage AI in the data management space — whether you're using AI for more basic needs or you're taking advantage of next-generation AI technologies — your goal should be to identify ways that AI can accelerate workflows and reduce toil for data engineers.
Much of the work that data engineers perform on a daily basis can be tedious and time-consuming. Converting data from one format to another by hand could take enormous amounts of time and is a boring task, to put it mildly. So is sifting through vast volumes of information to find data quality issues like redundant or empty cells. Even if you leverage tools to help search and sort data automatically, you're still likely to find yourself investing an inordinate amount of time on data quality if you have to write complex queries by hand to detect quality problems.
But if you can substitute AI-based workflows for these tasks, you save yourself a lot of time and labor. By extension, you have more time and mindspace available to devote to tasks that create value — like generating insights based on data, rather than prepping and managing data.
Traditional Use Cases for AI in Data Management
For years, it has been possible to use AI to reduce toil in several major domains within data management.
Data profiling
They include, first, data profiling, which typically happens when organizations prepare to ingest data. Data profiling helps clean up data quality issues, such as leading or trailing spaces or duplicate entries.
Common data products already leverage AI to help with these tasks. For example, if you import a spreadsheet into Google Sheets, it might automatically suggest changes to improve data quality.
Thus, you don't need advanced AI to accelerate data profiling. You just need to know which data products to take advantage of.
Data security
AI can help streamline data security operations, too, especially those related to identifying sensitive information — such as personally identifiable information (PII), personal health information (PHI), and payment card information (PCI) — that exists within data you are working with. Since compliance regulations impose mandates related to how organizations use and secure this data, being able to detect it is critical from a data security and compliance perspective.
Here again, the ability to identify sensitive information using AI has long been a feature of many data products. You won't typically find it in very basic data management software like Google Sheets, but you can obtain it through data loss prevention (DLP) tools, which can automatically search data sets for information linked to compliance and security risks.
Data observation
Data observation is the process of monitoring how data is used to detect anomalies or patterns that could be the sign of a problem. For example, if you notice a sudden drop in the volume of data you're processing or the speed at which data transformations take place, you'll want to investigate further to determine whether there is an issue within your data pipelines.
AI can help with this process by performing anomaly detection, a feature you can find in many data observability tools. AI typically can't tell you why a problem exists, but it will at least accelerate the process of detecting the issue so that you can respond faster.
Next-Generation Use Cases for AI in Data Engineering
Any organization that wants to manage data in an efficient way should already be taking advantage of the types of AI-based data management and data engineering techniques I described above. However, if you want to be truly forward-thinking, you should also be on the lookout for novel approaches to using AI to streamline data workflows.
The greatest opportunity I see on this front is using generative AI to assist with data homogenization. Data homogenization involves taking data from multiple sources and normalizing it to fit a preset data model. It's a common task that businesses need to perform when they have data from multiple systems; for example, a retailer that operates both online and in-store may operate different payment solutions for each context, then merge the payment data so that it can analyze it centrally.
Data homogenization is complex because it typically requires creating a large number of nuanced transformations. You have to identify how each field within each set of data that you're homogenizing must change to fit your data model. There is more complexity and need for customization here than you can address efficiently with standard pattern-matching algorithms.
With generative AI, however, you could potentially automate data homogenization to a large extent. Generative AI tools could evaluate an existing data model, then determine how to change data to match it. They won't be able to homogenize the data with complete success on their own, but they'll significantly reduce the time it takes to set up the necessary transformations by hand.
Conclusion
AI has been at the forefront of innovation in data management and data engineering for years. But as AI technology evolves, data management strategies should evolve with it. Taking advantage of proven methods for leveraging AI to streamline data management is a basic step that every organization should take to reduce data engineer toil, but don't stop there. Look for ways to take advantage of more sophisticated AI solutions to streamline data management further, using novel techniques that are just now emerging.
About the Author
You May Also Like