Bad Data Impacting Organizations' Resources, Revenue
The data downtime phenomenon is taking time away from data engineers to focus on more innovative and value-generating projects.
October 14, 2022
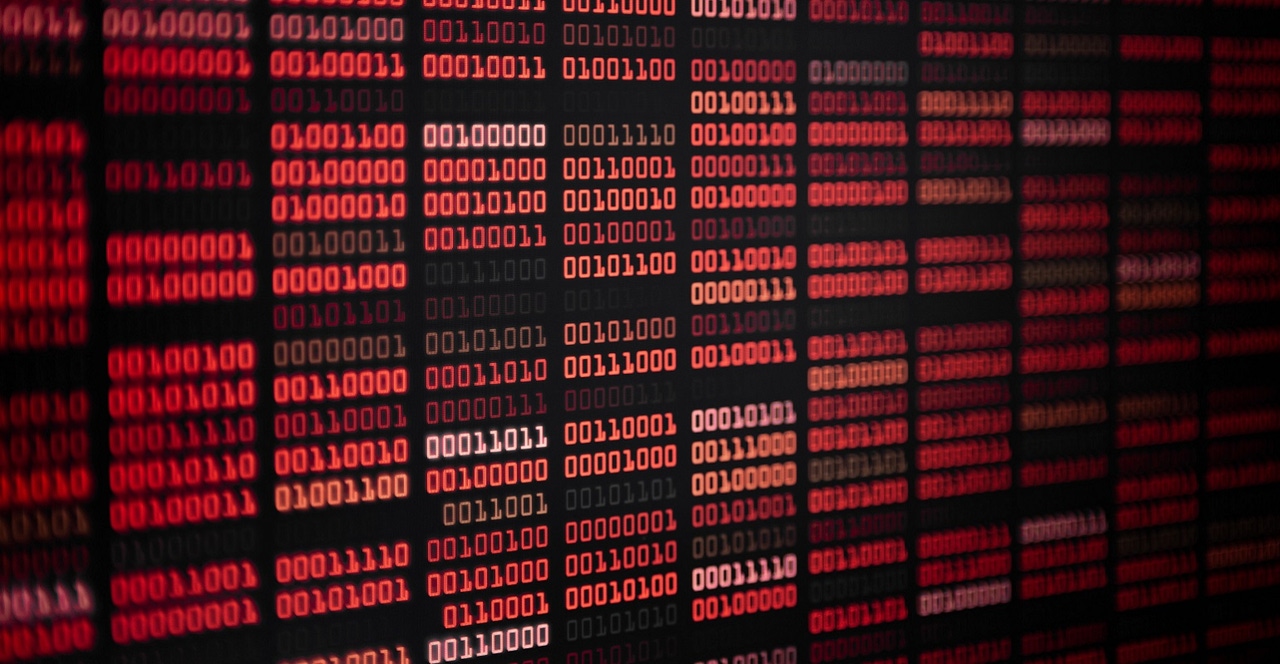
Data professionals are spending 40% of their time evaluating or checking data quality, and poor data quality impacts 26% of their companies' revenue, according to a Wakefield Research and Monte Carlo survey of more than 300 data engineers.
The survey revealed that most respondents take 4 hours or more to detect an incident and, once detected, take an average of 9 hours to resolve it.
The average organization experiences about 61 data-related incidents per month, each of which takes an average of 13 hours to identify and resolve, adding up to an average of about 793 hours per month, per company.
"With the average organization experiencing an average of 61 incidents per month, you can see how that quickly adds up," said Monte Carlo CEO and co-founder Barr Moses.
Time spent firefighting data quality issues means teams can't focus on more innovative projects, such as building pipelines or scaling data systems that can drive revenue and growth for the business, she said.
"Even the most trivial-seeming errors in data quality can snowball into a much bigger issue down the line."
— Barr Moses, CEO, Monte Carlo
The survey found that time spent on data quality isn't the only cost organizations face, with respondents reporting that, on average, bad data impacts 26% of their revenue.
Nearly half said business stakeholders are impacted by issues they don't catch "most of the time" or "all the time."
The 'Data Downtime' Phenomenon
Over the past several years, companies have been ingesting larger and larger volumes of data to power decision-making and drive the development of digital services, according to Moses.
Simultaneously, more employees across their organizations are relying on this data to inform their day-to-day work.
"Any time spent on data quality is time engineers are not spending generating value. That's the best-case scenario. The worst case is companies are relying on incorrect data to make decisions and then end up making costly errors."
— John Bambenek, principal threat hunter, Netenrich
"As a result of this increased data ingestion and adoption, data teams must build more complex and nuanced data pipelines that support multiple use cases across the business," she said. "With more data, more users, and more complexity comes a higher likelihood of data systems breaking; we call this phenomenon data downtime."
Data downtime — periods of time when data is partial, erroneous, missing, or otherwise inaccurate — only multiplies as data systems become increasingly complex, supporting an endless ecosystem of sources and consumers.
"Any time spent on data quality is time engineers are not spending generating value," said John Bambenek, principal threat hunter at Netenrich. "That's the best-case scenario. The worst case is companies are relying on incorrect data to make decisions and then end up making costly errors."
Simply put, the data quality issues were always there; they just weren't generating impact.
When applications run in silos, there is no need to do data normalizing if the solitary application works, Bambenek said.
"As businesses are collecting more and more data into data lakes, they are finding these quality issues as they are trying to make sense and use of it all," he said. "Data that is 'good enough' for one use, may be inaccurate for another."
In an ideal world, Bambenek said, all data will conform to a universal data model so translation and normalization issues will be straightforward to handle.
"As new applications are created or modified, they can simply develop to the data model, so it needs not be created from scratch each time," he explained.
The Consequences of Bad Data Can Be Significant
Moses noted that data is constantly changing, and the consequences of working with unreliable data are far more significant than just an inaccurate dashboard or report.
"Even the most trivial-seeming errors in data quality can snowball into a much bigger issue down the line," she said. "For data engineers and developers, data downtime means wasted time and resources; for data consumers, it erodes confidence in your decision-making."
Particularly in this economic climate, the margin of error for bad data is lower than ever — simply put, companies can't afford to rely on unreliable dashboards or outdated reports to make critical decisions or power customer-facing products, Moses said.
Just like mechanical engineers look for signs that their machines need preventive maintenance to avoid costly breakdowns, data teams need to monitor indicators of data reliability to understand when proactive steps are needed to avoid costly data incidents, she said.
"You don't want to be in a situation where you are repairing the pipeline after its burst and the damage is done," she said. "The good news is that 88% of respondents reported they were already investing or planning to invest in data quality solutions, like data observability, within six months."
Bambenek believes data quality efforts will become more important going forward because these data lakes are ensuring data is being used for purposes beyond its original intention, often to make business decisions.
"The more important decisions that are getting made, the higher the cost of the error," he said. "That said, the direction will continue to be toward making big data-enabled decisions so normalization and quality will continue to grow in significance."
By and large, the two major processes to improve data quality, according to Moses, are testing and data observability.
One of the most common ways to discover data quality issues before they enter your production data pipeline is by testing your data.
"With testing, data engineers can validate their organization's assumptions about the data and write logic to prevent the issue from working its way downstream," she said. "Data testing is a must-have to help catch specific, known problems that surface in your data pipelines and will warn you when new data or code breaks your original assumptions."
About the Author
You May Also Like