Build Your GenAI Strategy on a Rock-Solid Foundation (Model)Build Your GenAI Strategy on a Rock-Solid Foundation (Model)
The market for AI foundation models is still in its infancy. While enterprises still rely mostly on pretrained large language models for AI needs, the market is evolving and changing quickly. Tech leaders must understand how to navigate it.
April 18, 2024
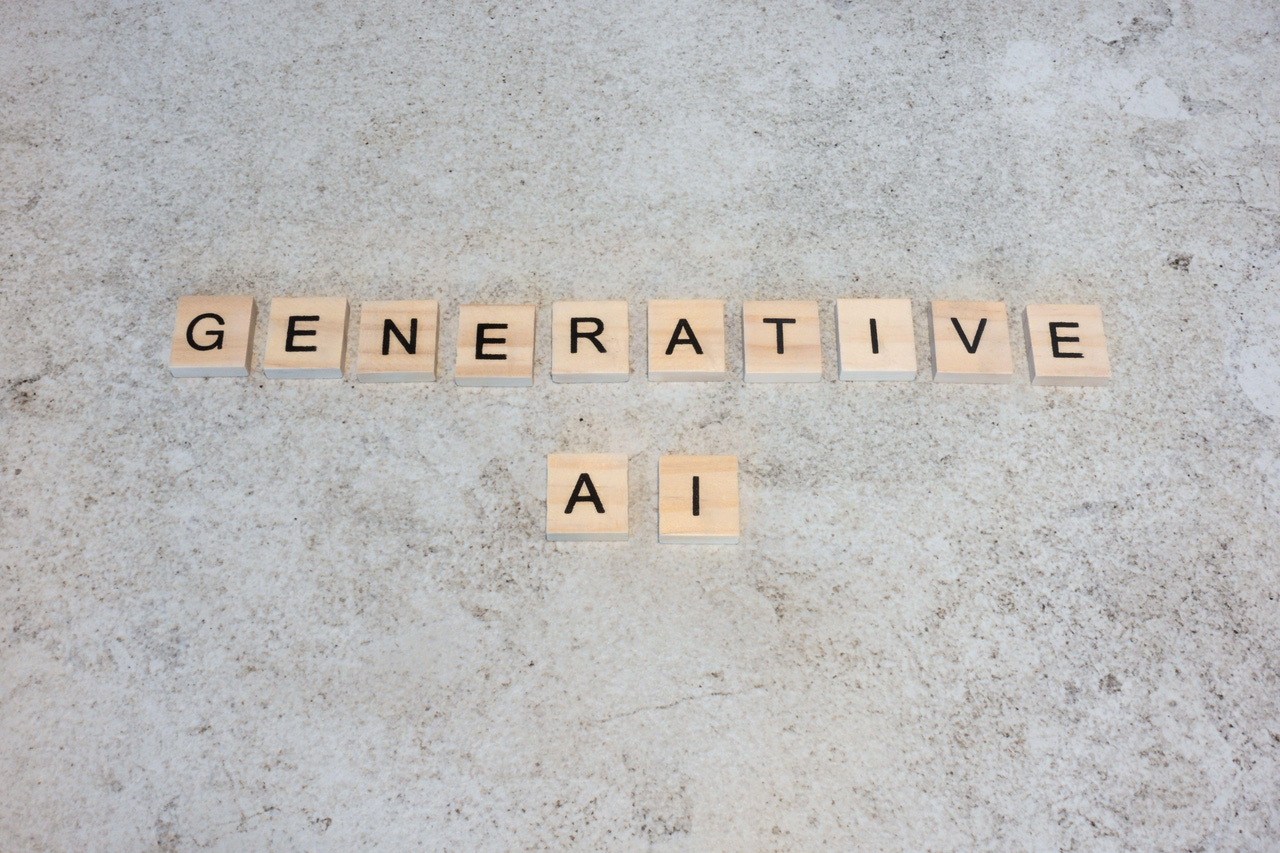
OpenAI’s ChatGPT set the record for fast-growing consumer application, and there are now scores of other models similar to GPT-3.5 available (both proprietary and open-source), but don’t be fooled: The market for foundation models powering generative AI (genAI) and predictive AI is still in its infancy. For any language-related genAI task — be it writing code, supporting customer service, or creating ad copy — enterprises are relying on what Forrester is calling AI foundation models for language (AI-FMLs). These are the pretrained (typically) large language models (LLMs) that can ingest and generate text, though multimodal models, which can also ingest and produce audio, images, or video, have crested the horizon. This market is evolving and changing quickly, and tech leaders must understand how to navigate it.
Next-generation AI applications will be built on foundation models, but …
Foundation models are the bedrock of genAI-powered applications, and there will be many models (large and small) targeted at different parts of data pipelines and workflows. Forrester clients can learn more about key concepts related to foundation models in our report, The Technology Leader’s Primer For AI Foundation Models, but all readers searching for AI-FMLs with which to build their applications need to know that:
AI-FMLs will create efficiencies at scale across domains and work functions. Many leaders are familiar with AI-FMLs’ capabilities for Q&A and summarization, but these models also excel in other domains like data preparation. For example, general-purpose AI-FMLs enable businesses to extract and understand information such as sentiments, topics, and named entities from ingested content. Traditional machine learning models require massive sets of training data, are resource-intensive to build, and don’t scale reliably across multiple domains. AI-FMLs are allowing developers to bypass building their own ML models for these tasks (thus bypassing laborious data preparation work) and to create applications that can more easily adapt to other tasks and domains.
But no single foundation model will support all the needs of an enterprise. There is not currently a do-everything model that can meet the needs of every team within an organization. Tech executives should plan to utilize multiple foundation models, depending upon the data or application workflow. Some tasks may require a high-end model for specific types of summarizations or analysis, but many tasks can be accomplished with models that are smaller or lower-performance on paper.
Enterprises must select foundation models carefully.
For the foreseeable future, most enterprises will source their foundation models from third parties and not pretrain their own. Forrester clients can start building their AI-FML purchasing strategy using our new report, The AI Foundation Models For Language Landscape, Q2 2024, which includes information on how AI-FML vendors differ in terms of offerings, size, and market focus. When choosing an AI-FML, enterprises must:
Weigh cost, power, and domain training. Sometimes cutting-edge models will confer a competitive advantage, but other times, the cost of running them will outweigh the benefits, and older or smaller open-source models will suffice. But don’t simply look at the base model capabilities: An AI-FML may work for many generalized language tasks, but it may also need significant work to align to your use case. Some industries use domain-specific language in very precise ways (think manufacturing or medicine), and general-purpose models may not cut it for applications in those industries.
Vet models based on their ecosystem capabilities. AI-FMLs deployed for business are parts of larger application ecosystems that support accuracy and transparency in model behavior. Capabilities for engineering/testing/validating prompts, developing retrieval-augmented generation (RAG) architectures, and plugging into external applications’ APIs are essential parts of AI-FMLs in genAI applications. Before committing to a model, find out whether it will work with the needs of your technology ecosystem and whether it will connect all your tools effectively.
We will release a Forrester Wave™ evaluation covering AI-FML this summer, looking at the leading vendors based on scoring criteria such as data preparation, training tools, and model governance. Forrester clients can discuss our evaluative research — or foundation models generally — in more depth by scheduling a guidance session with an AI analyst.
This article originally appeared on Forrester's Featured Blogs.
Read more about:
Generative AIAbout the Author
You May Also Like