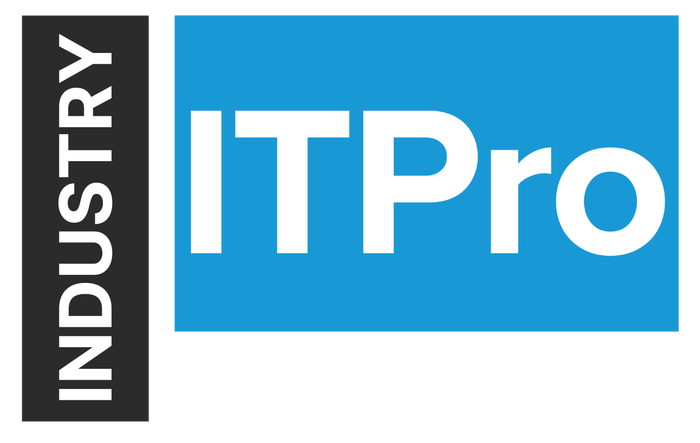
Insight and analysis on the information technology space from industry thought leaders.
Effectively Integrating AI with IGA: The Great Identity Bake-OffEffectively Integrating AI with IGA: The Great Identity Bake-Off
As excitement builds around large language models, AI integrations in Identity Governance and Administration promise to enhance efficiency and decision-making — but only with careful planning.
November 14, 2024
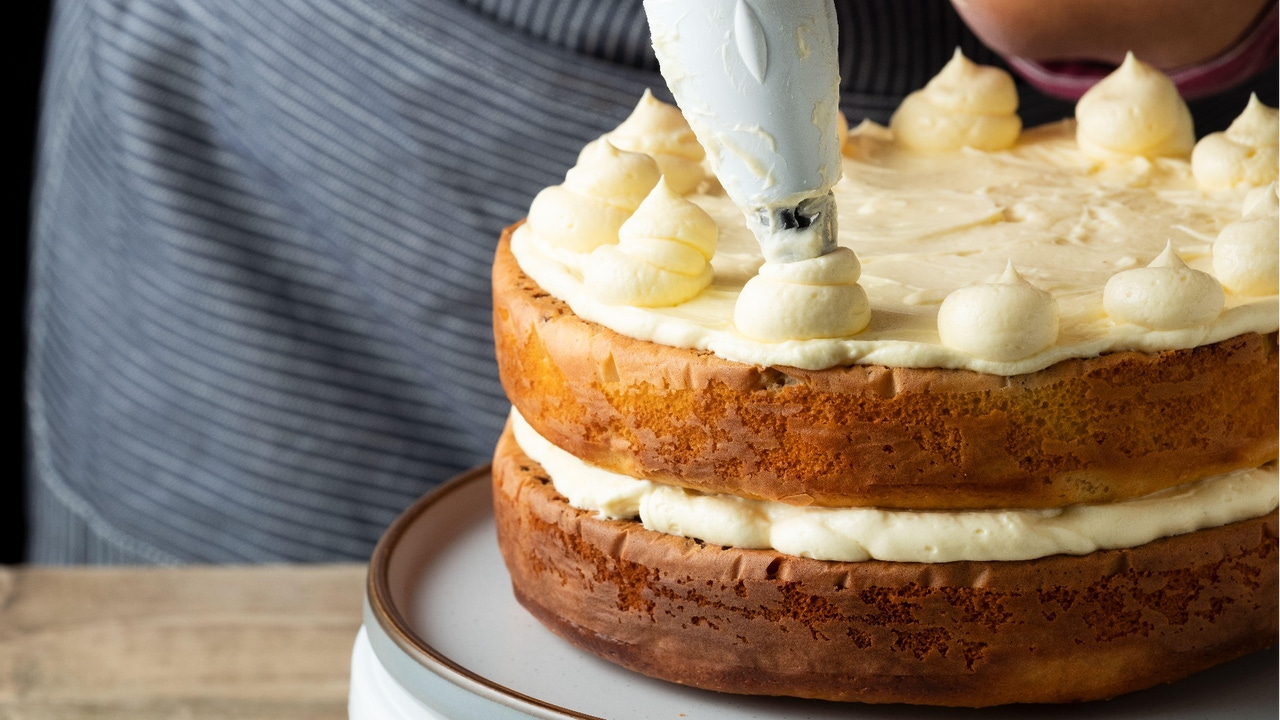
There is still a massive buzz around large language models (LLMs) and generative AI, but it's not the answer to every question for all business cases, including Identity Governance and Administration (IGA). While AI integrations with IGA are nothing new, these rapidly evolving technologies present new and exciting integration opportunities.
That being said, it's essential to be mindful when integrating AI with IGA. A clear strategy and approach to identifying the correct technology for the appropriate use cases is paramount. Failing to do so may result in a solution that looks good but is lacking from a governance or security perspective.
A Layer Cake of Technology
I like to look at this integration as similar to baking a multi-tiered cake. For all the sweet hype around AI right now, it has to be the icing. Licking the bowl might have been the most fun part of baking as a kid, but there's no structure or substance to icing alone. Then you have your well-defined IGA processes and data; this is your sponge cake. It's pretty solid, but things might crumble if you keep stacking it higher and higher, i.e., adding more identities, systems, and policies.
Just spraying icing everywhere — or adding AI without strategy — will leave you with a scene like the infamous cake scene in Disney's Sleeping Beauty, where they're propping the wonky cake up with a broom. However, layering AI between your processes and using it to improve how data is outwardly presented to your end users will leave you with that perfectly layered cake — it's structurally sound and good to look at!
Defining AI and Identifying Opportunities for AI in IGA
It is also important to start by defining artificial intelligence — as all AI is ultimately data science, whether it is machine learning, deep learning, LLMs, or generative AI. Knowing when to use which discipline is crucial. For example, machine learning algorithms are great for decision intelligence and data segmentation/classification, whereas generative AI is more useful for content generation and conversational user interfaces.
If we look at integrating AI specifically with IGA, we can already see access approval and review decision support, role mining, and risk score automation. These use cases are pretty well-defined, but there is always room for improvement, and there is far more potential yet, including expanding chatbot and AI assistant functionality for end users and much more.
But AI isn't a guaranteed instant value creator. There's a lot of work that needs to be done to make it work for IGA.
Existing AI/IGA Integrations and Where to Go Next
Human-computer co-decisions are the most established "AI" discipline as we know it. Computers can process large quantities of data at an incredible pace and then present it in an easily digestible format. This is a great example of the coexistence between technology, human decision-making, and accountability.
Dive deeper into the other layers of impact AI can have on IGA, and there is enormous potential. Staying with utilization, beyond intelligent decision support and role mining, AI could drive further intelligent risk mitigation and entitlement policy co-generation and be utilized as chatbot assistants with the front-end UI and back-end documentation. If done properly, this would add value and improve end-user satisfaction when interacting with IGA.
Switching focus to the implementation layer, AI integrations could benefit end users, vendors, and partners in the IGA space through data clean-up automation, configuration guidance, and connectivity/API schema automation. Finally, at the development layer, AI integrations could mean faster feature delivery and product improvements through things like product documentation assistance, product development improvements via agents like co-pilot, and AI-supported product pen-testing.
All the above needs to be done in a structured way, with a clear strategy and with end-user enablement in mind. For example, end users who have never interacted with an AI assistant before may need to be enabled on prompt engineering, and developers must be clear about what data can and cannot be shared with particular AI models.
Ethical, Legal and Privacy Considerations
Today, organizations struggle with universal data quality, and data clean-up initiatives are expensive and time-consuming. This data may also be limited in size, and AI models need larger and higher-quality data sets. There is an opportunity to utilize generic models, and given the speed of AI development and the fact that they are striving for better and more precise models with lower data requirements, these challenges may become more manageable.
From a confidentiality and integrity perspective, AI needs a broad data reference to provide accurate and detailed answers. Creating granular access models that compare each data point in a GPT model to the IGA access of the user interacting with the model is highly complex. Without a well-thought-out generative AI strategy, delivering on this may end up sacrificing the confidentiality and integrity of your organization's data.
Finally, from a legal perspective, AI has no legal entity, which is a problem for regulatory compliance, dictating accountability and trackability to a legal individual. Machines can be objectively more precise under the right conditions but cannot be held liable for incorrect actions. AI can also undermine the critical thinking of experienced human beings — i.e., would you have approved this access if AI hadn't recommended you do so?
Ultimately, you need to identify the right balance between AI & AI — artificial intelligence and accountable individuals.
Toward Meaningful IGA AI integrations
Despite the gloomy nature of some of the considerations above, there is still plenty to be excited about in terms of the potential and possibilities that emerging AI technologies present within the IGA space and beyond. New legislation, such as the EU AI Act, is a great step in the right direction to ensure organizations act ethically when dealing with huge amounts of customer data while not stifling innovation.
Regarding your organization, the importance of ensuring you have an AI strategy that is regularly reviewed can't be underestimated. Align this with your IGA and broader cybersecurity requirements and user stories, and it should become clear when applying the right type of AI will create the right type of value and prevent falling into the trap of AI being the answer before you even know the question. Be critical, be challenging, be pragmatic, but be excited, too. There's a lot of very cool stuff on the horizon in the world of IGA and AI.
About the author:
Craig Ramsay has a wealth of experience within identity, primarily focused around IAM and IGA. He has worked at financial institutions helping them set up and run their identity functions before moving to the vendor side into professional services and is now a senior solutions architect at Omada. He has strong experience in user lifecycle management, role management, violation management, and identity strategy with knowledge of complementary identity technologies such as PAM, DAG, CIEM, and others. Craig is based out of Edinburgh, Scotland.
About the Author
You May Also Like