MLPerf Is Changing the AI Hardware Performance Conversation. Here’s how
What’s improving machine learning? Is it competition, or is it something else?
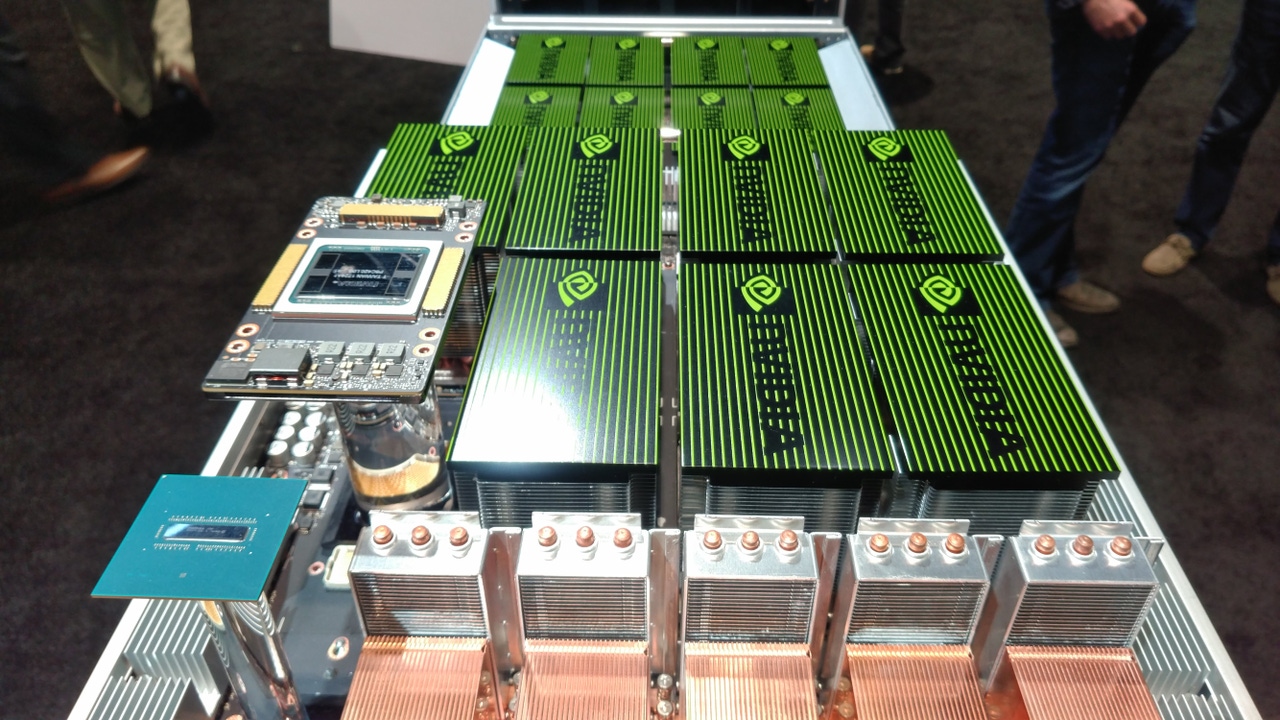
What should “performance” mean in the context of machine learning (ML)? With more supercomputers and cloud computing clusters supporting snap-judgment decisions every minute, artificial intelligence (AI) engineers are finding out it’s just as important to improve the way deep learning algorithms deliver their results as it is to improve the performance of the processors and accelerators that produce them.
Since May 2018, researchers from Baidu, Google, Harvard, Stanford, and UC Berkeley have been developing the MLPerf benchmark. It’s a tool that measures the amount of time consumed in training a machine learning model to the point where its inferences (the ability to make estimates or predictions) reach a 75.9 percent confidence level. ML system builders and architects have been invited to use MLPerf to gauge the accuracy and performance of their systems, and perhaps along the way to do a little bragging about them.
However, in June 2019 MLPerf was expanded, incorporating a new testing category into what’s suddenly become a suite of performance analysis tools. With the addition of an inference benchmark, MLPerf 0.5 can also clock the time algorithms consume post-training in using the data they’ve built up to reach conclusions.
Time-to-Solution
Not every person involved in the operation of an ML system will be a data scientist, says Ramesh Radhakrishnan, technology strategist at Dell EMC and a contributor to the MLPerf development group. Without a complete understanding of what’s going on under the hood, the mechanism of ML can be completely opaque. A simple measurement, such as the total number of reasonably correct predictions, can go a long way toward giving everyone involved in the ML management process a basic competency.
To read the rest of this free article, please fill out the form below:
Read more about:
Data Center KnowledgeAbout the Authors
You May Also Like