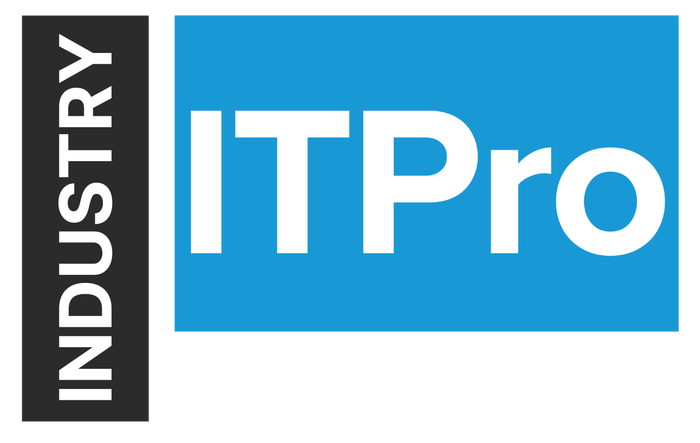
Insight and analysis on the information technology space from industry thought leaders.
Beyond Business Email Compromise: Multi-Channel Phishing Is Here To Stay
Phishing and BEC attacks continue to grow more sophisticated with AI, bypassing traditional defense and expanding across multi-channel platforms like email, text, and social media.
October 25, 2024
.jpg?width=1280&auto=webp&quality=95&format=jpg&disable=upscale)
By Abhilash Garimella, VP of Research at Bolster.ai
For years, phishing attacks have been blamed for stolen credentials that often lead to breaches, data loss, and various types of business email compromise (BEC). Despite the ongoing investments in security solutions meant to keep malicious actors out of networks, BEC remains a primary attack vector—becoming significantly more dangerous due to the proliferation of generative AI. Secure email gateways—once good at finding and stopping known text-based threat signatures—are now vulnerable to attacks since modern adversaries quickly learned that AI can easily eliminate traditional indicators of compromise (IoC), such as bad domains or malicious links and attachments.
Phishing scams also use online surveillance via social media profiles to target victims. Through generative AI, attackers can easily launch multi-pronged scams that employ email, text, social media channels, and phony websites and conduct impersonation attempts via voice or video calls. The attack surface has expanded greatly. Our company recently found that social media-originating phishing attacks have grown 170% since last year, with a 28-times increase in impersonations on LinkedIn.
One reason for why phishing attacks remain a common threat is simply that they work. They continue to be quite effective at tricking human users into paying phony invoices or sharing sensitive information. In fact, according to the Cybersecurity Infrastructure Security Agency, more than 90% of all cyberattacks begin with a phishing scam.
As AI-driven BEC attacks outpace the highly manual, time- and people-intensive threat detection and takedown mechanisms, security and IT teams struggle to defend against them. Traditional cybersecurity measures cannot handle attacks at this momentum, scale, and level of sophistication.
The Shortfalls of Traditional Security
Combating multi-channel phishing and impersonation attacks requires a defense strategy that can easily counter the AI-powered BEC threat. Traditional cybersecurity measures such as spam filters or email encryption are inadequate at stopping a phishing attack presented as a malicious QR code programmed to download malware when a user scans it. Nor do solutions that only analyze email at the user level to recognize or quarantine threats. These reactive approaches only serve to expose the organization to greater risk.
Modern cyber protection, therefore, requires solutions that deliver visibility into malicious activity from outside the organization’s perimeter, scanning across the web, social channels, and mobile devices and through natural language text, images, and even the dark web to identify threats. The context of how phishing and impersonation attacks unfold is critical to detecting and eliminating threats before they even reach an email inbox.
The Abundance of AI-Driven Security
Multi-agent AI systems are revolutionizing phishing detection by automating threat hunting and analysis. These systems combine contextual threat detection and takedown measures with steps to stop the source of the attack, including identifying and takedown domains suspected to become hosts for email servers and phishing attacks. These systems, powered by large language models (LLMs) and computer vision technologies, proactively scan the web to identify phishing and fraud-targeting brands. This allows organizations to identify and disrupt BEC attacks proactively before they inflict damage.
The process begins with gathering data from various threat intelligence sources and open-source integrations. AI-driven models analyze potential phishing URLs, apps, and websites by extracting key features—such as page structure, natural language text, images, and logos—and evaluating them using advanced NLP and image recognition techniques. Multiple LLMs work in tandem to assess the intent behind web content and detect misuse of trademarks, copyrights, and brand assets.
By combining data from multiple models, this approach enables the real-time identification of phishing pages and malicious apps, providing security teams with prioritized threat insights. AI and multi-agent systems streamline the detection process, significantly reducing response times and offering actionable summaries of the phishing threat landscape.
Security Preparedness Remains Relevant
While traditional security approaches like multi-factor authentication fail to stop multi-channel and AI-driven phishing attacks, it and other measures still have a role to play in a comprehensive cybersecurity strategy. In fact, modern sign-in procedures that employ FIDO-approved methods can completely remove insecure passwords from the user authentication process, thus establishing a zero-trust architecture and eliminating a common vector of phishing attacks.
There is great optimism and hope on the horizon, as many security solutions are also becoming equipped with generative AI technology, advancing our collective abilities to detect phony emails or voicemails that closely resemble legitimate messages. When combined with a comprehensive managed detection and incident response plan, organizations can minimize the impact of phishing attacks when or if they occur. This includes proactively simulating roles and responsibilities for organizational stakeholders so that they understand the steps required if a phishing attack leads to a data breach or other security incident. When organizations know their incident response plan protocols and procedures, they are better equipped to contain the attack and recover systems swiftly and effectively.
About the Author
Abhilash Garimella is the VP of Research at Bolster AI where he leads the threat intelligence and SOC team to detect and take down digital threats. Abhilash has a master’s in computer engineering and deep learning, and his work covers cybersecurity, online fraud detection, threat hunting, and applied machine learning. Prior to Bolster, Abhilash conducted threat research at McAfee and was the original scientist at Bolster developing models for automated threat detection and response.
About the Author
You May Also Like